Full Paper View Go Back
Parametric and Nonparametric Regression Modeling for Oil Sectors Stock Prices Time Series Data
Manikandan B.1 , Rajarathinam A.2
Section:Research Paper, Product Type: Isroset-Journal
Vol.6 ,
Issue.3 , pp.49-54, Jun-2019
CrossRef-DOI: https://doi.org/10.26438/ijsrmss/v6i3.4954
Online published on Jun 30, 2019
Copyright © Manikandan B. , Rajarathinam A. . This is an open access article distributed under the Creative Commons Attribution License, which permits unrestricted use, distribution, and reproduction in any medium, provided the original work is properly cited.
View this paper at Google Scholar | DPI Digital Library
How to Cite this Paper
- IEEE Citation
- MLA Citation
- APA Citation
- BibTex Citation
- RIS Citation
IEEE Style Citation: Manikandan B. , Rajarathinam A., “Parametric and Nonparametric Regression Modeling for Oil Sectors Stock Prices Time Series Data,” International Journal of Scientific Research in Mathematical and Statistical Sciences, Vol.6, Issue.3, pp.49-54, 2019.
MLA Style Citation: Manikandan B. , Rajarathinam A. "Parametric and Nonparametric Regression Modeling for Oil Sectors Stock Prices Time Series Data." International Journal of Scientific Research in Mathematical and Statistical Sciences 6.3 (2019): 49-54.
APA Style Citation: Manikandan B. , Rajarathinam A., (2019). Parametric and Nonparametric Regression Modeling for Oil Sectors Stock Prices Time Series Data. International Journal of Scientific Research in Mathematical and Statistical Sciences, 6(3), 49-54.
BibTex Style Citation:
@article{B._2019,
author = {Manikandan B. , Rajarathinam A.},
title = {Parametric and Nonparametric Regression Modeling for Oil Sectors Stock Prices Time Series Data},
journal = {International Journal of Scientific Research in Mathematical and Statistical Sciences},
issue_date = {6 2019},
volume = {6},
Issue = {3},
month = {6},
year = {2019},
issn = {2347-2693},
pages = {49-54},
url = {https://www.isroset.org/journal/IJSRMSS/full_paper_view.php?paper_id=1324},
doi = {https://doi.org/10.26438/ijcse/v6i3.4954}
publisher = {IJCSE, Indore, INDIA},
}
RIS Style Citation:
TY - JOUR
DO = {https://doi.org/10.26438/ijcse/v6i3.4954}
UR - https://www.isroset.org/journal/IJSRMSS/full_paper_view.php?paper_id=1324
TI - Parametric and Nonparametric Regression Modeling for Oil Sectors Stock Prices Time Series Data
T2 - International Journal of Scientific Research in Mathematical and Statistical Sciences
AU - Manikandan B. , Rajarathinam A.
PY - 2019
DA - 2019/06/30
PB - IJCSE, Indore, INDIA
SP - 49-54
IS - 3
VL - 6
SN - 2347-2693
ER -
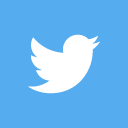
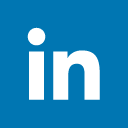
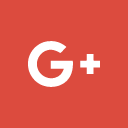
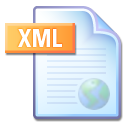
Abstract :
The present investigation is carried out to study the trends in different oil sectors viz., Indian Oil Corporation, Bharat Petroleum and Hindustan Petroleum, stock prices time series data based on parametric and nonparametric regression models. In parametric models different linear models are employed. The statistically most suited parametric models are selected on the basis of highest adjusted R2, significant regression co-efficient and co-efficient of determination (R2), assumptions of normality and independence of residuals. The best fitted model is selected based on the model performance measures such as, Root Mean Square Error, Mean Absolute Error, and Mean Absolute Percentage Error. Nonparametric estimates of underlying growth functions are computed at each and every time points. Relative growth rates are estimated based on the best fitted trend function. In this study none of the parametric models are found suitable to study the trend. Nonparametric regression is found an appropriate tool to study the trends. Decreases in trends with no significant jump have been observed. The mean growth rate is high for Bharat Petroleum Corporation Limited in comparison to that of Hindustan Petroleum Corporation Limited and Indian Oil Corporation.
Key-Words / Index Term :
Parametric model, Nonparametric, Kernel Smoothers, Band width, Cross–validation
References :
[1] D. C. Montgomery, E. A. Peck and G. G. Vining, “Introduction to Linear Regression Analysis”, john Wiley & sons, Inc, 2003.
[2] G. E. P. Box, and D. A. Pierce, “Distribution of Residual Autocorrelations in Autoregressive-Integrated Moving Average Time series Models”. Journal of the American Statistical Association. Vol. 65, Issue.332, pp. 1509-1526, 1970.
[3] G. E. P. Box, and G. M. Jenkins, “Time Series Analysis: Forecasting and Control”, Second Edition, Holden Day, 1976.
[4] G. M. Ljung and G. E. P. Box, “On a Measure of a lack of Fit in Time Series Models”. Biometrika. Vol. 65, Issue. 2, pp. 297-303, 1978.
[5] J. Fan, “Design Adaptive Nonparametric regression”. Journal of American Statistical Association, Vol. 87, pp. 998-1004, 1992.
[6] K. Takezawa, “Introduction to Nonparametric Regression”. John Wiley & Sons, 2006.
[7] N. R. Drapper and H. Smith, “Applied Regression Analysis”. 3rd Edition, John Wiley & Sons, USA. 1998.
[8] S. Makridakis, S. C. Wheelwright and R. J. Hyndma, “Forecasting: Methods and Applications”, 3rd Edition. Wiley publications, 2008.
[9] W. Hardle, “Applied Non-parametric Regression”. Cambridge University Press, 1990.
You do not have rights to view the full text article.
Please contact administration for subscription to Journal or individual article.
Mail us at support@isroset.org or view contact page for more details.