Full Paper View Go Back
Community Structure Detection in Social Networking Data Using Text Mining Approach
S.Arora 1 , P. Shukla2 , N. Karankar3
- Dept. of Computer Science and Engineering, IET-DAVV, Indore, India.
- Dept. of Computer Science and Engineering, IET-DAVV, Indore, India.
- Dept. of Computer Science and Engineering, IET-DAVV, Indore, India.
Correspondence should be addressed to: shrutiaro26@gmail.com.
Section:Research Paper, Product Type: Isroset-Journal
Vol.5 ,
Issue.4 , pp.9-15, Aug-2017
Online published on Aug 30, 2017
Copyright © S.Arora, P. Shukla, N. Karankar . This is an open access article distributed under the Creative Commons Attribution License, which permits unrestricted use, distribution, and reproduction in any medium, provided the original work is properly cited.
View this paper at Google Scholar | DPI Digital Library
How to Cite this Paper
- IEEE Citation
- MLA Citation
- APA Citation
- BibTex Citation
- RIS Citation
IEEE Style Citation: S.Arora, P. Shukla, N. Karankar, “Community Structure Detection in Social Networking Data Using Text Mining Approach,” International Journal of Scientific Research in Computer Science and Engineering, Vol.5, Issue.4, pp.9-15, 2017.
MLA Style Citation: S.Arora, P. Shukla, N. Karankar "Community Structure Detection in Social Networking Data Using Text Mining Approach." International Journal of Scientific Research in Computer Science and Engineering 5.4 (2017): 9-15.
APA Style Citation: S.Arora, P. Shukla, N. Karankar, (2017). Community Structure Detection in Social Networking Data Using Text Mining Approach. International Journal of Scientific Research in Computer Science and Engineering, 5(4), 9-15.
BibTex Style Citation:
@article{Shukla_2017,
author = {S.Arora, P. Shukla, N. Karankar},
title = {Community Structure Detection in Social Networking Data Using Text Mining Approach},
journal = {International Journal of Scientific Research in Computer Science and Engineering},
issue_date = {8 2017},
volume = {5},
Issue = {4},
month = {8},
year = {2017},
issn = {2347-2693},
pages = {9-15},
url = {https://www.isroset.org/journal/IJSRCSE/full_paper_view.php?paper_id=430},
publisher = {IJCSE, Indore, INDIA},
}
RIS Style Citation:
TY - JOUR
UR - https://www.isroset.org/journal/IJSRCSE/full_paper_view.php?paper_id=430
TI - Community Structure Detection in Social Networking Data Using Text Mining Approach
T2 - International Journal of Scientific Research in Computer Science and Engineering
AU - S.Arora, P. Shukla, N. Karankar
PY - 2017
DA - 2017/08/30
PB - IJCSE, Indore, INDIA
SP - 9-15
IS - 4
VL - 5
SN - 2347-2693
ER -
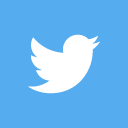
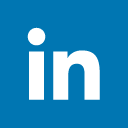
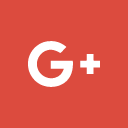
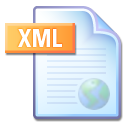
Abstract :
In data mining techniques some of the problems are resolved using the visualization techniques. Among them some of techniques are derived from the graph theory and transparent data modelling. The data structures such as decision trees and semantic graph representation is one of the key implementation of the graph based solution development. Among these technique one of the mathematical model termed as the community detection is a part of data mining solution discovery technique. Data mining techniques are used for finding the application centric patterns recovery from the raw set of data. Additionally the community detection technique is a visual technique for performing the unsupervised learning. During community detection the data objects are keeping connected to represent the bounding among them. Therefore in order to perform categorization task in automatic manner this technique can be employed in different nature of data. In this presented work the social media text is used for community detection. Communities are the group of objects that are highly similar in their properties. Therefore an algorithm is proposed in this work, that first refines the text content, then the text features are computed form raw text. In next the data is evaluated to find the number of possible communities in the data and finally the data is grouped in the communities and their visualization is performed. The proposed algorithm not only used to find the community structure from the data that also provides the relationship among two different communities. The experimental results in terms of precision, recall, f-measures demonstrate the proposed model is efficient and accurate as compared to traditional clustering algorithms namely the k-means clustering.
Key-Words / Index Term :
Clustering, Community Detection, Complex Network, Tweeter, Data Mining
References :
[1] A.Biswas, B.Biswas, “Investigating community structure in perspective of ego network”, Expert Systems with Applications, 42 (2015) 6913–6934, 2015 ,Elsevier Ltd
[2] Arnaboldi, Passarella, Conti, Pezzoni “Analysis of ego network structure in online social network”,pp. 31-40, 2012
[3] Y.Wang, &L.Gao“An edge-based clustering algorithm to detect social circles in ego networks”, Journal of Computers, Vol 8,2013
[4] A. Mislove, M. Marcon, K. P. Gummadi, P. Druschel, and B. Bhattacharjee. “Measurement and analysis of online social networks”. In IMC ’07, pp 29–42, 2007.
[5] G. Palla, I. Der´enyi, I. Farkas, and T. Vicsek. “Uncovering the overlapping community structure of complex networks in nature and society”. Nature, 435(7043):pp814–818, 2005.
[6] J. Leskovec, L. Adamic, and B. Huberman. “The dynamics of viral marketing”. TWeb, ACM, 1(1), 2007.
[7] S. L. Feld. “The focused organization of social ties”. Am. J. of Sociology, 86(5):1015–1035, 1981.
[8] G. Flake, S. Lawrence, and C. Giles.“ Efficient identification of web communities”. In KDD ’00, pages 150–160, 2000.
[9] M. Girvan and M. Newman. “Community structure in social and biological networks”. PNAS, 99(12):7821–7826, 2002.
[10] M. S. Granovetter. “The strength of weak ties”. Am. J. of Sociology, 78:1360–1380, 1973.
[11] R. Solanki, “Principle of Data Mining”, McGraw-Hill Publication, India, pp. 108-128, 1998.
[12] Liu, H., &juan Ban, X. .“Clustering by growing incremental self-organizingneural network”. Expert Systems with Applications, 42:4965–4981,2015,ElsevierLtd
[13] R.R.Khorasgani., J.Chen, &O.R.Zaïane, O. R. “Top leaders community detectionapproach” in information networks. In International conference on knowledge discovery and data mining,KDD’10: ACM,Citeseer, (pp. 9),2010
You do not have rights to view the full text article.
Please contact administration for subscription to Journal or individual article.
Mail us at support@isroset.org or view contact page for more details.