Full Paper View Go Back
Predictive Machine Learning Model for Detection and Classification of Diabetes
T. Parvin1 , T. Nasrin2 , J. Khatun3 , M. Chatterjee4
Section:Research Paper, Product Type: Journal-Paper
Vol.7 ,
Issue.9 , pp.11-17, Sep-2021
Online published on Sep 30, 2021
Copyright © T. Parvin, T. Nasrin, J. Khatun, M. Chatterjee . This is an open access article distributed under the Creative Commons Attribution License, which permits unrestricted use, distribution, and reproduction in any medium, provided the original work is properly cited.
View this paper at Google Scholar | DPI Digital Library
How to Cite this Paper
- IEEE Citation
- MLA Citation
- APA Citation
- BibTex Citation
- RIS Citation
IEEE Style Citation: T. Parvin, T. Nasrin, J. Khatun, M. Chatterjee, “Predictive Machine Learning Model for Detection and Classification of Diabetes,” International Journal of Scientific Research in Multidisciplinary Studies , Vol.7, Issue.9, pp.11-17, 2021.
MLA Style Citation: T. Parvin, T. Nasrin, J. Khatun, M. Chatterjee "Predictive Machine Learning Model for Detection and Classification of Diabetes." International Journal of Scientific Research in Multidisciplinary Studies 7.9 (2021): 11-17.
APA Style Citation: T. Parvin, T. Nasrin, J. Khatun, M. Chatterjee, (2021). Predictive Machine Learning Model for Detection and Classification of Diabetes. International Journal of Scientific Research in Multidisciplinary Studies , 7(9), 11-17.
BibTex Style Citation:
@article{Parvin_2021,
author = {T. Parvin, T. Nasrin, J. Khatun, M. Chatterjee},
title = {Predictive Machine Learning Model for Detection and Classification of Diabetes},
journal = {International Journal of Scientific Research in Multidisciplinary Studies },
issue_date = {9 2021},
volume = {7},
Issue = {9},
month = {9},
year = {2021},
issn = {2347-2693},
pages = {11-17},
url = {https://www.isroset.org/journal/IJSRMS/full_paper_view.php?paper_id=2514},
publisher = {IJCSE, Indore, INDIA},
}
RIS Style Citation:
TY - JOUR
UR - https://www.isroset.org/journal/IJSRMS/full_paper_view.php?paper_id=2514
TI - Predictive Machine Learning Model for Detection and Classification of Diabetes
T2 - International Journal of Scientific Research in Multidisciplinary Studies
AU - T. Parvin, T. Nasrin, J. Khatun, M. Chatterjee
PY - 2021
DA - 2021/09/30
PB - IJCSE, Indore, INDIA
SP - 11-17
IS - 9
VL - 7
SN - 2347-2693
ER -
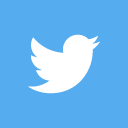
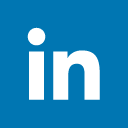
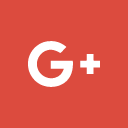
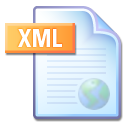
Abstract :
Many of the intriguing and relevant uses of machine learning may be seen in a medical organization. The concept of machine learning is quickly gaining attention in the healthcare industry. The research and analysis on the medical datasets facilitate people to take proper precautions and care so that diseases can be prevented. Different methodologies are extensively employed in the development of disease prediction decision support systems using a set of medical datasets. This paper aims to explore the different types of machine learning algorithms that can help in the prediction and decision-making of diseases. In this paper, we focus on the early prediction of diabetes. Diabetes is one of the world`s fastest-growing diseases, and it necessitates constant monitoring. To analyze this, we consider several machine learning techniques that can aid in the early detection of this disease.
Key-Words / Index Term :
Healthcare; Machine Learning; Diabetes; Random forest; Classification
References :
[1] Aditya Tomar, Disease Prediction System using data mining techniques, in International Journal of Advanced Research in computer and Communication Engineering, ISO 3297, July 2016.
[2] Dr. B.Srinivasan, K.Pavya, A study on data mining prediction techniques in healthcare sector, in International Research Journal of Engineering and Technology (IRJET), March-2016.
[3] Jensen, P., Jensen, L. & Brunak, S. Mining electronic health records: towards better research applications and clinical care. Nat Rev Genet 13, 395–405 (2012). https://doi.org/10.1038/nrg3208 .
[4] Yulei wang1, Jun yang2, Viming.Big Health Application System based on Health Internet of Things and Big Data. IEEE Access PP(99):1-1,DOI: 10.1109/ACCESS.2016.2638449
[5] Saranya P and Satheeskumar B. A Survey on Feature Selection of Cancer Disease Using Data Mining Techniques, International Journal of Computer Science and Mobile Computing, Vol.5 Issue.5, May- 2016, pg. 713-719.
[6] Dr Saravana kumar N M, Eswari T, Sampath P and Lavanya S,” Predictive Methodology for Diabetic Data Analysis in Big Data”, 2nd International Symposium on Big Data and Cloud Computing,2015.
[7]
[8] Aiswarya Iyer, S. Jeyalatha and Ronak Sumbaly,” Diagnosis of Diabetes Using Classification Mining Techniques”, International Journal of Data Mining & Knowledge Management Process (IJDKP) Vol.5, No.1, January 2015.
[9] K.VijiyaKumar, B.Lavanya, I.Nirmala, S.Sofia Caroline, "Random Forest Algorithm for the Prediction of Diabetes ".Proceeding of International Conference on Systems Computation Automation and Networking, 2019.
[10] Nonso Nnamoko, Abir Hussain, David England, "Predicting Diabetes Onset: an Ensemble Supervised Learning Approach ". IEEE Congress on Evolutionary Computation (CEC), 2018
[11] Deeraj Shetty, Kishor Rit, Sohail Shaikh, Nikita Patil, "Diabetes Disease Prediction Using Data Mining ".International Conference on Innovations in Information, Embedded and Communication Systems (ICIIECS), 2017.
[12] Dua, D. and Graff, C. (2019). UCI Machine Learning Repository [http://archive.ics.uci.edu/ml]. Irvine, CA: University of California, School of Information and Computer Science.
[13] Dua, D. and Graff, C. (2019). UCI Machine Learning Repository [http://archive.ics.uci.edu/ml]. Irvine, CA: University of California, School of Information and Computer Science.
[14] Rahman, M.M.; Davis, D.N. Addressing the class imbalance problem in medical datasets. Int. J. Mach. Learn. Comput. 2013, 3, 224–228.
[15] Guo, X.; Yin, Y.; Dong, C.; Yang, G.; Zhou, G. On the Class Imbalance Problem. In Proceedings of the 2008 Fourth International Conference on Natural Computation, Jinan, China, 18–20 October 2008; Volume 4, pp. 192–201.
[16] Bunkhumpornpat, C.; Sinapiromsaran, K.; Lursinsap, C. MUTE: Majority under-sampling technique. In Proceedings of the 2011 8th International Conference on Information, Communications & Signal Processing; Institute of Electrical and Electronics Engineers (IEEE), Singapore, 13–16 December 2011; pp. 1–4.
[17] Chawla, N.V.; Bowyer, K.W.; Hall, L.O.; Kegelmeyer,W.P. SMOTE: Synthetic minority over-sampling technique. J. Artif. Intell. Res. 2002, 16, 321–357.
[18] M.M. Mastoli, U.R. Pol, Rahul D. Patil, “Reasoning with Certainty Factor for Prediction of Diabetes Disease on Machine Learning Platform,” International Journal of Scientific Research in Computer Science and Engineering, Vol.8, Issue.1, pp.93-97, 2020
You do not have rights to view the full text article.
Please contact administration for subscription to Journal or individual article.
Mail us at support@isroset.org or view contact page for more details.