Full Paper View Go Back
Estimation of Household Energy Consumption: A Survey
R. Nigam1 , R. Jain2 , A. Sehgal3 , R. Maheshwari4 , P. S. Mehra5
Section:Survey Paper, Product Type: Journal-Paper
Vol.6 ,
Issue.2 , pp.53-63, Dec-2019
Online published on Dec 31, 2019
Copyright © R. Nigam, R. Jain, A. Sehgal, R. Maheshwari, P. S. Mehra . This is an open access article distributed under the Creative Commons Attribution License, which permits unrestricted use, distribution, and reproduction in any medium, provided the original work is properly cited.
View this paper at Google Scholar | DPI Digital Library
How to Cite this Paper
- IEEE Citation
- MLA Citation
- APA Citation
- BibTex Citation
- RIS Citation
IEEE Style Citation: R. Nigam, R. Jain, A. Sehgal, R. Maheshwari, P. S. Mehra, “Estimation of Household Energy Consumption: A Survey,” World Academics Journal of Engineering Sciences, Vol.6, Issue.2, pp.53-63, 2019.
MLA Style Citation: R. Nigam, R. Jain, A. Sehgal, R. Maheshwari, P. S. Mehra "Estimation of Household Energy Consumption: A Survey." World Academics Journal of Engineering Sciences 6.2 (2019): 53-63.
APA Style Citation: R. Nigam, R. Jain, A. Sehgal, R. Maheshwari, P. S. Mehra, (2019). Estimation of Household Energy Consumption: A Survey. World Academics Journal of Engineering Sciences, 6(2), 53-63.
BibTex Style Citation:
@article{Nigam_2019,
author = {R. Nigam, R. Jain, A. Sehgal, R. Maheshwari, P. S. Mehra},
title = {Estimation of Household Energy Consumption: A Survey},
journal = {World Academics Journal of Engineering Sciences},
issue_date = {12 2019},
volume = {6},
Issue = {2},
month = {12},
year = {2019},
issn = {2347-2693},
pages = {53-63},
url = {https://www.isroset.org/journal/WAJES/full_paper_view.php?paper_id=1610},
publisher = {IJCSE, Indore, INDIA},
}
RIS Style Citation:
TY - JOUR
UR - https://www.isroset.org/journal/WAJES/full_paper_view.php?paper_id=1610
TI - Estimation of Household Energy Consumption: A Survey
T2 - World Academics Journal of Engineering Sciences
AU - R. Nigam, R. Jain, A. Sehgal, R. Maheshwari, P. S. Mehra
PY - 2019
DA - 2019/12/31
PB - IJCSE, Indore, INDIA
SP - 53-63
IS - 2
VL - 6
SN - 2347-2693
ER -
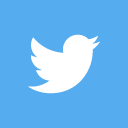
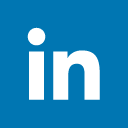
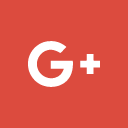
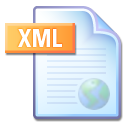
Abstract :
Now-a-days energy consumption has become one of the most studied topics, not just from a climate prospective, but also from technological view of point. The power consumed by millions of household, around the globe, comes majorly from non-renewable sources of energy, which are rapidly depleting. There is an urgent need generating to find ways to effectively consume energy for a sustainable environment. Many researchers have proposed model for Energy Consumption, especially Household sector, to gather different optimized patterns, thus to find solutions to various energy consumption problems. This paper considered various previous works done in the related field, from the year 2010 to 2019, to give a comprehensive study about models applied and dataset used, besides their optimization techniques and results.
Key-Words / Index Term :
Energy Consumption; Household Energy Consumption; Optimization; Machine Learning Techniques; Survey
References :
[1] Zhang W, Robinson C, Guhathakurta S, Garikapati VM, Dilkina B, Brown MA, et al. Estimating residential energy consumption in metropolitan areas: A microsimulation approach. Energy 2018;155:162–73. doi:10.1016/j.energy.2018.04.161.
[2] Bansal A, Rompikuntla SK, Gopinadhan J, Kaur A, Kazi ZA. Energy Consumption Forecasting for Smart Meters 2015.
[3] Gajowniczek K, Ząbkowski T. Electricity forecasting on the individual household level enhanced based on activity patterns. PLoS One 2017;12:e0174098. doi:10.1371/journal.pone.0174098.
[4] Mehra PS, Doja MN, Alam B. Stable period enhancement for zonal (SPEZ)-based clustering in heterogeneous WSN. Smart Innov. Syst. Technol., vol. 79, Springer Science and Business Media Deutschland GmbH; 2018, p. 887–96. doi:10.1007/978-981-10-5828-8_83.
[5] Zhou D, Balandat M, Tomlin C. Residential Demand Response Targeting Using Machine Learning with Observational Data 2016.
[6] Nishida K, Takeda A, Iwata S, Kiho M, Nakayama I. Household energy consumption prediction by feature selection of lifestyle data. 2017 IEEE Int. Conf. Smart Grid Commun. SmartGridComm 2017, vol. 2018- Janua, Institute of Electrical and Electronics Engineers Inc.; 2018, p. 235–40. doi:10.1109/SmartGridComm.2017.8340676.
[7] Ziel F. Forecasting Electricity Spot Prices Using Lasso: On Capturing the Autoregressive Intraday Structure. IEEE Trans Power Syst 2016;31:4977–87. doi:10.1109/TPWRS.2016.2521545.
[8] Mirowski P, Chen S, Kam Ho T, Yu CN. Demand forecasting in smart grids. Bell Labs Tech J 2014;18:135–58. doi:10.1002/bltj.21650.
[9] Tureczek A, Nielsen P, Madsen H. Electricity Consumption Clustering Using Smart Meter Data. Energies 2018;11:859. doi:10.3390/en11040859.
[10] Zhou D, Balandat M, Tomlin C. A Bayesian Perspective on Residential Demand Response Using Smart Meter Data 2016.
[11] Tureczek AM, Nielsen PS. Structured Literature Review of Electricity Consumption Classification Using Smart Meter Data. Citation 2017;10. doi:10.3390/en10050584.
[12] McLoughlin F, Duffy A, Conlon M. A clustering approach to domestic electricity load profile characterisation using smart metering data. Appl Energy 2015;141:190–9. doi:10.1016/j.apenergy.2014.12.039.
[13] Van Dam SS, Bakker CA, Van Hal JDM. Home energy monitors: Impact over the medium-term. Build Res Inf 2010;38:458–69. doi:10.1080/09613218.2010.494832.
[14] Cheung LTO, Chow ASY, Fok L, Yu KM, Chou KL. The effect of self-determined motivation on household energy consumption behaviour in a metropolitan area in southern China. Energy Effic 2017;10:549–61. doi:10.1007/s12053-016-9472-5.
[15] Anvari-Moghaddam A, Monsef H, Rahimi-Kian A. Optimal smart home energy management considering energy saving and a comfortable lifestyle. IEEE Trans Smart Grid 2015;6:324–32. doi:10.1109/TSG.2014.2349352.
[16] Liu Y, Yuen C, Yu R, Zhang Y, Xie S. Queuing-Based Energy Consumption Management for Heterogeneous Residential Demands in Smart Grid. IEEE Trans Smart Grid 2016;7:1650–9. doi:10.1109/TSG.2015.2432571.
[17] Xue J, Ye J, Wang D, Yang B, Zhai J, Liu H. Household energy efficiency management and optimization with energy storage. 2016 IEEE 2nd Annu. South. Power Electron. Conf. SPEC 2016, Institute of Electrical and Electronics Engineers Inc.; 2016. doi:10.1109/SPEC.2016.7846052.
[18] Cerquitelli T, Chicco G, Di Corso E, Ventura F, Montesano G, Del Pizzo A, et al. Discovering electricity consumption over time for residential consumers through cluster analysis. 2018 14th Int. Conf. Dev. Appl. Syst. DAS 2018 - Proc., Institute of Electrical and Electronics Engineers Inc.; 2018, p. 164–9. doi:10.1109/DAAS.2018.8396090.
[19] Al-Otaibi R, Jin N, Wilcox T, Flach P. Feature Construction and Calibration for Clustering Daily Load Curves from Smart-Meter Data. IEEE Trans Ind Informatics 2016;12:645–54. doi:10.1109/TII.2016.2528819.
[20] Elattar EE, Goulermas J, Wu QH. Electric load forecasting based on locally weighted support vector regression. IEEE Trans Syst Man Cybern Part C Appl Rev 2010;40:438–47. doi:10.1109/TSMCC.2010.2040176.
[21] Eseye AT, Lehtonen M, Tukia T, Uimonen S, John Millar R. Machine learning based integrated feature selection approach for improved electricity demand forecasting in decentralized energy systems. IEEE Access 2019;7:91463–75. doi:10.1109/ACCESS.2019.2924685.
[22] Yoo SG, Myriam HÁ. Predicting residential electricity consumption using neural networks: A case study. J. Phys. Conf. Ser., vol. 1072, Institute of Physics Publishing; 2018. doi:10.1088/1742-6596/1072/1/012005.
[23] Edwards RE, New J, Parker LE. Predicting future hourly residential electrical consumption: A machine learning case study. Energy Build 2012;49:591–603. doi:10.1016/j.enbuild.2012.03.010.
[24] Ozoh P, Abd-Rahman S, Labadin J. Predicting electricity consumption: A comparative analysis of the accuracy of various computational techniques. 2015 9th Int. Conf. IT Asia Transform. Big Data into Knowledge, CITA 2015 - Proc., Institute of Electrical and Electronics Engineers Inc.; 2015. doi:10.1109/CITA.2015.7349819.
[25] Sun C, Sun F, Moura SJ. Nonlinear predictive energy management of residential buildings with photovoltaics & batteries. J Power Sources 2016;325:723–31. doi:10.1016/j.jpowsour.2016.06.076.
[26] Li K, Su H, Chu J. Forecasting building energy consumption using neural networks and hybrid neuro-fuzzy system: A comparative study. Energy Build 2011;43:2893–9. doi:10.1016/j.enbuild.2011.07.010.
[27] Agnetis A, De Pascale G, Detti P, Vicino A. Load scheduling for household energy consumption optimization. IEEE Trans Smart Grid 2013;4:2364–73. doi:10.1109/TSG.2013.2254506.
[28] Kwac J, Flora J, Rajagopal R. Household energy consumption segmentation using hourly data. IEEE Trans Smart Grid 2014;5:420–30. doi:10.1109/TSG.2013.2278477.
[29] Zhang XM, Grolinger K, Capretz MAM, Seewald L. Forecasting Residential Energy Consumption: Single Household Perspective. Proc. - 17th IEEE Int. Conf. Mach. Learn. Appl. ICMLA 2018, Institute of Electrical and Electronics Engineers Inc.; 2019, p. 110–7. doi:10.1109/ICMLA.2018.00024.
[30] Sharma VS, Pamnani RN. International Journal of Computer Science and Mobile Computing EFFECTIVE PREDICTION OF ELECTRICITY CONSUMPTION BASED ON EFFICIENT ANALYSIS OF HOUSEHOLD CHARACTERISTICS. vol. 6. 2017.
[31] Iwafune Y, Yagita Y, Ogimoto K. Estimation of appliance electricity consumption by monitoring currents on residential distribution boards. 2010 Int. Conf. Power Syst. Technol. Technol. Innov. Mak. Power Grid Smarter, POWERCON2010, 2010. doi:10.1109/POWERCON.2010.5666076.
[32] Ghofrani M, Hassanzadeh M, Etezadi-Amoli M, Fadali MS. Smart meter based short-term load forecasting for residential customers. NAPS 2011 - 43rd North Am. Power Symp., 2011. doi:10.1109/NAPS.2011.6025124.
[33] Li Y, Ng BL, Trayer M, Liu L. Automated residential demand response: Algorithmic implications of pricing models. IEEE Trans Smart Grid 2012;3:1712–21. doi:10.1109/TSG.2012.2218262.
[34] Mehra PS, Doja MN, Alam B. Enhanced Clustering Algorithm based on Fuzzy Logic (E-CAFL) for WSN. Scalable Comput Pract Exp 2019;20:41–54. doi:10.12694/scpe.v20i1.1443.
[35] Cai H, Shen S, Lin Q, Li X, Xiao H. Predicting the Energy Consumption of Residential Buildings for Regional Electricity Supply-Side and Demand-Side Management. IEEE Access 2019;7:30386–97. doi:10.1109/ACCESS.2019.2901257.
[36] Bhattacharjee S, Reichard G. Socio-economic factors affecting individual household energy consumption: A systematic review. ASME 2011 5th Int. Conf. Energy Sustain. ES 2011, 2011, p. 891–901. doi:10.1115/ES2011-54615.
[37] Subbiah R, Pal A, Nordberg EK, Marathe A, Marathe M V. Energy Demand Model for Residential Sector: A First Principles Approach. IEEE Trans Sustain Energy 2017;8:1215–24. doi:10.1109/TSTE.2017.2669990.
[38] Jain RK, Smith KM, Culligan PJ, Taylor JE. Forecasting energy consumption of multi-family residential buildings using support vector regression: Investigating the impact of temporal and spatial monitoring granularity on performance accuracy. Appl Energy 2014;123:168–78. doi:10.1016/j.apenergy.2014.02.057.
[39] Nagpal D, Srivastava R, Mehrotra D, Anuranjana. Feature selection approach for reducing the power consumption for a greener environment. 2nd Int. Conf. Telecommun. Networks, TEL-NET 2017, vol. 2018- January, Institute of Electrical and Electronics Engineers Inc.; 2018, p. 1–5. doi:10.1109/TEL-NET.2017.8343509.
[40] Amara F, Agbossou K, Dube Y, Kelouwani S, Cardenas A. Estimation of temperature correlation with household electricity demand for forecasting application. IECON Proc. (Industrial Electron. Conf., IEEE Computer Society; 2016, p. 3960–5. doi:10.1109/IECON.2016.7793935.
[41] Pirbazari AM, Chakravorty A, Rong C. Evaluating Feature Selection Methods for Short-Term Load Forecasting. 2019 IEEE Int. Conf. Big Data Smart Comput. BigComp 2019 - Proc., Institute of Electrical and Electronics Engineers Inc.; 2019. doi:10.1109/BIGCOMP.2019.8679188.
[42] Masoodian M, Buchwald I, Luz S, André E. Temporal visualization of energy consumption loads using time-tone. Proc. - 2017 21st Int. Conf. Inf. Vis. iV 2017, Institute of Electrical and Electronics Engineers Inc.; 2017, p. 146–51. doi:10.1109/iV.2017.13
You do not have rights to view the full text article.
Please contact administration for subscription to Journal or individual article.
Mail us at support@isroset.org or view contact page for more details.