Full Paper View Go Back
An Analytical Study on Music Listener Emotion through Logistic Regression
Md. Anzir Hossain Rafath1 , Fatema Tuz Zohura Mim2 , Md. Shafiur Rahman3
Section:Research Paper, Product Type: Journal-Paper
Vol.8 ,
Issue.3 , pp.15-20, Sep-2021
Online published on Sep 30, 2021
Copyright © Md. Anzir Hossain Rafath, Fatema Tuz Zohura Mim, Md. Shafiur Rahman . This is an open access article distributed under the Creative Commons Attribution License, which permits unrestricted use, distribution, and reproduction in any medium, provided the original work is properly cited.
View this paper at Google Scholar | DPI Digital Library
How to Cite this Paper
- IEEE Citation
- MLA Citation
- APA Citation
- BibTex Citation
- RIS Citation
IEEE Style Citation: Md. Anzir Hossain Rafath, Fatema Tuz Zohura Mim, Md. Shafiur Rahman, “An Analytical Study on Music Listener Emotion through Logistic Regression,” World Academics Journal of Engineering Sciences, Vol.8, Issue.3, pp.15-20, 2021.
MLA Style Citation: Md. Anzir Hossain Rafath, Fatema Tuz Zohura Mim, Md. Shafiur Rahman "An Analytical Study on Music Listener Emotion through Logistic Regression." World Academics Journal of Engineering Sciences 8.3 (2021): 15-20.
APA Style Citation: Md. Anzir Hossain Rafath, Fatema Tuz Zohura Mim, Md. Shafiur Rahman, (2021). An Analytical Study on Music Listener Emotion through Logistic Regression. World Academics Journal of Engineering Sciences, 8(3), 15-20.
BibTex Style Citation:
@article{Rafath_2021,
author = {Md. Anzir Hossain Rafath, Fatema Tuz Zohura Mim, Md. Shafiur Rahman},
title = {An Analytical Study on Music Listener Emotion through Logistic Regression},
journal = {World Academics Journal of Engineering Sciences},
issue_date = {9 2021},
volume = {8},
Issue = {3},
month = {9},
year = {2021},
issn = {2347-2693},
pages = {15-20},
url = {https://www.isroset.org/journal/WAJES/full_paper_view.php?paper_id=2532},
publisher = {IJCSE, Indore, INDIA},
}
RIS Style Citation:
TY - JOUR
UR - https://www.isroset.org/journal/WAJES/full_paper_view.php?paper_id=2532
TI - An Analytical Study on Music Listener Emotion through Logistic Regression
T2 - World Academics Journal of Engineering Sciences
AU - Md. Anzir Hossain Rafath, Fatema Tuz Zohura Mim, Md. Shafiur Rahman
PY - 2021
DA - 2021/09/30
PB - IJCSE, Indore, INDIA
SP - 15-20
IS - 3
VL - 8
SN - 2347-2693
ER -
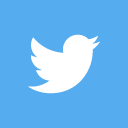
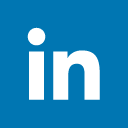
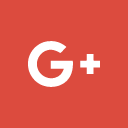
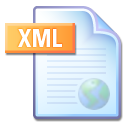
Abstract :
Now-a-days music listeners face immense obstacles when trying to find suitable music for a specific context. This is what actually motivated us to find a suitable solution on it. Here we presented a music classification system that provides the listener an opportunity to browse their music by mood. Mood classification while listening to music is an emerging domain of music information retrieval. This paper investigates the challenging issue of recognizing emotions while listening to music. Specifically, we formulate the music types as a regression problem to predict the human emotional state. No categorical taxonomy is used that’s why the regression approach is free of the ambiguity inherent to conventional categorical approaches. This will help to build an intuitive and contextually aware playlist generation tool. Talking of play list tool we have come to the point that most people are not sure that if their mood has been changed or not while listening to their favorite music, people tend to listen to emotional music when they deal with sorrow or disgust. In the time fear and anger they don’t listen any music. In the contrast they listen to music while having good feelings. We have achieved 78% of accuracy by taking the regression approach for the research.
Key-Words / Index Term :
Data Mining, Machine learning, Regression approach, Emotion prediction, Music analysis
References :
[1] K. Hevner, “Experimental studies of the elements of expression in music,” The American Journal of Psychology, vol. 48, no. 2, pp. 246{268, 1936.
[2] J. A. Russell, “A circumplex model of affect.,” Journal of personality and social psychology, vol. 39, no. 6, p. 1161, 1980.
[3] R. E. Thayer, The biopsychology of mood and arousal. Oxford University Press, 1990.
[4] E. Schubert, “Update of the hevner adjective checklist,” Perceptual and motor skills, vol. 96, no. 3 suppl, pp. 1117-1122, 2003.
[5] C. M. Whissell, “The dictionary of affect in language,” in The measurement of emotions, Elsevier, 1989, pp. 113{131.
[6] D. Baum, “Emomusic-classifying music according to emotion,” in Proceedings of the 7th Workshop on Data Analysis (WDA2006), Citeseer, 2006.
[7] K. C. Dewi and A. Harjoko, “Kid`s song classification based on mood parameters using k-nearest neighbor classification method and self organizing map,” in 2010 International Conference on Distributed Frameworks for Multimedia Applications, IEEE, pp. 1-5, 2010.
[8] Y.H. Yang, Y.C. Lin, Y.F. Su, and H. H. Chen, “A regression approach to music emotion recognition," IEEE Transactions on audio, speech, and language processing, vol. 16, no. 2, pp. 448-457, 2008.
[9] T. Li and M. Ogihara, “Content-based music similarity search and emotion detection,” in 2004 IEEE International Conference on Acoustics, Speech, and Signal Processing, IEEE, vol. 5, pp. V-705, 2004.
[10] D. Cabrera et al., “Psysound: A computer program for psychoacoustical analysis,” in Proceedings of the Australian Acoustical Society Conference, vol. 24, pp. 47-54, 1999.
[11] G. Tzanetakis and P. Cook, “Musical genre classification of audio signals,” IEEE Transactions on speech and audio processing, vol. 10, no. 5, pp. 293-302, 2002.
[12] A. Sen and M. Srivastava, Regression analysis: theory, methods, and applications. Springer Science & Business Media, 2012.
[13] A. J. Smola and B. Schölkopf, “A tutorial on support vector regression,” Statistics and computing, vol. 14, no. 3, pp. 199-222, 2004.
[14] D. P. Solomatine and D. L. Shrestha, “Adaboost. rt: A boosting algorithm for regression problems,” in 2004 IEEE International Joint Conference on Neural Networks (IEEE Cat. No. 04CH37541), IEEE, vol. 2, pp. 1163-1168, 2004.
[15] E. Schubert, Measurement and time series analysis of emotion in music. University of New South Wales Sydney, vol. 1, 1999.
[16] A. Hanjalic and L.-Q. Xu, “Affective video content representation and modeling,” IEEE transactions on multimedia, vol. 7, no. 1, pp. 143-154, 2005.
[17] Y.H. Yang, C.C. Liu, and H. H. Chen, “Music emotion classification: A fuzzy approach,” in Proceedings of the 14th ACM international conference on Multimedia, pp. 81-84, 2006.
[18] M. D. Korhonen, D. A. Clausi, and M. E. Jernigan, “Modeling emotional content of music using system identification,” IEEE Transactions on Systems, Man, and Cybernetics, Part B (Cybernetics), vol. 36, no. 3, pp. 588-599, 2006.
[19] B.j. Han, S. Rho, R. B. Dannenberg, and E. Hwang, “Smers: Music emotion recognition using support vector regression.,” in ISMIR, Citeseer, pp. 651-656, 2009.
[20] P. N. Juslin and J. A. Sloboda, Music and emotion: Theory and research. Oxford University Press, 2001.
[21] R. Sridharan, “Gaussian mixture models and the em algorithm,” Available in: http://people. csail. mit. edu/rameshvs/content/gmm-em. pdf, 2014.
[22] C.C. Chang and C.J. Lin, “Libsvm: A library for support vector machines,” ACM transactions on intelligent systems and technology (TIST), vol. 2, no. 3, pp. 1-27, 2011.
[23] Y. E. Kim, E. M. Schmidt, R. Migneco, B. G. Morton, P. Richardson, J. Scott, J. A. Speck, and D. Turnbull, “Music emotion recognition: A state of the art review,” in Proc. ismir, vol. 86, pp. 937-952, 2010.
[24] A. Mehrabian and J. A. Russell, An approach to environmental psychology. the MIT Press, 1974.
[25] M. M. Bradley and P. J. Lang, “Affective norms for English words (anew): Instruction manual and affective ratings,” Technical report C-1, the center for research in psychophysiology., Tech. Rep., 1999.
[26] Y. Hu, X. Chen, and D. Yang, “Lyric-based song emotion detection with affective lexicon and fuzzy clustering method.,” in ISMIR, pp. 123-128, 2009.
[27] L. Mion and G. De Poli, “Score-independent audio features for description of music expression,” IEEE Transactions on Audio, Speech, and Language Processing, vol. 16, no. 2, pp. 458-466, 2008.
[28] D. Yang and W.-S. Lee, “Disambiguating music emotion using software agents.,” in ISMIR, vol. 4, pp. 218-223, 2004.
[29] K. Bischo, C. S. Firan, R. Paiu, W. Nejdl, C. Laurier, and M. Sordo, “Music mood and theme classi_cation-a hybrid approach.,” in ISMIR, pp. 657-662, 2009.
[30] M. Soleymani, M. N. Caro, E. M. Schmidt, and Y.-H. Yang, “The mediaeval 2013 brave new task: Emotion in music.,” in MediaEval, Citeseer, 2013.
[31] F. Zhang, H. Meng, and M. Li, “Emotion extraction and recognition from music,” in 2016 12th International Conference on Natural Computation, Fuzzy Systems and Knowledge Discovery (ICNC-FSKD), IEEE, pp. 1728-1733. 2016.
[32] A. Aljanaki, F. Wiering, and R. C. Veltkamp, “Mirutrecht participation in mediaeval 2013: Emotion in music task.,” in MediaEval, Citeseer, 2013.
[33] J. Brownlee, Logistic Regression for Machine Learning, en-US, Mar.2016.[Online].Available:https://machinelearningmastery.com/logistic-regression-for-machine-learning
You do not have rights to view the full text article.
Please contact administration for subscription to Journal or individual article.
Mail us at support@isroset.org or view contact page for more details.