Main Menu
Journals Contents
Information
Download
Publication Certificate
Full Paper View Go Back
Integrated Intelligent Framework for Sensor Data Analysis
Kalyani S.1 , Venkat Rao K.2 , A. Mary Sowjanya3
Section:Research Paper, Product Type: Journal-Paper
Vol.7 ,
Issue.3 , pp.52-59, Sep-2020
Online published on Sep 30, 2020
Copyright © Kalyani S., Venkat Rao K., A. Mary Sowjanya . This is an open access article distributed under the Creative Commons Attribution License, which permits unrestricted use, distribution, and reproduction in any medium, provided the original work is properly cited.
View this paper at Google Scholar | DPI Digital Library
How to Cite this Paper
- IEEE Citation
- MLA Citation
- APA Citation
- BibTex Citation
- RIS Citation
IEEE Citation
IEEE Style Citation: Kalyani S., Venkat Rao K., A. Mary Sowjanya, “Integrated Intelligent Framework for Sensor Data Analysis,” World Academics Journal of Engineering Sciences, Vol.7, Issue.3, pp.52-59, 2020.
MLA Citation
MLA Style Citation: Kalyani S., Venkat Rao K., A. Mary Sowjanya "Integrated Intelligent Framework for Sensor Data Analysis." World Academics Journal of Engineering Sciences 7.3 (2020): 52-59.
APA Citation
APA Style Citation: Kalyani S., Venkat Rao K., A. Mary Sowjanya, (2020). Integrated Intelligent Framework for Sensor Data Analysis. World Academics Journal of Engineering Sciences, 7(3), 52-59.
BibTex Citation
BibTex Style Citation:
@article{S._2020,
author = {Kalyani S., Venkat Rao K., A. Mary Sowjanya},
title = {Integrated Intelligent Framework for Sensor Data Analysis},
journal = {World Academics Journal of Engineering Sciences},
issue_date = {9 2020},
volume = {7},
Issue = {3},
month = {9},
year = {2020},
issn = {2347-2693},
pages = {52-59},
url = {https://www.isroset.org/journal/WAJES/full_paper_view.php?paper_id=2083},
publisher = {IJCSE, Indore, INDIA},
}
RIS Citation
RIS Style Citation:
TY - JOUR
UR - https://www.isroset.org/journal/WAJES/full_paper_view.php?paper_id=2083
TI - Integrated Intelligent Framework for Sensor Data Analysis
T2 - World Academics Journal of Engineering Sciences
AU - Kalyani S., Venkat Rao K., A. Mary Sowjanya
PY - 2020
DA - 2020/09/30
PB - IJCSE, Indore, INDIA
SP - 52-59
IS - 3
VL - 7
SN - 2347-2693
ER -
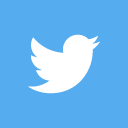
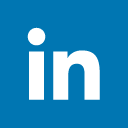
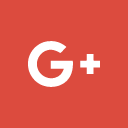
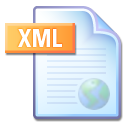
Abstract :
Increased smart devices in various industries is creating numerous sensors in each of the equipment prompting the need for methods and models for sensor data. Current research proposes a systematic approach to analyze the data generated from sensors attached to industrial equipment. Time to failure data is generated using the end of life information of assets available from the historical data. The methodology involves data cleaning, preprocessing, basics statistics, outlier, and anomaly detection. Present study focusses on prediction of RUL by using various Machine Learning models like Regression, Polynomial Regression, Random Forest, Decision Tree, XG Boost. In each of the model for RUL prediction RMSE, MAE are compared. Outcome of the RUL prediction is useful for decision maker to drive the business decision, hence Binary & Multi class classification is performed to solve business challenges. Labels for Binary & Multi class classification can be generated from the asset operation needs and maintenance manuals. Business case analysis includes the cost of maintenance and cost of non-maintaining a particular asset. Current research is aimed at integrating the machine intelligence and business intelligence so that the industrial operations optimized both in resource and profit. Multi Class classification is developed to find the classes based on multiple time periods for asset maintenance.
Key-Words / Index Term :
IoT, Life Estimation, RUL, Sensor Data
References :
[1] B. D. Minor, J. R. Doppa and D. J.Cook, “Learning Activity Predictors from Sensor Data: Algorithms, Evaluation, and Applications,” IEEE transactions on knowledge and data engineering, vol. 29, no. 12, pp.2744–2757, 2017.
[2] Ravi Katukam, B. Prajna, “Engineering Optimization using Artificial Neural Network,” International Journal of Innovations in Engineering & Technology (IJIET), , vol. 4, no. 3, pp. 63–72, 2014.
[3] M. Alexandra, P. Marko ,P. Maria,F. Carolina and M Dunja, “Using Machine Learning on Sensor Data”, Journal of Computing and Information Technology vol. 18, no. 4, pp. 341–347, 2010.
[4] H.A. Taha, A.H. Sakr, Y. Soumaya, “Aircraft Engine Remaining Useful Life Prediction Framework for Industry 4.0”, in Proc. ICIEOMT, Canada, pp. 23-25, 2019.
[5] C. Okoh, R. Roy, J. Mehnen, L. Redding “Overview of Remaining Useful Life Prediction Techniques in Through-Life Engineering Services” in Proc. CIRP 16 pp.158 – 163, 2014.
[6] A. L. Ellefsen, B. Emil, A. Vilmar, S. Ushakovb, H. Zhanga “Remaining useful life predictions for turbofan engine degradation using semi-supervised deep architecture” Reliability Engineering and System safety 183, pp.240-251, 2019.
[7] R R. Zhou, N. Serban N. Gebraeel, “Degradation modeling applied to residual lifetime prediction using functional data analysis”, The Annals of Applied Statistics, Vol. 5, no. 2B, pp. 1586–1610, 2011
[8] H. Hanachi, C. Mechefske, J. Liu, A. Banerjee, Y. Chen, “Performance-based gas turbine health monitoring, diagnostics, and prognostics: A survey” IEEE Trans. Reliab. Vol. 67,no. 3, pp.1340–1363 . 2018.
[9] S.K. Singh, S.Kumar, J.P Dwivedi, “A novel soft computing method for engine RUL prediction”, Multimed. Tools Appl Vol. 78, pp. 4065–4087, 2019.
[10] S. Al-Dahidi, F. Di Maio, P. Baraldi, E. Zio, “Remaining useful life estimation in heterogeneous fleets working under variable operating conditions,” Reliab. Eng. Syst. Saf., Vol 156, pp. 109–124. 2016.
[11] A. Saxena, K. Goebel, D. Simon, N. Eklund, “Damage Propagation for Aircraft Engine Run-To-Failure Simulation,” in Proc. I C PH M, Denver, CO, USA, PP. 6–9 2008.
[12] B. Zhang, L.J. Zhang, J.W. Xu, “Degradation feature selection for remaining useful life prediction of rolling element bearings,” Qual. Reliab. Eng. Int., no.32, pp. 547–554. 2016.
[13] M. Kim, C. Song, K. Liu, “ A generic health index approach for multisensor degradation modeling and sensor selection,” IEEE Trans. Autom. Sci. Eng., no.16, pp.1426–1437, 2019.
[14] P.F. Wang, B.D. Youn, C. Hu, “ A generic probabilistic framework for structural health prognostics and uncertainty management,” Mech. Syst. Signal Process., no. 28, pp. 622–637. 2012.
[15] C.Y. Song, K.B Liu, “ ” IISE Trans. no. 50, pp. 853–867 ,2018.
[16] Liu, K.B.; Huang, S. IEEE Trans. Autom. Sci. Eng., no. 13, pp. 344–354, 2016.
[17] K.T.Huynh, Langeron A, Grall, Degradation Modeling and RUL Estimation of Deteriorating Systems in S-Plane, IFAC,501-1(2017), 12249–12254
[18] S. Sankararaman, M. Daigle, A. Saxena and K. Goebel, "Analytical algorithms to quantify the uncertainty in remaining useful life prediction," 2013 IEEE Aerospace Conference, Big Sky, MT, 2013, pp. 1-11, doi: 10.1109/AERO.2013.6496971.
[19] Nathan Bolander , Hai Qiu , Neil Eklund , Ed Hindle , Taylor Rosenfeld3 Physics-based Remaining Useful Life Prediction for Aircraft Engine Bearing Prognosis, Annual Conference of the Prognostics and Health Management Society, 2009
[20] Jian Ma, Hua Su, Wan-lin Zhao,Bin Liu, Predicting the Remaining Useful Life of an Aircraft Engine Using a Stacked Sparse Autoencoder with Multilayer Self-Learning, Volume 2018
[21] Hu, Y., Liu, S., Lu, H. et al. Remaining Useful Life Model and Assessment of Mechanical Products: A Brief Review and a Note on the State Space Model Method. Chin. J. Mech. Eng. 32, 15, 2019.
[22] Yuhuang Zheng, Predicting Remaining Useful Life Based on Hilbert–Huang Entropy with Degradation Model, Volume 2019
[23] JunZhua , NanChena, ChangqingShen A new data-driven transferable remaining useful life prediction approach for bearing under different working conditions. Mechanical Systems and Signal Processing Volume 139, May 2020, 106602
[24] Zhang X, Xiao L, Kang J. Degradation prediction model based on a neural network with dynamic windows. Sensors (Basel). 2015;15(3):6996-7015. Published 2015 Mar 23. doi:10.3390/s150306996
[25] Narendhar Gugulothu, Vishnu TV, Pankaj Malhotra, Lovekesh Vig, Puneet Agarwal, Gautam, Predicting Remaining Useful Life using Time Series Embeddings based on Recurrent Neural Networks, tcs research
[26] Ahmed Mosallam, Kamal Medjaher, Nourredine Zerhouni ,Bayesian Approach for Remaining Useful Life Prediction, Chemical Engineering Transactions, Vol 13, 2013
[27] Pranav Kumar, Ravi Katukam, Simplex Tool for Engineering Optimization, IJERT, VOLUME 02, ISSUE 08, AUGUST 2013.
[28] C. Premalatha, Automatic Smart Irrigation System Using IOT, International Journal of Scientific Research in Computer Science and Engineering Vol.7, Issue.1, pp.1-5, February 2019.
You do not have rights to view the full text article.
Please contact administration for subscription to Journal or individual article.
Mail us at support@isroset.org or view contact page for more details.