Full Paper View Go Back
An Intelligent Approach for Prediction of Candidate Heart Disease Patients
A. Abdo1 , Ramadan Bakir2
Section:Research Paper, Product Type: Journal-Paper
Vol.8 ,
Issue.1 , pp.20-36, Feb-2020
Online published on Feb 28, 2020
Copyright © A. Abdo, Ramadan Bakir . This is an open access article distributed under the Creative Commons Attribution License, which permits unrestricted use, distribution, and reproduction in any medium, provided the original work is properly cited.
View this paper at Google Scholar | DPI Digital Library
How to Cite this Paper
- IEEE Citation
- MLA Citation
- APA Citation
- BibTex Citation
- RIS Citation
IEEE Style Citation: A. Abdo, Ramadan Bakir, “An Intelligent Approach for Prediction of Candidate Heart Disease Patients,” International Journal of Scientific Research in Computer Science and Engineering, Vol.8, Issue.1, pp.20-36, 2020.
MLA Style Citation: A. Abdo, Ramadan Bakir "An Intelligent Approach for Prediction of Candidate Heart Disease Patients." International Journal of Scientific Research in Computer Science and Engineering 8.1 (2020): 20-36.
APA Style Citation: A. Abdo, Ramadan Bakir, (2020). An Intelligent Approach for Prediction of Candidate Heart Disease Patients. International Journal of Scientific Research in Computer Science and Engineering, 8(1), 20-36.
BibTex Style Citation:
@article{Abdo_2020,
author = {A. Abdo, Ramadan Bakir},
title = {An Intelligent Approach for Prediction of Candidate Heart Disease Patients},
journal = {International Journal of Scientific Research in Computer Science and Engineering},
issue_date = {2 2020},
volume = {8},
Issue = {1},
month = {2},
year = {2020},
issn = {2347-2693},
pages = {20-36},
url = {https://www.isroset.org/journal/IJSRCSE/full_paper_view.php?paper_id=1688},
publisher = {IJCSE, Indore, INDIA},
}
RIS Style Citation:
TY - JOUR
UR - https://www.isroset.org/journal/IJSRCSE/full_paper_view.php?paper_id=1688
TI - An Intelligent Approach for Prediction of Candidate Heart Disease Patients
T2 - International Journal of Scientific Research in Computer Science and Engineering
AU - A. Abdo, Ramadan Bakir
PY - 2020
DA - 2020/02/28
PB - IJCSE, Indore, INDIA
SP - 20-36
IS - 1
VL - 8
SN - 2347-2693
ER -
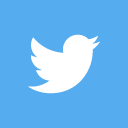
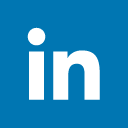
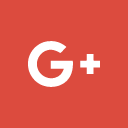
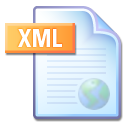
Abstract :
Coronary is serious disease and one of the most common heart diseases that needs great care in doctoral analysis and diagnosis. Doctoral experience is considered one of the most common factors that negatively affect diagnosis of Coronary Heart Disease (CHD); sometimes due to lack of experience. Under this context, many attempts have been done to build a CHD prediction system using different data mining (DM) methods to assist in diagnosis, and also to minimize risk in case of mistaken diagnosis. We have noted two weaknesses in these attempts, First: many of the previous researches have used the symptoms of the CHD disease as factors leading to the disease, which already exists; Second: there was highly imbalanced class distribution in the dataset, which in turn led to imbalanced class distribution in the results. We have worked on the first problem in a previous research to predict heart disease using risk factors using AHDBP (An Adaptive Heart Disease Behavior-Based Prediction model). In this research we have developed ACHDBP as an extension to AHDBP. A balancing technique called Synthetic Minority Over-sampling Technique (SMOTE) is used in the current research to handle this problem. Different classification algorithms will be deployed to get the most accurate results. 18 attributes were used to build the prediction system. Different SMOTE ratios between 100 and 500 were applied to the data. Results were recorded and compared with results obtained without using SMOTE algorithm. For all SMOTE sampling ratios the most classifier that achieved the best classifier was NB classifier. The average accuracy values for DT, SVM, NN, and NB was 73.85%, 73.76%, 73.04%, and 72.58% respectively. The obtained class distributions were balanced but the accuracy of the system was lowered, and we believe that happens because SMOTE algorithm is more effective for large databases.
Key-Words / Index Term :
Coronary, Classification, SMOTE Oversampling, symptoms, Risk Factors
References :
[1] Mozaffarian. D, Benjamin. E.J, et al. “Heart disease and stroke statistics”. American Heart Association. Circulation Vol.4 : pp. 38-360, . 2016 Jan 26.
[2] Fryar. C.D, Chen. T. Li X. “Prevalence of Uncontrolled Risk Factors for Cardiovascular Disease”. National Center for Health Statistics. Vol.103, pp.1-8, 2012 Aug.
[3] J C Wyatt,JLYLiu. “Basic Concepts in medical informatics”. Journal of Epidemiology & Community Health. Vol.11, pp.12-808, December 2002.
[4] Yang. Q, Wu. X. “10 Challenging Problems in Data Mining Research”. International Journal of Information Technology & Decision Making, Vol. 5, No.4, pp. 597–604, 2006.
[5] Emam. O. E, Abdo. A, Mona. M. M. “An Adaptive Heart Disease Behavior-Based Prediction System”. International Journal of Advanced Computer Science and Applications (IJACSA), Vol. 10, No. 1, 2019.
[6] Beers. M. “Aging and the Cardiovascular System. The Merck Manual of Geriatrics”. Merck & Co. Inc. Vol 6, Issue 1, 2016, pp. 47-51.
[7] Guyton. A, Hall. J. Textbook of medical physiology. Philadelphia, W. B. Saunders, pp. 116:1767, 2000.
[8] Bunch T.J. Weiss J.P. Crandall B.G. May H.T. Bair T.L. Osborn J.S. Day J.D. “Atrial fibrillation is independently associated with senile, vascular, and Alzheimer`s dementia. Heart Rhythm”. Vol 7, pp. 433-7, 2010.
[9] Kling JM. “Depression and the risk of myocardial infarction and coronary death: a meta-analysis of prospective cohort studies”. Medicine (Baltimore). February 2016.
[10] Rosengren A, Wilhelmsen L, Wedel H. “Coronary heart disease, cancer and mortality in male middle-aged light smokers”. Journal of Internal Medicine. Vol.4, pp.357–62, 1992.
[11] Ahlemeyer-Stubbe A, Coleman S. “A practical guide to data mining for business and industry”. John Wiley & Sons, 2014.
[12] Al-Zoubi. AM. Faris. H. Hassonah. MA et al. “evolving support vector machines using whale optimization algorithm for spam profiles detection on online social networks in different lingual contexts”. Knowledge Based Syst. Elsevier. 2018.
[13] Faris H, Hassonah MA, Al-Zoubi AM, Mirjalili S, Aljarah I. “A multi-verse optimizer approach for feature selection and optimizing SVM parameters based on a robust system architecture”. Comput Appl., Springer, Vol 3, No. 4, pp.1–15, 2017.
[14] Harley. W. “An Interactive Node-Link Visualization of Convolutional Neural Networks”. ISVC, pp. 867-877, 2015.
[15] Dougherty. J.R, Kohavi, et al. “Supervised and unsupervised discretization of continuous features”. In: Proceedings of the 12th international conference on machine learning. San Francisco: Morgan Kaufmann: pp. 194–202, 1995.
[16] Ozgür A. “Supervised and unsupervised machine learning techniques for text document categorization”, Citeseer. pp. 258–267, 2006.
[17] Al-Aidaroos K. Bakar A.A. Othman Z. “Medical data classification with naive Bayes approach”. Inf Technol J. pp. 11:1166, 2012.
[18] Ben-Hur, A., Ong. C.S., Sonnenburg. S, Schölkopf. B. Rätsch. G. “Support vector machines and kernels for computational biology”. PLoS Comput Biol 4, no.10 (2008): e1000173.
[19] Kang. P. cho. S. “Ensemble of under sampled SVMs for data imbalance problems”. Artif Intell. pp.107–118, 2006.
[20] Mease, D., Wyner. A.J. Buja. “A. Boosted classification trees and class probability/quantile estimation”. J Machine Learn Res Vol. 8, pp.409–439, 2007.
[21] Hido. S, Kashima. H, Takahashi. Y. “Roughly balanced bagging for imbalanced data”. Stat Anal Data Min Vol. 2, pp. 412–26, 2009.
[22] Zweig.M.H, Campbell G. “Receiver-operating characteristic (ROC) plots: a fundamental evaluation tool in clinical medicine”. Clin Chem. pp.39:561, 1993.
[23] Assari. R., Azimi. P. Taghva. M.R. “Heart Disease Diagnosis Using Data Mining Techniques”. Int J Econ Manag Sci. Vol. 6, 2017.
[24] Aravinthan. K. Vanitha. M. “A Novel Method for Prediction Of Heart Disease Using Naïve Bayes”. International Journal of Advanced Research Trends in Engineering and Technology (IJARTET) Vol. 3, Special Issue 20, April 2016.
[25] Mokashi. R. Madhuri N. Pooja T. Walke. “Heart Disease Prediction Using ANN and Improved K-Means”. International Journal of Innovative Research in Electrical, Electronics, Instrumentation and Control Engineering Vol. 4, Issue 4, April 2016.
[26] Boshra. H. Mirsaeid. S. “Prediction and Diagnosis of Heart Disease by Data Mining Techniques”. Journal of Multidisciplinary Engineering Science and Technology (JMEST), Vol. 2 Issue 2, February 2015.
[27] Hlaudi. D.M. Mosima. A. M. “Prediction of Heart Disease using Classification Algorithms”. Proceedings of the World Congress on Engineering and Computer Science. Vol 2, pp.22-24 October - 2014.
[28] Pandey. K. Prabhat. P, Jaiswal. K.L. Ashok K. S. “A Novel Frequent Feature Prediction Model For Heart Disease Diagnosis”. International Journal of Software & Hardware Research in Engineering. Vol. 1, Issue. 1, September 2013.
[29] Daniels. S.R. Arnett. D.K. Eckel. R.H. Gidding. S.S. Hayman. L.L. “Overweight in children and adolescents: Pathophysiology, consequences, prevention and treatment”. Circulation, 2012.
[30] Chaitral. S. Sulabha S.” Improved Study of Heart Disease Prediction System using Data Mining Classification Techniques. International Journal of Computer Applications”. Vol. 47–No.10, June 2012.
[31] Bhatla. N. Kiran. J. “A Novel Approach for Heart Disease Diagnosis using Data Mining and Fuzzy Logic”. international Journal of Computer Applications Vol. 54, pp. 0975 –8887, September 2012.
[32] Vijayarani. S. Sudha. S. “A Study of Heart Disease Prediction in Data Mining”. IRACST - International Journal of Computer Science and Information Technology & Security (IJCSITS), Vol. 2, No.5, October 2012.
[33] Chen. A.H, Huang. S.Y, Hong. P.S, Cheng. C.H, Lin E.J. HDPS: “Heart Disease Prediction System. Computing in Cardiology”. pp. 557-560, 2011.
[34] Anbarasi. M, Anupriya. E. “Enhanced Prediction of Heart Disease with Feature Subset Selection using Genetic Algorithm”. International Journal of Engineering Science and Technology Vol. 2, 2010.
[35] Shinde. S. B. Amrit. P. “Diagnosis of Heart Disease Using Data Mining Technique”. International Journal of Science and Research (IJSR). Vol. 2, No. 10, pp. 29-35, 2013.
[36] World Health Organization. “The risk factors leading to a heart attack”.
[37] Cleveland database: http://archive.ics.uci.edu/ml/datasets/Heart+Disease.
[38] Statlog database: http://archive.ics.uci.edu/ml/machine-learning-databases/statlog/heart/.
[39] Longadge. R, Dongre. S. “Class imbalance problem in data mining review”. pp.1305.1707, 2013.
[40] Chawla. NV, Japkowicz. N, Kotcz. A. “Editorial: special issue on learning from imbalanced data sets”. ACM SIGKDD Explor Newslett . Vol. 6, pp.1–6, 2004.
[41] He. H, Garcia. E.A. “Learning from imbalanced data”. IEEE Trans Know Data Eng . VOL. 21, NO. 9, SEPTEMBER 2009 .
[42] Thanathamathee. P, Lursinsap. C. Handling imbalanced data sets with synthetic boundary data generation using bootstrap re-sampling and AdaBoost techniques. Pattern Recognit Lett. Vol. 21(9), pp. 1263–1284, 2009.
[43] Longadge. R, Dongre. S. Class imbalance problem in data mining review. 2013arXiv:1305.1707. Chawla NV, Japkowicz N, Kotcz A. Editorial: special issue on learning from imbalanced data sets. ACM SIGKDD Explor Newslett Vol.6, pp.1–6, 2004.
[44] Rahman. M.M, Davis. D. Addressing the class imbalance problem in medical datasets. Int J Mach Learn Comput. pp.3:224, 2003.
[45] Chawla. N.V, Bowyer. K.W, Hall. L.O, Kegelmeyer. W.P. Smote: synthetic minority oversampling technique. J Artif Intell Res. Vol. 16, pp.321–57, 2004.
[46] Witten. I.H. Frank. E. Hall. M.A, Pal. C.J. Data mining: practical machine learning tools and techniques. Morgan Kaufmann; 2016.
[47] Rahman. M.M, Davis. D. Addressing the class imbalance problem in medical datasets. Int J Mach Learn Comput. Vol. 3, 2013.
[48] Bogner. C, KĂĽhnel. A, Huwe. B. Predicting with limited data – increasing the accuracy in vis-nir diffuse reflectance spectroscopy by smote. 2014 6th Workshop on Hyperspectral Image and Signal Processing: Evolution in Remote Sensing (WHISPERS) pp.1–4, 2014.
You do not have rights to view the full text article.
Please contact administration for subscription to Journal or individual article.
Mail us at support@isroset.org or view contact page for more details.