Full Paper View Go Back
Obsa Gelchu1 , Getachew Gobena2
Section:Research Paper, Product Type: Journal-Paper
Vol.9 ,
Issue.6 , pp.33-41, Jun-2023
Online published on Jun 30, 2023
Copyright © Obsa Gelchu, Getachew Gobena . This is an open access article distributed under the Creative Commons Attribution License, which permits unrestricted use, distribution, and reproduction in any medium, provided the original work is properly cited.
View this paper at Google Scholar | DPI Digital Library
How to Cite this Paper
- IEEE Citation
- MLA Citation
- APA Citation
- BibTex Citation
- RIS Citation
IEEE Style Citation: Obsa Gelchu, Getachew Gobena, “Designing Predictive Model from Clinical Records to Predict Stroke Disease Using Machine Learning Techniques,” International Journal of Scientific Research in Multidisciplinary Studies , Vol.9, Issue.6, pp.33-41, 2023.
MLA Style Citation: Obsa Gelchu, Getachew Gobena "Designing Predictive Model from Clinical Records to Predict Stroke Disease Using Machine Learning Techniques." International Journal of Scientific Research in Multidisciplinary Studies 9.6 (2023): 33-41.
APA Style Citation: Obsa Gelchu, Getachew Gobena, (2023). Designing Predictive Model from Clinical Records to Predict Stroke Disease Using Machine Learning Techniques. International Journal of Scientific Research in Multidisciplinary Studies , 9(6), 33-41.
BibTex Style Citation:
@article{Gelchu_2023,
author = {Obsa Gelchu, Getachew Gobena},
title = {Designing Predictive Model from Clinical Records to Predict Stroke Disease Using Machine Learning Techniques},
journal = {International Journal of Scientific Research in Multidisciplinary Studies },
issue_date = {6 2023},
volume = {9},
Issue = {6},
month = {6},
year = {2023},
issn = {2347-2693},
pages = {33-41},
url = {https://www.isroset.org/journal/IJSRMS/full_paper_view.php?paper_id=3183},
publisher = {IJCSE, Indore, INDIA},
}
RIS Style Citation:
TY - JOUR
UR - https://www.isroset.org/journal/IJSRMS/full_paper_view.php?paper_id=3183
TI - Designing Predictive Model from Clinical Records to Predict Stroke Disease Using Machine Learning Techniques
T2 - International Journal of Scientific Research in Multidisciplinary Studies
AU - Obsa Gelchu, Getachew Gobena
PY - 2023
DA - 2023/06/30
PB - IJCSE, Indore, INDIA
SP - 33-41
IS - 6
VL - 9
SN - 2347-2693
ER -
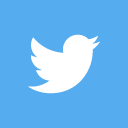
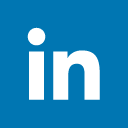
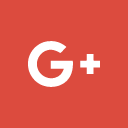
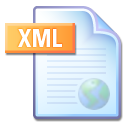
Abstract :
Stroke disease is a cardiovascular disease that when the blood supply to the brain is interrupted, causing a part of the brain to die. This study aims to design and develop a predictive model from clinical records to predict Stroke Disease using machine learning techniques to achieve the proposed objectives we collected datasets from local Hospitals. This study applied five supervised machine learning classifier algorithms namely, Support Vector Machine, Logistic Regression, Random Forest, Decision Tree, and Naïve Bayes along with univariate feature selection. Finally, the results of the study show that RF scored a prediction accuracy of 97.88% with an AUC of 99.43%, and DT scored a prediction accuracy of 96% with an AUC score of 96.%. The other model is the support vector machine which scored a prediction accuracy of 92.6 % with an AUC score of 96.9 %. Logistic regression scored a prediction accuracy of 84.77% with an AUC score of 91 % and the last model is Naïve Bayes which scored a prediction accuracy of 90.43%, with AUC 95.9%. The experiment revealed that Random Forest and Decision Tree classifiers are the most promising for stroke disease prediction in their performance.
Key-Words / Index Term :
Machine Learning, Stroke, Support Vector Machine, Stroke disease prediction, Logistic regression.
References :
[1] I. K. Nti, O. Nyarko-Boateng, J. Aning, G. K. Fosu, H. A. Pokuaa, and F. Kyeremeh, “Early Detection of Stroke for Ensuring Health and Well-Being Based on Categorical Gradient Boosting Machine,” J. ICT Res. Appl., vol. 16, no. 3, pp. 313–332, 2022, doi: 10.5614/itbj.ict.res.appl.2022.16.3.8.
[2] J. I. Cameron, G. Naglie, F. L. Silver, and M. A. M. Gignac, “Stroke family caregivers’ support needs change across the care continuum: A qualitative study using the timing it right framework,” Disabil. Rehabil., vol. 35, no. 4, pp. 315–324, 2013, doi: 10.3109/09638288.2012.691937.
[3] B. S. Isaac, D. R. Bharathi, N. Priyanka, and J. John, “A Study on Management of Stroke in a Tertiary Care Hospital,” vol. 5, no. 1, pp. 26–41, 2019.
[4] O. Ogbonda, “Can Immunotherapy be used to Treat Ischemic Stroke??,” vol. 6, no. 1, pp. 154–166, 2019.
[5] G. Sailasya and G. L. A. Kumari, “Analyzing the Performance of Stroke Prediction using ML Classification Algorithms,” Int. J. Adv. Comput. Sci. Appl., vol. 12, no. 6, pp. 539–545, 2021, doi: 10.14569/IJACSA.2021.0120662.
[6] I. Ibrahim and A. Abdulazeez, “The Role of Machine Learning Algorithms for Diagnosing Diseases,” J. Appl. Sci. Technol. Trends, vol. 2, no. 01, pp. 10–19, 2021, doi: 10.38094/jastt20179.
[7] K. A. MAHESH, N. H. SHASHANK, S. SRIKANTH, and M. A. THEJAS, “Prediction of Stroke Using Machine Learning,” no. June, pp. 1–9, 2020.
[8] A. A. Tareke et al., “Magnitude and Predictors of In-Hospital Stroke Mortality in Ethiopia: A Systematic Review and Meta-Analysis,” Stroke Res. Treat., vol. 2022, pp. 1–13, 2022, doi: 10.1155/2022/7202657.
[9] A. Senbato, “Designing healthcare data analytics framework based on big data approach: in case of stroke disease prediction,” no. October, 2019.
[10] P. Chantamit-O-Pas and M. Goyal, “A case-based reasoning framework for prediction of stroke,” Adv. Intell. Syst. Comput., vol. 625, no. October, pp. 219–227, 2018, doi: 10.1007/978-981-10-5508-9_21.
[11] T. G. Temesgen, B. Teshome, and P. Njogu, “Treatment Outcomes and Associated Factors among Hospitalized Stroke Patients at Shashemene Referral Hospital, Ethiopia,” Stroke Res. Treat., vol. 2018, 2018, doi: 10.1155/2018/8079578.
[12] S. Gupta and S. Raheja, “Stroke Prediction using Machine Learning Methods,” Proc. Conflu. 2022 - 12th Int. Conf. Cloud Comput. Data Sci. Eng., no. 4, pp. 553–558, 2022, doi: 10.1109/Confluence52989.2022.9734197.
[13] Z. H. Gufue et al., “Survival of stroke patients according to hypertension status in Northern Ethiopia: Seven years retrospective cohort study,” Vasc. Health Risk Manag., vol. 16, pp. 389–401, 2020, doi: 10.2147/VHRM.S247667.
[14] T. Papadopoulos, A. Abrahim, D. Sergelidis, and K. Bitchava, “Original article ??????????,” vol. 2, no. January, pp. 119–123, 2011, doi: 10.4314/ajcem.v12i3.
[15] M. S. Singh and P. Choudhary, “Stroke prediction using artificial intelligence,” 2017 8th Ind. Autom. Electromechanical Eng. Conf. IEMECON 2017, pp. 158–161, 2017, doi: 10.1109/IEMECON.2017.8079581.
[16] O. Almadani and R. Alshammari, “Prediction of stroke using data mining classification techniques,” Int. J. Adv. Comput. Sci. Appl., vol. 9, no. 1, pp. 457–460, 2018, doi: 10.14569/IJACSA.2018.090163.
[17] M. U. Emon, M. S. Keya, T. I. Meghla, M. M. Rahman, M. S. Al Mamun, and M. S. Kaiser, “Performance Analysis of Machine Learning Approaches in Stroke Prediction,” Proc. 4th Int. Conf. Electron. Commun. Aerosp. Technol. ICECA 2020, no. January, pp. 1464–1469, 2020, doi: 10.1109/ICECA49313.2020.9297525.
[18] C. S. Nwosu, S. Dev, P. Bhardwaj, B. Veeravalli, and D. John, “Predicting Stroke from Electronic Health Records,” Proc. Annu. Int. Conf. IEEE Eng. Med. Biol. Soc. EMBS, no. July 2021, pp. 5704–5707, 2019, doi: 10.1109/EMBC.2019.8857234.
[19] S. Cheon, J. Kim, and J. Lim, “The use of deep learning to predict stroke patient mortality,” Int. J. Environ. Res. Public Health, vol. 16, no. 11, 2019, doi: 10.3390/ijerph16111876.
[20] S. Zhang, C. Zhang, and Q. Yang, “Data preparation for data mining,” Appl. Artif. Intell., vol. 17, no. 5–6, pp. 375–381, 2003, doi: 10.1080/713827180.
[21] S. B. Kotsiantis and D. Kanellopoulos, “Data preprocessing for supervised leaning,” Int. J. …, vol. 1, no. 2, pp. 1–7, 2006, doi: 10.1080/02331931003692557.
You do not have rights to view the full text article.
Please contact administration for subscription to Journal or individual article.
Mail us at support@isroset.org or view contact page for more details.