Full Paper View Go Back
Identification and Classification of Oil Palm Maturity Using Machine Learning Techniques
Murinto 1 , M. Rosyda2
Section:Research Paper, Product Type: Journal-Paper
Vol.8 ,
Issue.1 , pp.56-62, Jan-2022
Online published on Jan 31, 2022
Copyright © Murinto, M. Rosyda . This is an open access article distributed under the Creative Commons Attribution License, which permits unrestricted use, distribution, and reproduction in any medium, provided the original work is properly cited.
View this paper at Google Scholar | DPI Digital Library
How to Cite this Paper
- IEEE Citation
- MLA Citation
- APA Citation
- BibTex Citation
- RIS Citation
IEEE Style Citation: Murinto, M. Rosyda, “Identification and Classification of Oil Palm Maturity Using Machine Learning Techniques,” International Journal of Scientific Research in Multidisciplinary Studies , Vol.8, Issue.1, pp.56-62, 2022.
MLA Style Citation: Murinto, M. Rosyda "Identification and Classification of Oil Palm Maturity Using Machine Learning Techniques." International Journal of Scientific Research in Multidisciplinary Studies 8.1 (2022): 56-62.
APA Style Citation: Murinto, M. Rosyda, (2022). Identification and Classification of Oil Palm Maturity Using Machine Learning Techniques. International Journal of Scientific Research in Multidisciplinary Studies , 8(1), 56-62.
BibTex Style Citation:
@article{Rosyda_2022,
author = {Murinto, M. Rosyda},
title = {Identification and Classification of Oil Palm Maturity Using Machine Learning Techniques},
journal = {International Journal of Scientific Research in Multidisciplinary Studies },
issue_date = {1 2022},
volume = {8},
Issue = {1},
month = {1},
year = {2022},
issn = {2347-2693},
pages = {56-62},
url = {https://www.isroset.org/journal/IJSRMS/full_paper_view.php?paper_id=2687},
publisher = {IJCSE, Indore, INDIA},
}
RIS Style Citation:
TY - JOUR
UR - https://www.isroset.org/journal/IJSRMS/full_paper_view.php?paper_id=2687
TI - Identification and Classification of Oil Palm Maturity Using Machine Learning Techniques
T2 - International Journal of Scientific Research in Multidisciplinary Studies
AU - Murinto, M. Rosyda
PY - 2022
DA - 2022/01/31
PB - IJCSE, Indore, INDIA
SP - 56-62
IS - 1
VL - 8
SN - 2347-2693
ER -
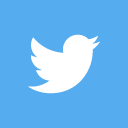
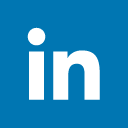
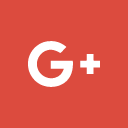
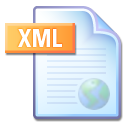
Abstract :
Oil palm is the main plantation crop in Indonesia, oil palm is the most efficient producer of vegetable oil. Oil palm fruit is one of the fruits that has a certain level of maturity in a relatively fast time. The distribution of oil palm fruit in various regions makes it important to identify and classify the maturity of oil palm fruit based on its maturity level. The degree of ripeness of the bunches at harvest is closely related to the oil content contained in the fruit. Accuracy problems are often encountered in research related to image classification. One challenge that arises is finding an appropriate representation of the data so that important structures of the data can be seen easily. One of the processes carried out to get better accuracy is the segmentation process. Through the use of proper segmentation techniques, the desired accuracy will be obtained. One of the techniques used in the segmentation method is to use the swarm optimization technique and its derivatives. In this study, identification and classification will be implemented using particle swarm optimization (PSO) at thresholding image segmentation in order to obtain better segmentation results when compared to the previous method. The classification is based on existing machine learning techniques, namely support vector machine (SVM). the accuracy rate for the classification of palm fruit maturity based on texture using the Support Vector Machine (SVM) method is obtained, which reaches 92.5%. From the accuracy obtained, it can be concluded that the method used to identify and classify in this study is good.
Key-Words / Index Term :
Classification, Particle Swarm Optimization, Support Vector Machine
References :
[1]. R. V. Kulkarni., G. K. Venayagamoorthy, “Bio-inspired algorithms for autonomous deployment and localization of sensor nodes,” IEEE Trans. Syst. Man Cybern. Part C Appl. Rev., 2010.
[2]. Murinto., N.R.D.P Astuti, & M.M Mardhia, “Multilevel Thresholding Hyperspectral Image Segmentation Based on Independent Component and Swarm Optimization Methods”, International Journal of Advances in Intelligent Informatics, 5(1), pp.66-75, 2019.
[3]. B. Sankur, “Survey over image thresholding techniques and quantitative performance evaluation,” J. Electron. Imaging, 2004
[4]. N. Otsu, “Threshold selection method from gray-level histogram,” IEEE Trans Syst Man Cybern, 1979.
[5]. J. Kennedy., R. Eberhart, “Particle swarm optimization,” in IEEE International Conference on Neural Networks - Conference Proceedings, 1995.
[6]. A. Nickabadi, M. M. Ebadzadeh, & R. Safabakhsh, “A novel particle swarm optimization algorithm with adaptive inertia weight,” Appl. Soft Comput. J., 2011.
[7]. V.Jain., A.Jain., A. K. Dubey, “Comparative Study between FA, ACO, and PSO Algorithms for Optimizing Quadratic Assignment Problem,” International Journal of Scientific Research in Computer Science and Engineering, Vol.6, Issue.2, pp.76-81, 2018.
[8]. Murinto., N.R.D.P.Astuti, “Feature reduction using minimum noise fraction and principal component analysis transforms for improving the classification of hyperspectral image”. Asia-Pacific Journal of Science and Technology, 22(1), 2017.
[9]. I.L.Mahargya., G.F. Shidik,"Improvement Support Vector Machine Using Genetic Algorithm in Farmers Term of Trade Prediction at Central Java Indonesia," International Journal on Advanced Science, Engineering and Information Technology, vol. 10, no. 6, pp. 2261-2269, 2020.
[10]. A.Aufar, I.S. Sitanggang., Annisa,"Parameter Optimization of Rainfall-runoff Model GR4J using Particle Swarm Optimization on Planting Calendar," International Journal on Advanced Science, Engineering and Information Technology, vol. 10, no. 6, pp. 2575-2581, 2020.
[11]. Z. Rustam.,D.A.Utami, R.Hidayat., J. Pandelaki & W. A. Nugroho,"Hybrid Preprocessing Method for Support Vector Machine for Classification of Imbalanced Cerebral Infarction Datasets," International Journal on Advanced Science, Engineering and Information Technology, vol. 9, no. 2, pp. 685-691, 2019.
[12]. M. A. Ismail. V. Mezhuyev., I. Darmawan. S.K.Mohd, S. Mohamad &A.O. Ibrahim,"Optimization of Biochemical Systems Production Using Combination of Newton Method and Particle Swarm Optimization," International Journal on Advanced Science, Engineering and Information Technology, vol. 9, no. 3, pp. 753-758, 2019.
[13]. A. Bustamam, D. Sarwinda, B. Abdillah & T.P. Kaloka,"Detecting Lesion Characteristics of Diabetic Retinopathy Using Machine Learning and Computer Vision," International Journal on Advanced Science, Engineering and Information Technology, vol. 10, no. 4, pp. 1367-1373, 2020.
[14]. R. Qayyum, K. Kamal, T. Zafar and S. Mathavan, "Wood defects classification using GLCM based features and PSO trained neural network," 22nd International Conference on Automation and Computing (ICAC), pp. 273-277, 2016.
[15]. B. Wang, Y. Sun, B. Xue & M. Zhang, "Evolving Deep Convolutional Neural Networks by Variable-Length Particle Swarm Optimization for Image Classification," IEEE Congress on Evolutionary Computation (CEC), pp. 1-8, 2018.
[16]. C. Zhang, X. Liu, G. Wang &Z. Cai, "Particle Swarm Optimization Based Deep Learning Architecture Search for Hyperspectral Image Classification," IGARSS 2020 - 2020 IEEE International Geoscience and Remote Sensing Symposium, pp. 509-512, 2020.
[17]. Xing, Z., Jia, H. An improved thermal exchange optimization based GLCM for multi-level image segmentation. Multimed Tools Appl 79, 12007–12040, 2020.
[18]. P. K. Mall, P. K. Singh & D. Yadav, "GLCM Based Feature Extraction and Medical X-RAY Image Classification using Machine Learning Techniques," IEEE Conference on Information and Communication Technology, pp. 1-6, 2019.
[19]. T. M. Alaoui., A.Sbihi , “Texture Classification Based on Co-occurrence Matrix and Neuro-Morphological Approach”, In: Petrosino A. (eds) Image Analysis and Processing – ICIAP 2013. ICIAP 2013.
[20]. P.S. Kumar., V.S. Dharun, “Extraction of Texture Features Using GLCM and Shape Features Using Connected Regions.” International Journal of Engineering and Technology 8.6, pp. 2926–2930. International Journal of Engineering and Technology, 2016.
[21]. Townsend, J. T. “Theoretical Analysis of an Alphabetic Confusion Matrix.” Perception & Psychophysics 9.1 (1971): 40–50.
[22]. S.Jha, S.Gupta., YSharma, : A Review of Feature Selecstion Techniques for Opiniion Mining Systems”, International Journal Of Scientific Research in Multidisiplinary Studies, Vol.6, No.9, pp.65-69, 2020.
You do not have rights to view the full text article.
Please contact administration for subscription to Journal or individual article.
Mail us at support@isroset.org or view contact page for more details.