Full Paper View Go Back
Ghada Rahal1 , Islem Dridi2
- Department of management from Ecole Supérieur des Sciences Economiques et Commerciales de Tunis, Tunisia.
- Department of management from Ecole Supérieur des Sciences Economiques et Commerciales de Tunis, Tunisia.
Section:Review Paper, Product Type: Journal-Paper
Vol.11 ,
Issue.1 , pp.47-50, Feb-2023
Online published on Feb 28, 2023
Copyright © Ghada Rahal, Islem Dridi . This is an open access article distributed under the Creative Commons Attribution License, which permits unrestricted use, distribution, and reproduction in any medium, provided the original work is properly cited.
View this paper at Google Scholar | DPI Digital Library
How to Cite this Paper
- IEEE Citation
- MLA Citation
- APA Citation
- BibTex Citation
- RIS Citation
IEEE Style Citation: Ghada Rahal, Islem Dridi, “Comparing Belief KNN, Adaptive KNN, Fuzzy KNN and System classification hybrid Algorithms: A comprehensive guide to understanding K-Nearest Neighbors,” International Journal of Scientific Research in Computer Science and Engineering, Vol.11, Issue.1, pp.47-50, 2023.
MLA Style Citation: Ghada Rahal, Islem Dridi "Comparing Belief KNN, Adaptive KNN, Fuzzy KNN and System classification hybrid Algorithms: A comprehensive guide to understanding K-Nearest Neighbors." International Journal of Scientific Research in Computer Science and Engineering 11.1 (2023): 47-50.
APA Style Citation: Ghada Rahal, Islem Dridi, (2023). Comparing Belief KNN, Adaptive KNN, Fuzzy KNN and System classification hybrid Algorithms: A comprehensive guide to understanding K-Nearest Neighbors. International Journal of Scientific Research in Computer Science and Engineering, 11(1), 47-50.
BibTex Style Citation:
@article{Rahal_2023,
author = {Ghada Rahal, Islem Dridi},
title = {Comparing Belief KNN, Adaptive KNN, Fuzzy KNN and System classification hybrid Algorithms: A comprehensive guide to understanding K-Nearest Neighbors},
journal = {International Journal of Scientific Research in Computer Science and Engineering},
issue_date = {2 2023},
volume = {11},
Issue = {1},
month = {2},
year = {2023},
issn = {2347-2693},
pages = {47-50},
url = {https://www.isroset.org/journal/IJSRCSE/full_paper_view.php?paper_id=3051},
publisher = {IJCSE, Indore, INDIA},
}
RIS Style Citation:
TY - JOUR
UR - https://www.isroset.org/journal/IJSRCSE/full_paper_view.php?paper_id=3051
TI - Comparing Belief KNN, Adaptive KNN, Fuzzy KNN and System classification hybrid Algorithms: A comprehensive guide to understanding K-Nearest Neighbors
T2 - International Journal of Scientific Research in Computer Science and Engineering
AU - Ghada Rahal, Islem Dridi
PY - 2023
DA - 2023/02/28
PB - IJCSE, Indore, INDIA
SP - 47-50
IS - 1
VL - 11
SN - 2347-2693
ER -
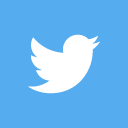
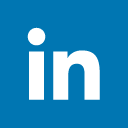
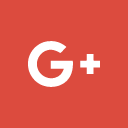
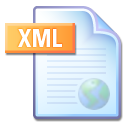
Abstract :
This research is about to present and compare four of K-Nearest Neighbors (KNN) versions (Belief KNN, Adaptive KNN, Fuzzy KNN, System classification hybrid), that have recently been studied.
Furthermore, indicate their limits. Computers may now ingest data without even being entirely programmed thanks to machine learning. Unsupervised learning and supervised are both types for machine learning.
Computers adopt an objective in supervised learning which translates an input to an output based on training input-output pairs. K-Nearest Neighbors is among the most successful and frequently used in supervised learning methods.
This study is the first step in trying to clarify the difference in algorithm between KNN versions. Results will show benefits and drawbacks of these four KNN varieties, otherwise, representing the critics for each KNN type.
This work can be beneficial for researchers learning to improve their studies in supervised machines, by showing how KNN versions whether explain the research and subsequent comparison measures for distance regulation.
Key-Words / Index Term :
Machine learning, Supervised learning, KNN, Fuzzy KNN, System classification hybrid, Belief KNN, Adaptive KNN
References :
[1]. John McCarthy, “What is artificial intelligence?”, 2004.
[2]. Daniel Belanche, Luis V. Casaló Bach Xuan Tran et al., “Global Evolution of Research in Artificial Intelligence in Health and Medicine: A Bibliometric Study”, Journal of Clinical Medicine, 8, 360, 2019.
[3]. Daniel Belanche, Luis Vicente Casaló Ariño, Carlos Flavian, “Artificial Intelligence in FinTech: understanding robo-advisors adoption among customers”, Industrial Management & Data Systems, 119(7), 1411-1430, 2019.
[4]. Flavián Sumit Das, Aritra dey, Akash Pal, “Applications of Artificial Intelligence in Machine Learning: Review and Prospect,” International Journal of Computer Applications (0975 – 8887), Volume 115 – No. 9, April 2015.
[5]. Chashif Syadzali, Suryono Suryono2, and Jatmiko Endro Suseno2, “Business Intelligence using the K-Nearest Neighbor Algorithm to Analyze Customer Behavior in Online Crowdfunding Systems,” E3S Web of Conferences 202, 16005, 2020.
[6]. Zhiwei Huang et al., “Research on KNN Algorithm of Charging Device Based on Attack Recognition Method,” Journal of Physics: Conference Series 1646 (2020) 012047, 2020.
[7]. Zhiwei Huang et al., “Research on KNN Algorithm of Charging Device Based on Attack Recognition Method,” Journal of Physics: Conference Series 1646 012047, 2020.
[8]. D Kurniadi et al., “The prediction of scholarship recipients in higher education using k-Nearest neighbor algorithm,” IOP Conf. Series: Materials Science and Engineering 434 012039. Conference, 2018.
[9]. James M. Keller, Michel R. Gray, James A. Givens, JR, “A Fuzzy K-Nearest Neighbor Algorithm,” IEEE transcations on systems, man, and cybernetics, vol. smc-15, no. 4, 1985.
[10]. Zhun-ga Liu, Quan Pan, Jean Dezert, “A new belief-based K-nearest neighbor classification method,” Pattern Recognition 46 834–844, 2013.
[11]. Puning Zhao, Lifeng Lai, “Efficient Classification with Adaptive KNN,” The Thirty-Fifth AAAI Conference on Artificial Intelligence (AAAI-21).
[12]. Mehmet Aci, Cigdem _ Inan, Mutlu Avci, “A hybrid classification method of k nearest neighbor, Bayesian methods and genetic algorithm”, Expert Systems with Applications, 2010.
[13]. Emmanuel Ramasso, Michèle Rombaut, Noureddine Zerhouni, “Joint Prediction of Continuous and Discrete States in Time-Series Based on Belief Functions,” IEEE Transactions on Cybernetics, IEEE, 43 (1), pp.37-50, 2013.
You do not have rights to view the full text article.
Please contact administration for subscription to Journal or individual article.
Mail us at support@isroset.org or view contact page for more details.