Full Paper View Go Back
Diagnosis of Chronic Kidney Disease Using Optimised Feature Selection and Ensemble Technique
Olukiran Oyenike Adunni1 , Shoyemi Olufemi Segun2 , Aro Taye Oladele3 , Aiyeniko Olukayode4 , Adedokun Olufemi Adewale5
Section:Research Paper, Product Type: Journal-Paper
Vol.10 ,
Issue.3 , pp.19-25, Jun-2022
Online published on Jun 30, 2022
Copyright Β© Olukiran Oyenike Adunni, Shoyemi Olufemi Segun, Aro Taye Oladele, Aiyeniko Olukayode, Adedokun Olufemi Adewale . This is an open access article distributed under the Creative Commons Attribution License, which permits unrestricted use, distribution, and reproduction in any medium, provided the original work is properly cited.
View this paper at Google Scholar | DPI Digital Library
How to Cite this Paper
- IEEE Citation
- MLA Citation
- APA Citation
- BibTex Citation
- RIS Citation
IEEE Style Citation: Olukiran Oyenike Adunni, Shoyemi Olufemi Segun, Aro Taye Oladele, Aiyeniko Olukayode, Adedokun Olufemi Adewale, βDiagnosis of Chronic Kidney Disease Using Optimised Feature Selection and Ensemble Technique,β International Journal of Scientific Research in Computer Science and Engineering, Vol.10, Issue.3, pp.19-25, 2022.
MLA Style Citation: Olukiran Oyenike Adunni, Shoyemi Olufemi Segun, Aro Taye Oladele, Aiyeniko Olukayode, Adedokun Olufemi Adewale "Diagnosis of Chronic Kidney Disease Using Optimised Feature Selection and Ensemble Technique." International Journal of Scientific Research in Computer Science and Engineering 10.3 (2022): 19-25.
APA Style Citation: Olukiran Oyenike Adunni, Shoyemi Olufemi Segun, Aro Taye Oladele, Aiyeniko Olukayode, Adedokun Olufemi Adewale, (2022). Diagnosis of Chronic Kidney Disease Using Optimised Feature Selection and Ensemble Technique. International Journal of Scientific Research in Computer Science and Engineering, 10(3), 19-25.
BibTex Style Citation:
@article{Adunni_2022,
author = {Olukiran Oyenike Adunni, Shoyemi Olufemi Segun, Aro Taye Oladele, Aiyeniko Olukayode, Adedokun Olufemi Adewale},
title = {Diagnosis of Chronic Kidney Disease Using Optimised Feature Selection and Ensemble Technique},
journal = {International Journal of Scientific Research in Computer Science and Engineering},
issue_date = {6 2022},
volume = {10},
Issue = {3},
month = {6},
year = {2022},
issn = {2347-2693},
pages = {19-25},
url = {https://www.isroset.org/journal/IJSRCSE/full_paper_view.php?paper_id=2817},
publisher = {IJCSE, Indore, INDIA},
}
RIS Style Citation:
TY - JOUR
UR - https://www.isroset.org/journal/IJSRCSE/full_paper_view.php?paper_id=2817
TI - Diagnosis of Chronic Kidney Disease Using Optimised Feature Selection and Ensemble Technique
T2 - International Journal of Scientific Research in Computer Science and Engineering
AU - Olukiran Oyenike Adunni, Shoyemi Olufemi Segun, Aro Taye Oladele, Aiyeniko Olukayode, Adedokun Olufemi Adewale
PY - 2022
DA - 2022/06/30
PB - IJCSE, Indore, INDIA
SP - 19-25
IS - 3
VL - 10
SN - 2347-2693
ER -
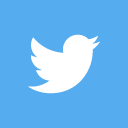
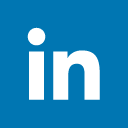
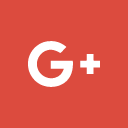
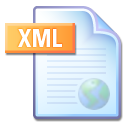
Abstract :
Chronic Kidney Disease (CKD) has been identified as an international challenge in healthcare that is increasing progressively. A survey showed that on average more than two million individuals over the world receive dialysis or transplanting kidney treatment to be alive. Prompt diagnosis of CKD is crucial. Prompt and applicable diagnosis demands the use of techniques in data mining. Recently, techniques now extend to a broad area in the diagnosis of a chronic kidney with importance mainly on accuracy via the simplification of disease by employing a selection of features together with pre-processing methods. This paper presented an optimised feature selection approach using the boosting of ensemble technique for CKD diagnostic model by the introduction of a nature-inspired computation algorithm known as Ant Colony Optimization for the selection of attributes from the CKD dataset. Seven selected learning algorithms were used for classification. The CKD diagnostic model was evaluated using an indigenous dataset collected from Ladoke Akintola University of Technology (LAUTECH) teaching hospital, Ogbomoso and Osogbo, University College HospitPredical (UCH), Ibadan, Oyo State and Obafemi Awolowo University Teaching Hospital. Results showed that the optimised CKD diagnostic model produced the best accuracy of 96.54% in Stage 5 of CKD using logistic regression classifier, the best sensitivity of 0.9650 was obtained in Stage 5 of CKD using logistic regression classifier and the best precision of 0.9700 was obtained in Stage 5 of CKD using logistic regression classifier
Key-Words / Index Term :
Chronic Kidney Disease, Diagnosis, Ensemble, Diagnostic Model
References :
[1] Arasu, S.D., & Thirumalaiselvi, R. "Review of Chronic Kidney Disease based on Data Mining Techniques" International Journal of Applied Engineering Research, Vol. 12, No. 23, pp. 13498β13505, 2017.
[2] Arumugam, V., & Priya, S. B. "Selecting Dominant Features for the Prediction of Early-Stage Chronic Kidney Disease". Intelligent Automation and Soft Computing, Vol. 31, No. 2, pp. 947β959, 2022.
[3] Basar, M. D., & Akan, A. "Detection of chronic kidney disease by using ensemble classifiers". 2017 10th International Conference on Electrical and Electronics Engineering, ELECO 2017, pp. 544β547, 2018.
[4] Chauchan, A. "Enhancing Academic Decision making at Higher Educational Institutions using Classification and Clustering Techniques" International Journal of Scientific Research in Computer Science and Engineering, Vol. 8 No. 2, pp. 39β44, 2020.
[5] Deepashri, K. S., & Kamath, A. "Survey on Techniques of Data Mining and its Applications". International Journal of Emerging Research in Management & Technology, Vol. 9359 No. 2, pp. 198β201, 2017.
[6] Gopika, S. & Vanitha, M. `"Survey on Prediction of Kidney Disease by using Data Mining Techniques" International Journal of Advanced Research in Computer and Communication Engineering, Vol. 6, No. 1, pp. 198β201. 2019.
[7] Hussain, K., Mohd Salleh, M. N., Cheng, S., & Shi, Y. "Metaheuristic research: a comprehensive survey" Artificial Intelligence Review, Vol. 52, No. 4, pp. 2191β2233, 2019.
[8] Imani, M. B., Pourhabibi, T., Keyvanpour, M. R., & Azmi, R. "A New Feature Selection Method Based on Ant Colony and Genetic Algorithm on Persian Font Recognition". International Journal of Machine Learning and Computing, Vol. 2, No. 3, pp. 278β282, 2012.
[9] O. A., Adetunmbi, A. O., Ogunrinde, R. B., & Badeji-Ajisafe, B. "Development of an Ensemble Approach to Chronic Kidney Disease Diagnosis" Scientific African, Vol. 8, pp. 1β15, 2020.
[10] Komal, N, K., Tulasi, R. L., & Vigneswari, D. "An Ensemble Multi-Model Technique for Predicting Chronic Kidney Disease". International Journal of Electrical and Computer Engineering (IJECE), Vol. 9, No. 2, pp13-21. 2019.
[11] Krishnaveni, N., & Radha, V. "Feature Selection Algorithms for Data Mining Classification: A Survey". Indian Journal of Science and Technology, Vol. 12, No. 6, pp.1β11, 2019.
[12] Kumar, V. & Minz, S. "Feature Selection: A literature Review." The Smart Computing Review, Vol. 4, No. 3, pp. 211β229, 2014.
[13] Luyckx, V. A., Tonelli, M., & Stanifer, J. W. "The global burden of kidney disease and the sustainable development goals". Bulletin of the World Health Organization, Vol. 96, No. 6, pp. 414β422, 2018.
[14] Oladeji, F A, Idowu, P A, Egejuru, N. (2019). "Model for Predicting the Risk of Kidney Stone using Data Mining Techniques". International Journal of Computer Application, Vol. 182, No. 38, pp.36β56, 2019.
[15] Patel, A., Shreya, P., & Amin. "A Survey on Heuristic Based Approach for Privacy-Preserving in Data Mining". International Journal of Scientific Research in Computer Science and Engineering, Vol. 5, No. 5, pp. 21β25, 2017.
[16] Ram, Shrawan and Doegar, A. "A Comparative Study of Data Mining Techniques for Predicting Disease Using Statlog Heart Disease Database". International Journal of Advanced Research in Computer Science and Software Engineering, Vol., No. 6, 1202β1210, 2015
[17] Shardlow, M. "An Analysis of Feature Selection Techniques". The University of Manchester, Vol. 14, No.1, pp.1β7, 2016.
[18] Tabassum, S., Mamatha B.& Majumdar, J. "Analysis and Prediction of Chronic Kidney Disease using Data Mining Techniques". International Journal of Engineering Research in Computer Science and Engineering, Vol. 4, No. 9, pp.25β32, 2017
[19] Teng, X., & Gong, Y. "Research on Application of Machine Learning in Data Mining". IOP Conference Series: Materials Science and Engineering, Vol. 392, No. 6. 2018.
[20] Tripathi, A., Nadaf, A., & Yadav, A. K. (2020). "Identification of the Stages of Chronic Kidney Disease Using Data Mining Approach". International Research Journal of Modernization in Engineering Technology and Science, Vol. 2, No. 8, pp.463β467, 2020.
[21] Wang, W., Chakraborty, G., & Chakraborty, B. "Predicting the Risk of Chronic Kidney Disease ( CKD ) Using Machine Learning Algorithm". Applied Sciences, Vol. 11, No.202, pp.1β17, 2021.
You do not have rights to view the full text article.
Please contact administration for subscription to Journal or individual article.
Mail us atΒ support@isroset.org or view contact page for more details.