Full Paper View Go Back
GreenLeafNet: ML Model for Precised and Efficient Detection of Edible Leaf Diseases
Umesha K.1 , Nandhiniumesh 2
Section:Research Paper, Product Type: Journal-Paper
Vol.9 ,
Issue.9 , pp.7-12, Sep-2023
Online published on Sep 30, 2023
Copyright © Umesha K., Nandhiniumesh . This is an open access article distributed under the Creative Commons Attribution License, which permits unrestricted use, distribution, and reproduction in any medium, provided the original work is properly cited.
View this paper at Google Scholar | DPI Digital Library
How to Cite this Paper
- IEEE Citation
- MLA Citation
- APA Citation
- BibTex Citation
- RIS Citation
IEEE Style Citation: Umesha K., Nandhiniumesh, “GreenLeafNet: ML Model for Precised and Efficient Detection of Edible Leaf Diseases,” International Journal of Scientific Research in Multidisciplinary Studies , Vol.9, Issue.9, pp.7-12, 2023.
MLA Style Citation: Umesha K., Nandhiniumesh "GreenLeafNet: ML Model for Precised and Efficient Detection of Edible Leaf Diseases." International Journal of Scientific Research in Multidisciplinary Studies 9.9 (2023): 7-12.
APA Style Citation: Umesha K., Nandhiniumesh, (2023). GreenLeafNet: ML Model for Precised and Efficient Detection of Edible Leaf Diseases. International Journal of Scientific Research in Multidisciplinary Studies , 9(9), 7-12.
BibTex Style Citation:
@article{K._2023,
author = { Umesha K., Nandhiniumesh},
title = {GreenLeafNet: ML Model for Precised and Efficient Detection of Edible Leaf Diseases},
journal = {International Journal of Scientific Research in Multidisciplinary Studies },
issue_date = {9 2023},
volume = {9},
Issue = {9},
month = {9},
year = {2023},
issn = {2347-2693},
pages = {7-12},
url = {https://www.isroset.org/journal/IJSRMS/full_paper_view.php?paper_id=3255},
publisher = {IJCSE, Indore, INDIA},
}
RIS Style Citation:
TY - JOUR
UR - https://www.isroset.org/journal/IJSRMS/full_paper_view.php?paper_id=3255
TI - GreenLeafNet: ML Model for Precised and Efficient Detection of Edible Leaf Diseases
T2 - International Journal of Scientific Research in Multidisciplinary Studies
AU - Umesha K., Nandhiniumesh
PY - 2023
DA - 2023/09/30
PB - IJCSE, Indore, INDIA
SP - 7-12
IS - 9
VL - 9
SN - 2347-2693
ER -
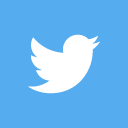
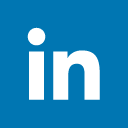
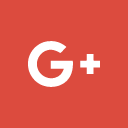
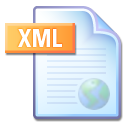
Abstract :
This study uses a ML model, to propose a deep learning strategy for the accurate forecasting of six frequent mint leaf diseases. The proposed model identifies and categorizes different illnesses based on the visual characteristics found in the input photos.The authors have gathered a dataset of photos of green leaves from six categories, to assess the effectiveness of the suggested model. The dataset was divided into testing, training, and validation sets after being preprocessed to ensure consistency in image size and color. On the validation and training collections, the suggested framework was trained and validated, and the testing set was used for the evaluation. All six classes in the evaluation scored highly on accuracy and precision, via a total precision of 90.91% and an adjusted F1-score of 90.90%. Additionally reported were the accuracy, recall, as well as F1-score for each class, all of which demonstrated strong performance. The outcomes show that the suggested CNN model is capable of correctly recognizing and categorizing the six prevalent mint leaf illnesses. This model can be a useful tool for disease detection and avoidance in mint crops, allowing farmers to take appropriate action before the illnesses can cause serious harm. As a result, this study shows the promise of deep learning methods for the precise and effective diagnosis of plant diseases. The suggested CNN system can be further developed to recognize and categorize other plant diseases, advancing precision farming and environmentally friendly crop management techniques.
Key-Words / Index Term :
Class, Crops, Precision, Diseases
References :
[1] Shekhar, Shashi, H. Garg, R. Agrawal, S. Shivani, and B. Sharma, “Hatred and trolling detection transliteration framework using hierarchical LSTM in code-mixed social media text,” in Complex & Intelligent Systems, pp.1–14, 2021.
[2] Trivedi et al., “Early detection and classification of tomato leaf disease using high-performance deep neural network,” Sensors 21, Vol.21, Issue.23, p.7987, 2021.
[3] R. Sharma and V. Kukreja, “Amalgamated convolutional long term network (CLTN) model for Lemon Citrus Canker Disease Multi-classification,” in in International conference on decision aid sciences and applications (DASA), pp.326–329, 2022.
[4] V.Bhardwaj and V. Kukreja, “Effect of pitch enhancement in Punjabi children’s speech recognition system under disparate acoustic conditions,” Appl. Acoust., Vol.177, pp.107918–107931, 2021.
[5] Sathiya, M. S. J. V., and V. Jeyabalaraja, “Plant Disease Classification of Basil and Mint Leaves using Convolutional Neural Networks,” Int. J. Intell. Syst. Appl. Eng. 11, Vol.2, pp.153–163, 2023.
[6] A. Hassan, Md, M. S. Islam, S. B. S. Md Mehedi Hasan, M. T. Habib, and M. S. Uddin, “Medicinal plant recognition from leaf images using deep learning,” Comput. Vis. Mach. Learn. Agric., Vol.2, pp.137–154, 2022.
[7] Lochan, R. Naga, A. S. Tomar, and R. Srinivasan, “Plant detection and classification using fast region-based convolution neural networks,” Artif. Intell. Evol. Comput. Eng. Syst., pp.623–634, 2020.
[8] Sathiya,V.,Josephine and D. Jeyabalaraja, “Identification And Classification Of Diseases In Basil And Mint Plants Using Psorbfnn,” J. Theory. Appl. Inf. Technol. 100, Vol.21, 2022.
[9] Borman, R. Indra, F. Rossi, D. Alamsyah, R. Nuraini, and Y. Jusman, “Classification of Medicinal Wild Plants Using Radial Basis Function Neural Network with Least Mean Square,” in In 2022 2nd International Conference on Electronic and Electrical Engineering and Intelligent System (ICE3IS), pp.141–146, 2022.
[10] Hsieh, Yu-Lun, Y.-C. Chang and W.-Y. Chang, “Hsieh, Yu-Lun, Yung-Chun Chang, and Wei-Yu Chang,” Nutr. 13, Vol.6, 2021.
[11] Oliveira, Americo, S. Pereira, and C. A. Silva, “Augmenting data when training a CNN for retinal vessel segmentation: How to warp?,” 2017 IEEE 5th Port. Meet. Bioeng., pp.1–4, 2017.
[12] Atha, A. Mutiara, and E. Zuliarso, “Deteksi Tanaman Herbal Khusus Untuk Penyakit Kulit Dan Penyakit Rambut Menggunakan Convolutional Neural Network (CNN) Dan Tensor flow,” JUPITER (Journal Penelit. Ilmu dan Tek. Computer) 14, Vol.2-a, pp.1–10, 2022.
[13] Bakkali Yedri, Oussama, M. B. Mohamed Ben Ahmed, and L. El Achaak, “A smart agricultural system to classify agricultural plants and fungus diseases using deep learning,” in In Emerging Trends in ICT for Sustainable Development: The Proceedings of NICE2020 International Conference, pp.229–239, 2021.
[14] Sunil, C. K., C. D. Jaidhar, and N. Patil, “Cardamom plant disease detection approach using EfficientNetV2,” IEEE Access 10, pp.789–804, 2021.
You do not have rights to view the full text article.
Please contact administration for subscription to Journal or individual article.
Mail us at support@isroset.org or view contact page for more details.