Full Paper View Go Back
Ensemble based J48 and random forest based C6H6 air pollution detection
Gagandeep Kaur1 , Harmanpreet Kaur2
- Computer Science & Engineering, Sri Sai college of Engineering and Technology, Manawala, Amritsar, India.
- Computer Science & Engineering, Sri Sai college of Engineering and Technology, Manawala, Amritsar, India.
Section:Research Paper, Product Type: Isroset-Journal
Vol.6 ,
Issue.2 , pp.41-50, Apr-2018
CrossRef-DOI: https://doi.org/10.26438/ijsrcse/v6i2.4150
Online published on Apr 30, 2018
Copyright © Gagandeep Kaur, Harmanpreet Kaur . This is an open access article distributed under the Creative Commons Attribution License, which permits unrestricted use, distribution, and reproduction in any medium, provided the original work is properly cited.
View this paper at Google Scholar | DPI Digital Library
How to Cite this Paper
- IEEE Citation
- MLA Citation
- APA Citation
- BibTex Citation
- RIS Citation
IEEE Style Citation: Gagandeep Kaur, Harmanpreet Kaur, “Ensemble based J48 and random forest based C6H6 air pollution detection,” International Journal of Scientific Research in Computer Science and Engineering, Vol.6, Issue.2, pp.41-50, 2018.
MLA Style Citation: Gagandeep Kaur, Harmanpreet Kaur "Ensemble based J48 and random forest based C6H6 air pollution detection." International Journal of Scientific Research in Computer Science and Engineering 6.2 (2018): 41-50.
APA Style Citation: Gagandeep Kaur, Harmanpreet Kaur, (2018). Ensemble based J48 and random forest based C6H6 air pollution detection. International Journal of Scientific Research in Computer Science and Engineering, 6(2), 41-50.
BibTex Style Citation:
@article{Kaur_2018,
author = {Gagandeep Kaur, Harmanpreet Kaur},
title = {Ensemble based J48 and random forest based C6H6 air pollution detection},
journal = {International Journal of Scientific Research in Computer Science and Engineering},
issue_date = {4 2018},
volume = {6},
Issue = {2},
month = {4},
year = {2018},
issn = {2347-2693},
pages = {41-50},
url = {https://www.isroset.org/journal/IJSRCSE/full_paper_view.php?paper_id=606},
doi = {https://doi.org/10.26438/ijcse/v6i2.4150}
publisher = {IJCSE, Indore, INDIA},
}
RIS Style Citation:
TY - JOUR
DO = {https://doi.org/10.26438/ijcse/v6i2.4150}
UR - https://www.isroset.org/journal/IJSRCSE/full_paper_view.php?paper_id=606
TI - Ensemble based J48 and random forest based C6H6 air pollution detection
T2 - International Journal of Scientific Research in Computer Science and Engineering
AU - Gagandeep Kaur, Harmanpreet Kaur
PY - 2018
DA - 2018/04/30
PB - IJCSE, Indore, INDIA
SP - 41-50
IS - 2
VL - 6
SN - 2347-2693
ER -
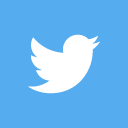
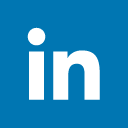
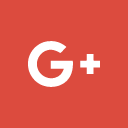
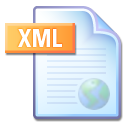
Abstract :
Air pollution has become a critical challenge for today’s world. An efficient monitoring of air pollution gases can help to reduce the pollution in the air. Air pollution cause us many diseases such as cancer etc. Benzene (C6H6) turn out to be more challenging issue in our society, because its sensors are costly to deploy and also not feasible to add too many sensors in urban areas. Therefore, in this paper an efficient monitoring of C6H6 gas has been done by using the ensemble approach. It is feasible to estimate C6H6 by using machine learning because there exists relationship between gases. Extensive experiments have been carried out to evaluate the effectiveness of the proposed technique. It has been found that the proposed technique significantly improves the performance of existing machine learning techniques.
Key-Words / Index Term :
C6H6 • Air pollution • Random forest • Machine learning
References :
[1]. Airbase - the european air quality database. http: //www.eea.europa.eu/data-and-maps/data/airbase-the-european-air-quality-database-8. Accessed: March 17, 2017.
[2]. C6H6pubchem open chemistry database. https://pubchem.ncbi.nlm. nih.gov/compound/C6H6. Accessed: March 17, 2017.
[3]. C6H6-B.csv national institute of standards and technology. https://www. nist.gov/file/36031. Accessed: March 17, 2017.
[4]. C6H6-nrm-part5.test.csv petravidnerovasensorsscikittest. https://github.com/PetraVidnerova/SensorsScikitTest/blob/master/ data/C6H6-nrm-part5.test.csv. Accessed: March 17, 2017.
[5]. Shahid Ali and Sreenivas Sremath Tirumala. Performance analysis of svm ensemble methods for air pollution data. In Proceedings of the 8th InternationalConference on Signal Processing Systems, pages 212–216. ACM, 2016.
[6]. Yun Bai, Yong Li, Xiaoxue Wang, JingjingXie, and Chuan Li. Air pollutants concentrations forecasting using back propagation neural network based on wavelet decomposition with meteorological conditions. Atmospheric pollution research, 7(3):557–566, 2016.
[7]. IliasBougoudis, Konstantinos Demertzis, and LazarosIliadis. Fast and low cost prediction of extreme air pollution values with hybrid unsupervised learning. Integrated Computer-Aided Engineering, 23(2):115–127, 2016.
[8]. IliasBougoudis, Konstantinos Demertzis, and LazarosIliadis. Hisycol a hybrid computational intelligence system for combined machine learning: the case of air pollution modeling in athens. Neural Computing and Applications, 27(5):1191– 1206, 2016.
[9]. Cole Brokamp, Roman Jandarov, M.B. Rao, Grace LeMasters, and Patrick Ryan.
Exposure assessment models for elemental components of particulate matter in an
urban environment: A comparison of regression and random forest approaches.
Atmospheric Environment, 151:1 – 11, 2017.
[10]. Ling Chen, Yaya Cai, Yifang Ding, MingqiLv, Cuili Yuan, and Gencai Chen. Spatially fine-grained urban air quality estimation using ensemble semi-supervised learning and pruning. In Proceedings of the 2016 ACM International Joint Conference on Pervasive and Ubiquitous Computing, pages 1076–1087. ACM, 2016. 38
[11]. S De Vito, E Massera, M Piga, L Martinotto, and G Di Francia. On field calibration of an electronic nose for C6H6 estimation in an urban pollution monitoring scenario. Sensors and Actuators B: Chemical, 129(2):750–757, 2008.
[12]. Saverio De Vito, Grazia Fattoruso, Matteo Pardo, Francesco Tortorella, and Girolamo Di Francia. Semi supervised learning methods in artificial olfaction: A novel approach to classification problems and drift counteraction. IEEE SensorsJournal, 12(11):3215–3224, 2012.
[13]. Saverio De Vito, Marco Piga, Luca Martinotto, and Girolamo Di Francia. Co, no2 and nox urban pollution monitoring with on field calibrated electronic nose
[14]. Qingli Dong, Yong Wang, and Peizhi Li. Multifractal behavior of an air pollutant time series and the relevance to the predictability. Environmental Pollution, 222:444–457, 2017.
[15]. Husanbir Singh Pannu, Dilbag Singh, and Avleen Kaur Malhi. "Improved particle swarm optimization based adaptive neuro‐fuzzy inference system for benzene detection." CLEAN–Soil, Air, Water (2018).
[16]. Eleni Fotopoulou, Anastasios Zafeiropoulos, Dimitris Papaspyros, Panagiotis Hasapis, George Tsiolis, ThanassisBouras, SpyrosMouzakitis, and Norma Zanetti. Linked data analytics in interdisciplinary studies: The health impact of air pollution in urban areas. IEEE Access, 4:149–164, 2016.
[17]. Yoav Freund and Llew Mason. The alternating decision tree learning algorithm.
In icml, volume 99, pages 124–133, 1999.
[18]. N. Goudarzi, D. Shahsavani, F. Emadi-Gandaghi, and M. Arab Chamjangali. Application of random forests method to predict the retention indices of some polycyclic aromatic hydrocarbons. Journal of Chromatography A, 1333:25 – 31, 2014.
[19]. Hui Hu, Sandie Ha, Jeffrey Roth, Greg Kearney, Evelyn O. Talbott, and XiaohuiXu. Ambient air pollution and hypertensive disorders of pregnancy: A systematic review and meta-analysis. Atmospheric Environment, 97:336 – 345, 2014.
[20]. Ibrahim Anwar Ibrahim and Tamer Khatib. A novel hybrid model for hourly global solar radiation prediction using random forests method and firefly algorithm. Energy Conversion and Management, 138:413 – 425, 2017. 39
[21]. Younoh Kim, Scott Knowles, James Manley, and Vlad Radoias. Long-run health consequences of air pollution: Evidence from indonesia’s forest fires of 1997. Economics & Human Biology, 26:186 – 198, 2017.
[22]. Ibai Lana, Javier Del Ser, Ales Padro, Manuel Velez, and Carlos Casanova-Mateo. The role of local urban traffic and meteorological conditions in air pollution: A data-based case study in madrid, spain. Atmospheric Environment, 145:424 – 438, 2016.
[23]. SirkkuManninen, Vitali Zverev, Igor Bergman, and Mikhail V. Kozlov. Consequences of long-term severe industrial pollution for aboveground carbon and nitrogen pools in northern taiga forests at local and regional scales. Science of TheTotal Environment, 536:616 – 624, 2015.
[24]. Nguyen Thi Trang Nhung, HereshAmini, Christian Schindler, MeltemKutlar Joss,
Tran Minh Dien, Nicole Probst-Hensch, Laura Perez, and Nino KÃ 1 4nzli. Shortterm association between ambient air pollution and pneumonia in children: A systematic review and meta-analysis of time-series and case-crossover studies. Environmental Pollution, 230:1000 – 1008, 2017.
[25]. PJ García Nieto, Elías F Combarro, JJ del Coz Díaz, and Elena Montañés. A svm-based regression model to study the air quality at local scale in oviedo urban area (northern spain): A case study. Applied Mathematics and Computation, 219(17):8923–8937, 2013.
[26]. Pavel G Polishchuk, Eugene N Muratov, Anatoly G Artemenko, Oleg G Kolumbin, Nail N Muratov, and Victor E Kuzmin. Application of random forest approach to qsar prediction of aquatic toxicity. Journal of chemical information and modeling, 49(11):2481–2488, 2009.
[27]. Kanchan Prasad, Amit Kumar Gorai, and Pramila Goyal. Development of anfis models for air quality forecasting and input optimization for reducing the computational cost and time. Atmospheric environment, 128:246–262, 2016.
[28]. Dilbag Singh and Vijay Kumar. "Modified gain intervention filter based dehazing technique." Journal of Modern Optics 64, no. 20 (2017): 2165-2178.
[29]. Dilbag Singh and Vijay Kumar. "Comprehensive survey on haze removal techniques." Multimedia Tools and Applications (2017): 1-26.
[30]. Dilbag Singh and Vijay Kumar. "Dehazing of remote sensing images using improved restoration model based dark channel prior." The Imaging Science Journal 65, no. 5 (2017): 282-292.
[31]. Dilbag Singh and Vijay Kumar. "Dehazing of remote sensing images using fourth-order partial differential equations based trilateral filter." IET Computer Vision (2017).
[32]. Dilbag Singh and Vijay Kumar. "Defogging of road images using gain coefficient-based trilateral filter." Journal of Electronic Imaging 27, no. 1 (2018): 013004.
[33]. Dilbag Singh and Vijay Kumar. "Single image haze removal using integrated dark and bright channel prior." Modern Physics Letters B (2018): 1850051.
[34]. Husanbir Singh Pannu, Dilbag Singh, and Avleen Kaur Malhi. "Multi-objective particle swarm optimization-based adaptive neuro-fuzzy inference system for benzene monitoring." Neural Computing and Applications: 1-11.
[35]. Ian H Witten, Eibe Frank, Mark A Hall, and Christopher J Pal. Data Mining:Practical machine learning tools and methods. Morgan Kaufmann, 2016.
[36]. John Yen and Reza Langari. Fuzzy logic: intelligence, control, and information. Prentice-Hall, Inc., 1998.
[37]. Yilmaz Yildirim and MahmutBayramoglu. Adaptive neuro-fuzzy based modelling for prediction of air pollution daily levels in city of zonguldak. Chemosphere,63(9):1575–1582, 2006.
[38]. Yongheng Zhao and Yanxia Zhang. Comparison of decision tree methods for finding active objects. Advances in Space Research, 41(12):1955–1959, 2008.
You do not have rights to view the full text article.
Please contact administration for subscription to Journal or individual article.
Mail us at support@isroset.org or view contact page for more details.