Full Paper View Go Back
E. Asiedu1
Section:Research Paper, Product Type: Conference-Paper
Vol.6 ,
Issue.6 , pp.70-80, Dec-2019
Online published on Dec 31, 2019
Copyright © E. Asiedu . This is an open access article distributed under the Creative Commons Attribution License, which permits unrestricted use, distribution, and reproduction in any medium, provided the original work is properly cited.
View this paper at Google Scholar | DPI Digital Library
How to Cite this Paper
- IEEE Citation
- MLA Citation
- APA Citation
- BibTex Citation
- RIS Citation
IEEE Style Citation: E. Asiedu, “In-Silico Methods for Investigating the Effect of Single Nucleotide Polymorphisms on the Structure and Function of Proteins,” International Journal of Scientific Research in Biological Sciences, Vol.6, Issue.6, pp.70-80, 2019.
MLA Style Citation: E. Asiedu "In-Silico Methods for Investigating the Effect of Single Nucleotide Polymorphisms on the Structure and Function of Proteins." International Journal of Scientific Research in Biological Sciences 6.6 (2019): 70-80.
APA Style Citation: E. Asiedu, (2019). In-Silico Methods for Investigating the Effect of Single Nucleotide Polymorphisms on the Structure and Function of Proteins. International Journal of Scientific Research in Biological Sciences, 6(6), 70-80.
BibTex Style Citation:
@article{Asiedu_2019,
author = {E. Asiedu},
title = {In-Silico Methods for Investigating the Effect of Single Nucleotide Polymorphisms on the Structure and Function of Proteins},
journal = {International Journal of Scientific Research in Biological Sciences},
issue_date = {12 2019},
volume = {6},
Issue = {6},
month = {12},
year = {2019},
issn = {2347-2693},
pages = {70-80},
url = {https://www.isroset.org/journal/IJSRBS/full_paper_view.php?paper_id=1612},
publisher = {IJCSE, Indore, INDIA},
}
RIS Style Citation:
TY - JOUR
UR - https://www.isroset.org/journal/IJSRBS/full_paper_view.php?paper_id=1612
TI - In-Silico Methods for Investigating the Effect of Single Nucleotide Polymorphisms on the Structure and Function of Proteins
T2 - International Journal of Scientific Research in Biological Sciences
AU - E. Asiedu
PY - 2019
DA - 2019/12/31
PB - IJCSE, Indore, INDIA
SP - 70-80
IS - 6
VL - 6
SN - 2347-2693
ER -
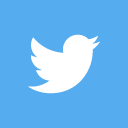
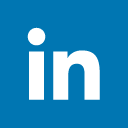
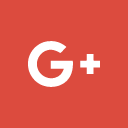
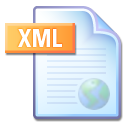
Abstract :
Single nucleotide polymorphisms (SNPs) are associated with diseases and drug response variabilities in humans. Elucidating the damaging and disease-associated SNPs using wet-laboratory approaches can be challenging and resource-demanding due to the large number of SNPs in the human genome. Due to the growth in the field of computational biology and bioinformatics, algorithms have been developed to help screen and filter out the most deleterious SNPs that are worth considering for wet-laboratory studies. This article reviews the existing in-silico based methods used to predict and characterize the effects of SNPs on protein structure and function. This cutting-edge approach will facilitate the search for novel therapeutics, help understand the etiology of diseases and fast-track the personalized medicine agenda.
Key-Words / Index Term :
single nucleotide polymorphism, docking, molecular dynamics, in-silico studies, protein dynamics, missense, prediction algorithm, mutation
References :
[1] J. Våge, F. Lingaas, “Single nucleotide polymorphisms (SNPs) in coding regions of canine dopamine- and serotonin-related genes”, BMC genetics, Vol.9, Issue.10, 2008. doi:10.1186/1471-2156-9-10.
[2] R. Madelaine, J. H. Notwell, G. Skariah, C. Halluin, C. Chen, G. Bejerano, P. Mourrain, “A screen for deeply conserved non-coding GWAS SNPs uncovers a MIR-9-2 functional mutation associated to retinal vasculature defects in human”, Nucleic acids research, Vol.46, Issue.7, pp.3517–3531, 2018. doi:10.1093/nar/gky166.
[3] J. Chen, W. Tian, “Explaining the disease phenotype of intergenic SNP throuh predicted long-range regulation”, Nucleic acids research, Vol.44, Issue.18, pp.8641–8654, 2016. doi:10.1093/nar/gkw519.
[4] T. Zhou, E. A. Ko, W. Gu, I. Lim, H. Bang, J. H. Ko, “Non-silent story on synonymous sites in voltage-gated ion channel genes”, PloS one, Vol.7, Issue.10, pp.e48541, 2012.
doi: 10.1371/journal.pone.0048541.
[5] A. A. Komar, “GENETICS: SNPs, Silent but Not Invisible. Science”, Vol.315, Issue.5811, pp.466–467, 2007. doi:10.1126/science.1138239.
[6] S. Behjati, P. S. Tarpey, “What is next generation sequencing? Archives of disease in childhood” Education and practice edition, Vol.98, Issue.6, pp.236–238, 2013. doi:10.1136/archdischild-2013-304340.
[7] M. J. Islam, A. M. Khan, M. R. Parves, M. N. Hossain, M. A. Halim, “Prediction of Deleterious Non-synonymous SNPs of Human STK11 Gene by Combining Algorithms”, Molecular Docking, and Molecular Dynamics Simulation. Scientific reports, Vol.9, Issue.1, pp.16426, 2019. doi:10.1038/s41598-019-52308-0.
[8] S. Bhavaniramya, R. Vanajothi, S. Vishnupriya, M. S. Al-Aboody, R. Vijayakumar, D. Baskaran, “Computational characterization of deleterious SNPs in Toll-like receptor gene that potentially cause mastitis in dairy cattle”, Biocatalysis and Agricultural Biotechnology, Vol.19, pp.101151, 2019.
doi: 10.1016/j.bcab.2019.101151.
[9] K. Muthusamy, S. Nagamani, “Vitamin D receptor (VDR) non-synonymous single nucleotide polymorphisms (nsSNPs) affect the calcitriol drug response - A theoretical insight”, Journal of Molecular Graphics and Modelling, Vol.81, pp.14–24, 2018. doi: 10.1016/j.jmgm.2018.02.004.
[10] D. Thirumal Kumar, C. George Priya Doss, P. Sneha, I. A. Tayubi, R. Siva, C. Chakraborty, R. Magesh, “Influence of V54M mutation in giant muscle protein titin: a computational screening and molecular dynamics approach”, Journal of Biomolecular Structure and Dynamics, Vol.35, Issue.5, pp.917–928, 2016. doi:10.1080/07391102.2016.1166456.
[11] I. Khan, I. A. Ansari, P. Singh, J. F. P. Dass, F. Khan, “Identification and characterization of functional single nucleotide polymorphisms (SNPs) in Axin 1 gene: a molecular dynamics approach”, Cell Biochemistry and Biophysics, Vol.76, Issue.1-2, pp.173–185, 2017. doi:10.1007/s12013-017-0818-1.
[12] C. G. Doss, C. Chakraborty, L. Chen, H. Zhu, “Integrating in silico prediction methods, molecular docking, and molecular dynamics simulation to predict the impact of ALK missense mutations in structural perspective”, BioMed research international, Vol.2014, pp.895831, 2014. doi:10.1155/2014/895831.
[13] I. A. Adzhubei, S. Schmidt, L. Peshkin, V. E. Ramensky, A. Gerasimova, P. Bork, A. S. Kondrashov, S. R. Sunyaev, “A method and server for predicting damaging missense mutations’, Nature Methods, Vol.7, Issue.4, pp.248-249, 2010.
[14] P. C. Ng, S. Henikoff, “Accounting for human polymorphisms predicted to affect protein function” Genome Research, Vol.12, pp.436–446, 2002.
[15] H. Ashkenazy, S. Abadi, E. Martz, O. Chay, I. Mayrose, T. Pupko, N. Ben-Tal, “ConSurf 2016: an improved methodology to estimate and visualize evolutionary conservation in macromolecules”, Nucleic acids research, Vol.44, Issue.1, pp.344–350, 2016. doi:10.1093/nar/gkw408.
[16] S. T. Sherry, M. H. Ward, M. Kholodov, J. Baker, L. Phan, E. M. Smigielski, K. Sirotkin, “dbSNP: the NCBI database of genetic variation”, Nucleic acids research, Vol.29, Issue.1, pp.308–311, 2002. doi:10.1093/nar/29.1.308.
[17] E. M. Smigielski, K. Sirotkin, M. Ward, S. T. Sherry, “dbSNP: a database of single nucleotide polymorphisms”, Nucleic Acids Research, Vol.28, Issue.1, pp.352–355, 2000.
https://doi.org/10.1093/nar/28.1.352
[18] P. D. Stenson, M. Mort, E. V. Ball, K. Evans, M. Hayden, S. Heywood, D. N. Cooper, “The Human Gene Mutation Database: towards a comprehensive repository of inherited mutation data for medical research, genetic diagnosis and next-generation sequencing studies”, Human genetics, Vol.136, Issue.6, pp.665–677, 2017. doi: 10.1007/s00439-017-1779-6.
[19] S. Ngak-Leng, K. Prateek; H. Jing, S. Henikoff, S. Georg, P. C. Ng, “SIFT web server: predicting effects of amino acid substitutions on proteins”, Nucleic Acids Research, Vol.40, pp.452–457. 2012, doi:10.1093/nar/gks539.
[20] T. L. J. Mah, E. S. H. Low, E. Lee, “In silico SNP analysis and bioinformatics tools: a review of the state of the art to aid drug discovery”, Drug Discovery Today, Vol.16, 2011.
[21] P. C. Ng, S. Henikoff, “Predicting deleterious amino acid substitutions”, Genome Research, Vol.11, pp.863–874, 2001.
[22] Y. Choi, G. E. Sims, S. Murphy, J. R. Miller, A. P. Chan, “Predicting the Functional Effect of Amino Acid Substitutions and Indels”, PLoS ONE, Vol.7, Issue.10, pp.e46688, 2012.
[23] Y. Choi, A. P. Chan, “PROVEAN web server: a tool to predict the functional effect of amino acid substitutions and indels”, Bioinformatics, Vol.31, Issue.16, pp.2745-2747, 2015.
[24] Y. Choi, “A Fast Computation of Pairwise Sequence Alignment Scores between a Protein and a Set of Single-Locus Variants of another Protein”, In Proceedings of the ACM Conference on Bioinformatics, Computational Biology and Biomedicine (BCB `12) ACM, New York, NY, USA, pp.414-417, 2012.
[25] Y. Bromberg, B. Rost, “SNAP: predict effect of non-synonymous polymorphisms on function”, Nucleic Acids Research, Vol.35, Issue.11, pp.3823-3835, 2007.
[26] M. Hecht, Y. Bromberg, B. Rost, “Better prediction of functional effects for sequence variant”, BMC Genomics; Vol.16(Suppl 8): S1, 2015.
[27] V. Ramensky, P. Bork, S. Sunyaev, “Human non-synonymous SNPs: server and survey”, Nucleic Acids Research, Vol.30, Issue.17, pp.3894-3900, 2002.
[28] S. R. Sunyaev, F. Eisenhaber, I. V. Rodchenkov, B. Eisenhaber, V. G. Tumanyan, E. N. Kuznetsov, “PSIC: profile extraction from sequence alignments with position-specific counts of independent observations”, Protein Engineering, Vol.12, Issue.5, pp.387-394, 1999.
[29] H. Tang, D. P. Thomas, “PANTHER-PSEP: predicting disease-causing genetic variants using position-specific evolutionary preservation”, Bioinformatics, Vol.32, Issue.14, 2016. doi: 10.1093/bioinformatics/btw222.
[30] E. A. Stone, A. Sidow, “Physicochemical constraint violation by missense substitutions mediates impairment of protein function and disease severity”, Genome Research, Vol.15, pp.978–986, 2005. doi:10.1101/gr.3804205.
[31] L. Bao, M. Zhou, Y. Cui, “nsSNPAnalyzer: identifying disease-associated nonsynonymous single nucleotide polymorphisms”, Nucleic Acids Research, Vol.33, pp.480–482, 2005
doi:10.1093/nar/gki372.
[32] P. D. Thomas, A. Kejariwal, “Coding single-nucleotide polymorphisms associated with complex vs. Mendelian disease: Evolutionary evidence for differences in molecular effects”, Proc Natl Acad Sci, Vol.101, pp.15398–15403, 2004. doi:10.1073/pnas.0404380101.
[33] E. Capriotti, R. Calabrese, R. Casadio, “Predicting the insurgence of human genetic diseases associated to single point protein mutations with support vector machines and evolutionary information”, Bioinformatics, Vol.22, pp.729–2734, 2006. doi:10.1093/bioinformatics/btl423.
[34] V. Pejaver, S. D. Mooney, P. Radivojac, “Missense variant pathogenicity predictors generalize well across a range of function-specific prediction challenges”, bioRxiv, 134981, 2017, doi: https://doi.org/10.1101/134981.
[35] C. Ferrer-Costa, J. L. Gelpi, L. Zamakola, I. Parraga, X. de la Cruz, M. Orozco, “PMUT: a web-based tool for the annotation of pathological mutations on proteins”, Bioinformatics (Oxford, England), Vol.21, pp.3176–3178, 2005.
[36] V. López-Ferrando, A. Gazzo, X. de la Cruz, M. Orozco, J. L. Gelpí, “PMut: a web-based tool for the annotation of pathological variants on proteins, 2017 update”, Nucleic Acids Research, 2017. doi: 10.1093/nar/gkx313.
[37] H. Ashkenazy, E. Erez, E. Martz, T. Pupko, N. Ben-Tal, “ConSurf 2010: calculating evolutionary conservation in sequence and structure of proteins and nucleic acids”, Nucleic Acids Research, Vol.38, pp.529-533, 2010. doi: 10.1093/nar/gkq399; PMID: 20478830.
[38] G. Celniker, G. Nimrod, H. Ashkenazy, F. Glaser, E. Martz, I. T. Mayrose Pupko, N. Ben-Tal, “ConSurf: Using Evolutionary Data to Raise Testable Hypotheses about Protein Function”, Israel Journal of Chemistry, Vol.53: pp.199-206, 2013. doi: 10.1002/ijch.201200096.
[39] D. B. Kitchen, H. Decornez, J. R. Furr, J. Bajorath, “Docking and scoring in virtual screening for drug discovery: methods and applications”, Nature Reviews. Drug Discovery, Vol.3, Issue.11, pp.935–949, 2004. doi:10.1038/nrd1549.
[40] N. S. Pagadala, K. Syed, J. Tuszynski, “Software for molecular docking: a review”, Biophysical reviews, Vol.9, Issue.2, pp.91–102, 2017. doi:10.1007/s12551-016-0247-1.
[41] B. Q. Wei, L. H. Weaver, A. M. Ferrari, B. W. Matthews, B. K. Shoichet, “Testing a flexible-receptor docking algorithm in a model binding site”, Journal of Molecular Biology, Vol.337, Issue.5, pp.1161–82, 2004. doi: 10.1016/j.jmb.2004.02.015.
[42] A. Hernandez-Santoyo, A. Yair, V. Altuzar, H. Vivanco-Cid, C. Mendoza-Barrer, “Protein-Protein and Protein-Ligand Docking”, Protein Engineering - Technology and Application, 2013. doi: 10.5772/56376.
[43] E.; Yuriev, P. A. Ramsland, “Latest developments in molecular docking: 2010-2011 in review”, Journal of Molecular Recognition, Vol.26, Issue.5, pp.215–239, 2013, doi:10.1002/jmr.2266.
[44] V. Khanna, S. Ranganathan, N. Petrovsky, “Rational Structure-Based Drug Design”, Reference Module in Life Sciences, 2018. doi:10.1016/b978-0-12-809633-8.20275-6.
[45] X. Y. Meng, H. X. Zhang, M. Mezei, M. Cui, “Molecular docking: a powerful approach for structure-based drug discovery”, Current computer-aided drug design, Vol.7, Issue.2, pp.146–157, 2011. doi:10.2174/157340911795677602.
[46] A. A. T. Naqvi, T. Mohammad, G. M. Hasan, M. I. Hassan, “Advancements in Docking and Molecular Dynamics Simulations Towards Ligand-receptor Interactions and Structure-function Relationships”, Current Topics in Medicinal Chemistry, Vol.18, Issue.20, pp.1755–1768, 2018.
[47] P. Kangueane, C. Nilofer, “Protein-Protein Docking: Methods and Tools”, Protein-Protein and Domain-Domain Interactions, pp.161–168, 2018. doi: 10.1007/978-981-10-7347-2_14.
[48] C. George Priya Doss, N. Nagasundaram, C. Chakraborty, L. Chen, H. Zhu, “Extrapolating the effect of deleterious nsSNPs in the binding adaptability of flavopiridol with CDK7 protein: a molecular dynamics approach”, Human genomics, Vol.7, Issue.1, pp.10, 2013. doi:10.1186/1479-7364-7-10.
[49] C. George Priya Doss, C. Chakraborty, V. Narayan, D. hirumal Kumar, “Computational Approaches and Resources in Single Amino Acid Substitutions Analysis Toward Clinical Research”, Advances in Protein Chemistry and Structural Biology, Vol.94, pp.365–423, 2014. doi:10.1016/b978-0-12-800168-4.00010-x.
[50] K.Lundstrom, “Structural genomics for membrane proteins”, Cellular and Molecular Life Sciences, Vol.63, Issue.22, pp.2597–2607, 2006. doi:10.1007/s00018-006-6252-y.
[51] M. Kovermann, P. Rogne, M. Wolf-Watz, “Protein dynamics and function from solution state NMR spectroscopy”, Quarterly Reviews of Biophysics, Vol.49, 2016. doi:10.1017/s0033583516000019.
[52] R. Nussinov, J. A. Papin, I. Vakser, “Computing the Dynamic Supramolecular Structural Proteome”, PLOS Computational Biology, Vol.13, Issue.1, pp.e1005290, 2017. doi: 10.1371/journal.pcbi.1005290.
[53] I. Yu, T. Mori, T. Ando, R. Harada, J. Jung, Y. Sugita, M. Feig, “Biomolecular interactions modulate macromolecular structure and dynamics in atomistic model of a bacterial cytoplasm”, eLife, Vol.5, pp.e19274, 2016. doi:10.7554/eLife.19274.
[54] A. Hospital, J. R. Goñi, M. Orozco, J. L. Gelpí, “Molecular dynamics simulations: advances and applications”, Advances and applications in bioinformatics and chemistry, Vol.8, pp.37–47, 2015. doi:10.2147/AABC.S70333.
[55] M. Karplus, J. A. McCammon, “Molecular dynamics simulations of biomolecules”, Nature Structural Biology, Vol.9, Issue.9, pp.646–652, 2002. doi:10.1038/nsb0902-646.
[56] S. A. Hollingsworth, R. O. Dror, “Molecular Dynamics Simulation for All”, Neuron, 2018, Vol.99, Issue.6, pp.1129–1143, 2018. doi: 10.1016/j.neuron.2018.08.011.
[57] J. R. Perilla, B. C. Goh, C. K. Cassidy, B. Liu, R. C. Bernardi, T. Rudack, K. Schulten, “Molecular dynamics simulations of large macromolecular complexes”, Current Opinion in Structural Biology, Vol.31, pp.64–74, 2015. doi: 10.1016/j.sbi.2015.03.007.
[58] M. J. Abraham, T. Murtola, R. Schulz, S. Páll, J. C. Smith, B. Hess, E. Lindahl, “GROMACS: High performance molecular simulations through multi-level parallelism from laptops to supercomputers”, SoftwareX, Vol.1-2, pp.19–25, 2015. doi: 10.1016/j.softx.2015.06.001.
[59] B. R. Brooks, C. L. Brooks 3rd, A. D. Mackerell Jr., et al. “CHARMM: the biomolecular simulation program”, Journal of Computational Chemistry, Vol.30, Issue.10, pp.1545–1614, 2009. doi:10.1002/jcc.21287.
[60] D. A. Case, T. E. Cheatham, T. Darden, H. Gohlke, R. Luo, K. M. Merz Jr, R. J. Woods, “The Amber biomolecular simulation programs”, Journal of computational chemistry, Vol.26, Issue.16, pp.1668–1688, 2005. doi:10.1002/jcc.20290.
[61] Y. Wang, C. B. Harrison, K. Schulten, J. A. McCammon, “Implementation of Accelerated Molecular Dynamics in NAMD”, Computational science & discovery, Vol.4, Issue.1, pp.015002, 2011. doi:10.1088/1749-4699/4/1/015002.
[62] E. Krieger, G. Vriend, “YASARA View - molecular graphics for all devices - from smartphones to workstations”, Bioinformatics (Oxford, England), Vol.30, Issue.20, pp.2981–2982, 2014. doi:10.1093/bioinformatics/btu426.
[63] I. Lappalainen, J. Lopez, L. Skipper, T.Hefferon, J. D. Spalding, D. M. Church, et al., “dbVar and DGVa: public archives for genomic structural variation. Nucleic Acids Research”, Vol.41, Issue.D1, pp.D936–D941, 2012. doi:10.1093/nar/gks1213
[64] A. Hamosh, A. F. Scott, J. S. Amberger, C. A. Bocchini, V. A. McKusick, “Online Mendelian Inheritance in Man (OMIM), a knowledgebase of human genes and genetic disorders”, Nucleic acids research, Vol.33, pp.514–517, 2005. doi:10.1093/nar/gki033.
[65] R. A. Gibbs, E. Boerwinkle, H. Doddapaneni, Y. Han, V. Korchina, J. G. Reid, “A global reference for human genetic variation”, Nature, Vol.526, Issue.7571, pp.68–74, 2015. doi:10.1038/nature15393.
[66] J. M. Barbarino, M. Whirl-Carrillo, R. B. Altman, T. E. Klein, “PharmGKB: A worldwide resource for pharmacogenomic information”. Wiley interdisciplinary reviews. Systems biology and medicine, Vol.10, Issue.4, pp.e1417, 2018. doi:10.1002/wsbm.1417.
[67] E. M. McDonagh, M. Whirl-Carrillo, Y. Garten, R. B. Altman, T. E. Klein, “From pharmacogenomic knowledge acquisition to clinical applications: the PharmGKB as a clinical pharmacogenomic biomarker resource”, Biomarkers in Medicine, Vol.5, Issue.6, pp.795-806, 2011.
[68] C. F. Thorn, T. E. Klein, R. B. Altman, “PharmGKB: the pharmacogenomics knowledge base”, Methods in Molecular Biology, 2013, doi:1015:311–320.
[69] K. Higasa, N. Miyake, J. Yoshimura, K. Okamura, T. Niihori, H. Saitsu, F. Matsuda, “Human genetic variation database, a reference database of genetic variations in the Japanese population”, Journal of human genetics, Vol.61, Issue.6, pp.547–553, 2016. doi:10.1038/jhg.2016.12.
[70] M. J. Landrum, J. M. Lee, M. Benson, G. R. Brown, C. Chao, S. Chitipiralla, D. R. Maglott, “ClinVar: improving access to variant interpretations and supporting evidence”, Nucleic Acids Research, 2017, Vol.46, Issue.D1, pp.1062–1067, 2017. doi:10.1093/nar/gkx1153.
[71] I. F. A. C. Fokkema, P. E. M. Taschner, G. C. P. Schaafsma, J. Celli, J. F. J. Laros, J. T. den Dunnen, “LOVD v.2.0: the next generation in gene variant databases”, Human Mutation, Vol.32, Issue.5, pp.557–563, 2011. doi:10.1002/humu.21438.
[72] K. J. Karczewski, B. Weisburd, B. Thomas, M. Solomonson, D. M. Ruderfer, D. Kavanagh, D. G. MacArthur, “The ExAC browser: displaying reference data information from over 60, 000 exomes”, Nucleic acids research, Vol.45, Issue.1, pp840–845, 2017. doi:10.1093/nar/gkw971.
[73] J. G. Tate, S. Bamford, H. C. Jubb, Z. Sondka, D. M. Beare, N. Bindal, S. A. Forbes, “COSMIC: The Catalogue of Somatic Mutations in Cancer”, Nucleic Acids Research, 2018. doi:10.1093/nar/gky1015.
[74] M. C. Brandon, “MITOMAP: a human mitochondrial genome database--2004 update”, Nucleic Acids Research, Vol.33, pp.D611–D613, 2004. doi:10.1093/nar/gki079.
[75] J. R. MacDonald, R. Ziman, R. K. Yuen, L. Feuk, S. W. Scherer, “The Database of Genomic Variants: a curated collection of structural variation in the human genome”, Nucleic acids research, Vol.42, pp.D986–D992, 2014. doi:10.1093/nar/gkt958.
[76] T. C. Ofoegbu, A. David, L. A. Kelley, S. Mezulis, S. A. Islam, S. F. Mersmann, M. Sternberg, “PhyreRisk: A Dynamic Web Application to Bridge Genomics, Proteomics and 3D Structural Data to Guide Interpretation of Human Genetic Variants”, Journal of molecular biology, Vol.431, Issue.13, pp.2460–2466, 2019. doi: 10.1016/j.jmb.2019.04.043.
[77] H. Venselaar, T.A. te Beek, R.K. Kuipers, et al., “Protein structure analysis of mutations causing inheritable diseases: An e-Science approach with life scientist friendly interfaces”, BMC Bioinformatics, Vol.11, Issue.548, 2010. doi: 10.1186/1471-2105-11-548.
[78] J. Thusberg, A. Olatubosun, M. Vihinen, “Performance of mutation pathogenicity prediction methods on missense variants”, Human Mutation, Vol.32, pp.358-368., 2011.
[79] E. Capriotti, R Calabrese, P Fariselli, P. L. Martelli, R. B. Altman, R. Casadio, “WS-SNPs&GO: a web server for predicting the deleterious effect of human protein variants using functional annotation”, BMC Genomics, Suppl 3: S6, 2013.
[80] T. Jian, W. Ningfeng, G. Jun G. Xuexia Z. Juhua, F. Yunliu, “Predicting the phenotypic effects of non-synonymous single nucleotide polymorphisms based on support vector machines”, BMC Bioinformatics, Vol.8, Issue.1, pp.450, 2007.
[81] G. De Baets, J. Van Durme, J. Reumers, S. Maurer-Stroh, P. Vanhee, J. Dopazo, F. Rousseau, “SNPeffect 4.0: on-line prediction of molecular and structural effects of protein-coding variants”, Nucleic acids research, Vol.40, pp.D935–D939, 2012. doi:10.1093/nar/gkr996.
[82] M. Nawar, J. M. Steven, G. Jones, G. Jörg, “Improved measures for evolutionary conservation that exploit taxonomy distances”, Nature Communications, Vol.10, pp.1556, 2019. doi: 10.1038/s41467-019-09583-2.
[83] C. M. Yates, I. Filippis, L. A. Kelley, M. J. E. Sternberg, “SuSPect: Enhanced Prediction of Single Amino Acid Variant (SAV) Phenotype Using Network Features”, Journal of Molecular Biology, Vol.426, Issue.14, pp.2692–2701, 2014. doi: 10.1016/j.jmb.2014.04.026
[84] S. Forli, R. Huey, M. E. Pique, M. F. Sanner, D. S. Goodsell, A. J. Olson, “Computational protein-ligand docking and virtual drug screening with the AutoDock suite”, Nature protocols, Vol.11, Issue.5, pp.905–919, 2016. doi:10.1038/nprot.2016.051.
[85] A. Grosdidier, V. Zoete, O. Michielin, “SwissDock, a protein-small molecule docking web service based on EADock DSS”, Nucleic acids research, Vol.39, pp.W270–W277, 2011. doi:10.1093/nar/gkr366.
[86] W. J. Allen, T. E. Balius, S. Mukherjee, S. R. Brozell, D. T. Lang, P. T. Moustakas, R. C. Rizzo, “DOCK 6: Impact of new features and current docking performance”, Journal of Computational Chemistry, Vol.36, Issue.15, pp.1132–1156, 2015. doi:10.1002/jcc.23905.
[87] J. M. Yang, C. C. Chen, “GEMDOCK: A generic evolutionary method for molecular docking”, Proteins: Structure, Function, and Bioinformatics, Vol.55, Issue.2, pp.288–304, 2004. doi:10.1002/prot.20035.
[88] O. Trott, A. J. Olson, “AutoDock Vina: improving the speed and accuracy of docking with a new scoring function, efficient optimization, and multithreading”, Journal of computational chemistry, Vol.31, Issue.2, pp.455–461, 2010. doi:10.1002/jcc.21334.
[89] M. McGann, “FRED Pose Prediction and Virtual Screening Accuracy”, Journal of Chemical Information and Modeling, Vol.51, Issue.3, pp.578–596, 2011. doi:10.1021/ci100436p.
[90] S. Vajda, C. Yueh, D. Beglov, T. Bohnuud, S. E. Mottarella, B. Xia, D. R. Hall, D. Kozakov, “New additions to the ClusPro server motivated by CAPRI”, Proteins: Structure, Function, and Bioinformatics, Vol.85, Issue.3, pp.435-444, 2017.
[91] D. Kozakov, R. Brenke, S. R. Comeau, S. Vajda, “PIPER: An FFT-based protein docking program with pairwise potentials”, Proteins: Structure, Function, and Bioinformatics, Vol.65, Issue.2, pp.392–406, 2006. doi:10.1002/prot.21117.
[92] D. Schneidman-Duhovny, Y. Inbar, R. Nussinov, H. J. Wolfson, “PatchDock and SymmDock: servers for rigid and symmetric docking”, Nucleic acids research, Vol.33, pp.W363–W367, 2005. doi:10.1093/nar/gki481.
[93] A. Tovchigrechko, I. A. Vakser, “GRAMM-X public web server for protein-protein docking”, Nucleic acids research, Vol.34, pp.W310–W314, 2006. doi:10.1093/nar/gkl206.
[94] S. Chaudhury, M. Berrondo, B. D. Weitzner, P. Muthu, H. Bergman, J. J. Gray, “Benchmarking and analysis of protein docking performance in Rosetta v3.2”, PloS one, Vol.6, Issue.8, pp.e22477, 2011. doi: 10.1371/journal.pone.0022477.
[95] T. M. K. Cheng, T. L. Blundell, J. Fernandez-Recio, “pyDock: Electrostatics and desolvation for effective scoring of rigid-body protein-protein docking”, Proteins: Structure, Function, and Bioinformatics, Vol.68, Issue.2, pp.503–515, 2007. doi:10.1002/prot.21419.
[96] B. Jiménez-García, C. Pons, J. Fernández-Recio, “pyDockWEB: a web server for rigid-body protein–protein docking using electrostatics and desolvation scoring”, Bioinformatics, Vol.29, Issue.13, pp.1698–1699, 2013. doi:10.1093/bioinformatics/btt262
[97] G. C. P. Van Zundert, J. P. G. L. M. Rodrigues, M. Trellet, C. Schmitz, P. L. Kastritis, E. Karaca, A. M. J. J. Bonvin, “The HADDOCK2.2 Web Server: User-Friendly Integrative Modeling of Biomolecular Complexes”, Journal of Molecular Biology, Vol.428, Issue.4, pp.720–725, 2016. doi: 10.1016/j.jmb.2015.09.014.
[98] Y. Yan, D. Zhang, P. Zhou, B. Li, S. Y. Huang, “HDOCK: a web server for protein-protein and protein-DNA/RNA docking based on a hybrid strategy”, Nucleic acids research, Vol.45, Issue.W1, pp.W365–W373, 2017. doi:10.1093/nar/gkx407.
[99] D. Schneidman-Duhovny, Y. Inbar, R. Nussinov, H. J. Wolfson, “PatchDock and SymmDock: servers for rigid and symmetric docking”, Nucleic acids research, Vol.33, pp.W363–W367, 2015. doi:10.1093/nar/gki481
You do not have rights to view the full text article.
Please contact administration for subscription to Journal or individual article.
Mail us at support@isroset.org or view contact page for more details.