Full Paper View Go Back
Comparative Analysis of Deep Learning Models for Detection of COVID-19 from chest X-Ray Images
Oluwadare Adepeju Adebisi1 , John Adedapo Ojo2 , Olatunde Michael Oni3
Section:Research Paper, Product Type: Journal-Paper
Vol.8 ,
Issue.5 , pp.28-35, Oct-2020
Online published on Oct 31, 2020
Copyright © Oluwadare Adepeju Adebisi, John Adedapo Ojo, Olatunde Michael Oni . This is an open access article distributed under the Creative Commons Attribution License, which permits unrestricted use, distribution, and reproduction in any medium, provided the original work is properly cited.
View this paper at Google Scholar | DPI Digital Library
How to Cite this Paper
- IEEE Citation
- MLA Citation
- APA Citation
- BibTex Citation
- RIS Citation
IEEE Style Citation: Oluwadare Adepeju Adebisi, John Adedapo Ojo, Olatunde Michael Oni, “Comparative Analysis of Deep Learning Models for Detection of COVID-19 from chest X-Ray Images,” International Journal of Scientific Research in Computer Science and Engineering, Vol.8, Issue.5, pp.28-35, 2020.
MLA Style Citation: Oluwadare Adepeju Adebisi, John Adedapo Ojo, Olatunde Michael Oni "Comparative Analysis of Deep Learning Models for Detection of COVID-19 from chest X-Ray Images." International Journal of Scientific Research in Computer Science and Engineering 8.5 (2020): 28-35.
APA Style Citation: Oluwadare Adepeju Adebisi, John Adedapo Ojo, Olatunde Michael Oni, (2020). Comparative Analysis of Deep Learning Models for Detection of COVID-19 from chest X-Ray Images. International Journal of Scientific Research in Computer Science and Engineering, 8(5), 28-35.
BibTex Style Citation:
@article{Adebisi_2020,
author = {Oluwadare Adepeju Adebisi, John Adedapo Ojo, Olatunde Michael Oni},
title = {Comparative Analysis of Deep Learning Models for Detection of COVID-19 from chest X-Ray Images},
journal = {International Journal of Scientific Research in Computer Science and Engineering},
issue_date = {10 2020},
volume = {8},
Issue = {5},
month = {10},
year = {2020},
issn = {2347-2693},
pages = {28-35},
url = {https://www.isroset.org/journal/IJSRCSE/full_paper_view.php?paper_id=2100},
publisher = {IJCSE, Indore, INDIA},
}
RIS Style Citation:
TY - JOUR
UR - https://www.isroset.org/journal/IJSRCSE/full_paper_view.php?paper_id=2100
TI - Comparative Analysis of Deep Learning Models for Detection of COVID-19 from chest X-Ray Images
T2 - International Journal of Scientific Research in Computer Science and Engineering
AU - Oluwadare Adepeju Adebisi, John Adedapo Ojo, Olatunde Michael Oni
PY - 2020
DA - 2020/10/31
PB - IJCSE, Indore, INDIA
SP - 28-35
IS - 5
VL - 8
SN - 2347-2693
ER -
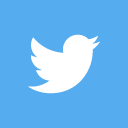
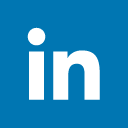
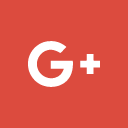
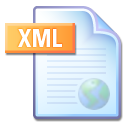
Abstract :
The coronavirus disease is a viral infectious disease resulting from severe acute respiratory syndrome coronavirus. The new coronavirus, which began in China, in December 2019, has rapidly become pandemic and resulted to over 500000 deaths worldwide. Prompt detection of COVID-19 is necessary to prevent the transmission of COVID-19. In this research, we developed Deep Learning (DL) models for detection of COVID-19 from chest X-ray Images and evaluated the performance of the models by using accuracy, sensitivity and specificity. 401 COVID-19 chest X-ray images were obtained from open access database developed by Dr Cohen while 397 normal and 390 pneumonia chest X-ray images were obtained from Kaggle repository. Modified Alexnet, Googlenet and SqueezeNet were used to classify the chest X-ray images. Transfer learning with Alexnet achieved an overall best performance of 100% accuracy, 100% sensitivity and 100% specificity on binary test dataset and 98.31% accuracy, 98.55% sensitivity and 99.37% specificity on three classes test dataset. The work will provide early detection of COVID-19 and thereby enhance medical decisions, treatments and management procedures.
Key-Words / Index Term :
Coronavirus; X-ray Images; Deep Learning; Convolutional Neural Network; Transfer Learning
References :
[1] M. Nicola, Z. Alsafi, C. Sohrabi, A. Kerwan, A. Al Jabir, C. Iosifidis, M. Agha, R. Agha, "The socio economic implications of the coronavirus pandemic (COVID-19) A review”. International journal of surgery (London, England) vol. 78, pp.185–193, 2020
[2] A. Nath, “Impact of COVID-19 Pandemic Lockdown on Mental Well-Being amongst Individuals in Society,” International Journal of Scientific Research in Network Security and Communication, Vol.8, Issue.4, pp.6-9, 2020
[3] A. Hajjar, A. Ziad, A. Memish, K. McIntosh, “Middle East Respiratory Syndrome Coronavirus (MERS- CoV): A Perpetual Challenge,” Ann Saudi Med, Vol. 33 issue 5, pp.427–436, 2013
[4] C. Cheng, Susanna K. P. Lau, Y. Woo, Y Yuen, ”Syndrome Coronavirus as an Agent of Emerging and Reemerging Infection’ ’American Society for Microbiology, Vol. 20 issue 4, pp .660-894–436, 2007
[5] S .Lauer, K. Grantz, F. Jones, Q Zheng, R. Meredith, S. Azman. The Incubation Period of Coronavirus Disease 2019 (COVID- 19) From Publicly Reported Confirmed Cases: Estimation and Application. Annals of internal medicine, Vol 172 issue 9, pp. 577–582, 2020
[6] H. Guo, Y.Zhou , X. Liu , J. Tan, "The impact of the covid-19 epidemic on the utilization of emergency dental Services” , Journal of Dental Sciences, 2020
[7] S. Chavez,B. Long, A. Koyfman, Y.Liang, "Coronavirus disease (covid-19): A primer for emergency physicians, The American journal of emergency medicine” vol. 6757 issue 20, pp 3018-3019, 2020
[8] D.Hemdan, M. Shouman, M. Karar, "COVIDX-Net: A framework of deep learning classifiers to diagnose COVID-19 in x-ray images," arXiv preprint arXiv:2003.11055, pp 1-14, 2020
[9] M. Avci, N. Kozaci, “ Comparison of X-Ray Imaging and
Computed Tomography Scan in the Evaluation of Knee
Trauma’’. Medicina , 2019, Vol 55, issue10 , 2019
[10] Y. Bengio, Y. LeCun,and G. Hinton, Deep Learning". Nature.: vol 7553 issue 521 , 436-444, 2015.
[11] T. Ozturk, M. Talo, E. Yildirimc, U. Baloglu, O. Yildirim, U. Acharya, " Automated Detection of COVID-19 Cases Using Deep Neural Networks with X-Ray Images” Computers in Biology and Medicine, 121: 10379, 2020
[12] J.P. Cohen, “ COVID-19 image data collection, 2020
[13] A. Goldbloom , “ Chest image kaggle data collection’, 2010
[14] Z. Alom , M. Rahman, S. Nasrin , M. Tarek., K. Asari, "COVID_MTNet: COVID-19 Detection with Multi-Task Deep Learning Approaches”, 2004.03747,arXiv eess.iv, 2020
[15] L.Wang, and W. Alexander, "COVID-Net: A Tailored Deep Convolutional Neura Network Design for Detection of COVID-19 Cases from Chest Radiography Images." arXiv preprint arXiv: 2003. 09871, 2020.
[16] D. Ioannis, I. Apostolopoulos., B. Tzani, "COVID-19: Automatic detection from X-ray images utilizing Transfer Learning with Convolutional Neural Networks,” arXiv:2003.11617 (20013), 2020
[17] M. Barstugan, U. Ozkaya , S. Ozturk, " Coronavirus (covid-19) classification using CT image by machine learning methods”, arXiv preprint arXiv:2003.09424, 2020
[18] M.Farooq, A. Hafeez, "Covid-resnet:" A deep learning framework for screening of covid19 from radiographs,” arXiv preprint arXiv:2003.14395 , 2020
[19] A. Narin, C. Kaya, and Z. Pamuk, "Automatic Detection of Coronavirus Disease (COVID-[19] Using X-ray Images and Deep Convolutional Neural Networks), ” arXiv preprint arXiv:2003.10849, 2020
[20] J. Zhang, Y. Xie and Y. Chunhua,"COVID-19 Screening on Chest X-ray Images Using Deep Learning based Anomaly Detection,” arXiv:2003.12338v1, 2020
[21] W. Linda, "COVID?Net. A Tailored Deep Convolutional Neural Network Design for Detection of COVID?19 Cases from Chest Radiography Images,” 2020
[22] P. Sethy,. S. Behera, " Detection of Coronavirus Disease (COVID-19) Based on Deep Features", doi:10.20944/preprints 20200 3.0300.v1), 2020
[23] A. Krizhevsky,I. Sutskever , and G. Hinton " ImageNet Classification with Deep Convolutional Neural Networks,” Advances in Neural Information Processing Systems, pp 1-5, 2020
[24] O Russakovsky, J. Deng, H. Su, J. Krause, S Satheesh, S. Ma, Z. Huang, ImageNet Large Scale Visual Recognition Challenge. International Journal of Computer Vision, 2015
[25] C. Szegedy ,W. Liu, Y. Jia,, P. Sermanet, S. Reed, D. Anguelov, V. Vanhoucke, " Going deeper with convolutions, IEEE Conference on Computer Vision and Pattern Recognition (CVPR) pp1-9, 2015
[26] N. Iandola, S. Han, W. Moskewicz , K.Ashraf, J.William , K. Keutzer "SqueezeNet: Alexnet level accuracy with 50x fewer parameters and <0.5 MB model size. "Preprint, submitted https://arxiv.org/abs/ 1602.07360, 2016
[27] N. Japkwowicz, M.Shah, "Evaluating Learning Algorithm: A Classification Perspective,’’Cambridge University press, pp.74-97, 2020
[28] M.Ali, F. Machot, A. Mosa, and P. Grausberg, "A Review of Multi-Class Object Classification for Video Surveillance Systems," In: 7th Conference Proceedings of the GI Autonomous Systems, vol.835, Spain, 2014
You do not have rights to view the full text article.
Please contact administration for subscription to Journal or individual article.
Mail us at support@isroset.org or view contact page for more details.