Main Menu
Journals Contents
Information
Download
Publication Certificate
Full Paper View Go Back
Monitoring Process Mean and Variability Using Artificial Neural Networks
S.M. Nimbale1 , V.B. Ghute2
Section:Research Paper, Product Type: Isroset-Journal
Vol.6 ,
Issue.3 , pp.153-158, Jun-2019
CrossRef-DOI: https://doi.org/10.26438/ijsrmss/v6i3.153158
Online published on Jun 30, 2019
Copyright © S.M. Nimbale, V.B. Ghute . This is an open access article distributed under the Creative Commons Attribution License, which permits unrestricted use, distribution, and reproduction in any medium, provided the original work is properly cited.
View this paper at Google Scholar | DPI Digital Library
How to Cite this Paper
- IEEE Citation
- MLA Citation
- APA Citation
- BibTex Citation
- RIS Citation
IEEE Citation
IEEE Style Citation: S.M. Nimbale, V.B. Ghute, “Monitoring Process Mean and Variability Using Artificial Neural Networks,” International Journal of Scientific Research in Mathematical and Statistical Sciences, Vol.6, Issue.3, pp.153-158, 2019.
MLA Citation
MLA Style Citation: S.M. Nimbale, V.B. Ghute "Monitoring Process Mean and Variability Using Artificial Neural Networks." International Journal of Scientific Research in Mathematical and Statistical Sciences 6.3 (2019): 153-158.
APA Citation
APA Style Citation: S.M. Nimbale, V.B. Ghute, (2019). Monitoring Process Mean and Variability Using Artificial Neural Networks. International Journal of Scientific Research in Mathematical and Statistical Sciences, 6(3), 153-158.
BibTex Citation
BibTex Style Citation:
@article{Nimbale_2019,
author = {S.M. Nimbale, V.B. Ghute},
title = {Monitoring Process Mean and Variability Using Artificial Neural Networks},
journal = {International Journal of Scientific Research in Mathematical and Statistical Sciences},
issue_date = {6 2019},
volume = {6},
Issue = {3},
month = {6},
year = {2019},
issn = {2347-2693},
pages = {153-158},
url = {https://www.isroset.org/journal/IJSRMSS/full_paper_view.php?paper_id=1400},
doi = {https://doi.org/10.26438/ijcse/v6i3.153158}
publisher = {IJCSE, Indore, INDIA},
}
RIS Citation
RIS Style Citation:
TY - JOUR
DO = {https://doi.org/10.26438/ijcse/v6i3.153158}
UR - https://www.isroset.org/journal/IJSRMSS/full_paper_view.php?paper_id=1400
TI - Monitoring Process Mean and Variability Using Artificial Neural Networks
T2 - International Journal of Scientific Research in Mathematical and Statistical Sciences
AU - S.M. Nimbale, V.B. Ghute
PY - 2019
DA - 2019/06/30
PB - IJCSE, Indore, INDIA
SP - 153-158
IS - 3
VL - 6
SN - 2347-2693
ER -
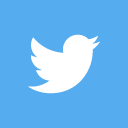
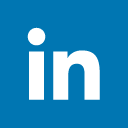
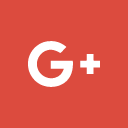
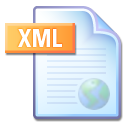
Abstract :
In today`s modern market quality of product is most preferable parameter for customers. In order to fulfill the customer requirements manufacturing industries implementing the advanced technology in order to improve and maintain high quality of product. Consequently its real time need that process variation is to be controlled in very advanced approach.The Shewhart chart is usually used to monitor shifts in process mean, whereas, the R and S charts are most widely used in industry to monitor process variability. In this paper, artificial neural network based approach is developed for monitoring the mean and variability of the process. The ARL performance of the proposed approach is evaluated by using simulation and is compared with traditional Shewhart and S charts under normal process distribution. The proposed study indicates that ANN scheme is effective than and S charts for monitoring the process mean and variability.
Key-Words / Index Term :
Artificial neural network; Statistical process control; X chart; S chart; Average run length
References :
[1] Alhammadi Y. S. and Michael, A. B., “Neural network control chart architecture for monitoring non-conformities in a Poisson process”, Industrial Engineering and Management. 2013,115, pp.2169-0316.
[2] Barghash M. A., “A diverse neural network ensemble team for mean shift detection in X-bar and CUSUM control charts”, Jordan Journal of Mechanical and Industrial Engineering, 2011,5(4), pp.291-300.
[3] Cheng, C. S., “A multi-layered neural network model for detecting changes in the process mean”, Computers and Industrial Engineering, 28(1), 51-61, 1995.
[4] Ho, E. S. and Chang, S. I., “A two neural network approach for process variance change detection and classification”, Journal of Production Research. 1999, 37, pp.1581-1599.
[5] Junsub, Y., Prybutok, V.R., and Clayton, H.R. “ARL comparisons between neural network models and -control charts for quality characteristics that are non-normally distributed”, Economic Quality Control. 2009, 16(1), pp.5–15.
[6] McCulloch, W. S. and Pitts, W., “A logical calculus of the ideas immanent in nervous activity”, The bulletin of mathematical biophysics. 1943, 5(4), pp.115-133.
[7] Moller, M. F. “A scaled conjugate gradient algorithm for fast supervised learning Neural Networks”, 1993, 6(4), pp.525-533.
[8] Montgomery, D. C. “Introduction to statistical quality control”, New York: Wiley. 2009.
[9] Nimbale, S. M. and Ghute, V. B., “Artificial neural network model for monitoring the fraction nonconforming control chart”, International Journal of Science and Research, 4 (3), 2319-7064, 2015.
[10] Nimbale, S. M. and Ghute, V. B., “A neural network based individual control chart”, International Journal of Engineering Research and Technology, 5 (5), 24-27, 2016.
[11] Parker, L. E., “Notes on Multilayer, Feed forward Neural Networks”, Projects in Machine Learning. 2006.
[12] Psarakis, S., “The use of neural networks in statistical process control charts”, Quality and Reliability Engineering International. 2011, 27, 641-650.
[13] Pugh, G. A., “Synthetic neural networks for process control”, Computer and Industrial Engineering. 1989,17, 24-26.
[14] Pugh, G. A., “A comparison of neural network to SPC charts”, Computer and Industrial Engineering. 1991, 21, pp.253-255.
[15] Sandhu, P. S. and Chhabra, S., “A comparative analysis of Conjugate Gradient Algorithms & PSO based neural network approaches for reusability evaluation of procedure based software systems”, Chiang Mai Journal of Science. 2011, 38, pp.123-135.
[16] Smith, A. E., “X-bar and R control chart interpretation using neural computing”, International Journal of Production Research. 1994, 32, pp.309-320.
[17] Stutzle, T., “A neural network approach to quality control charts”, International Workshop on Artificial Neural Networks, pp.1135-1141, 1995.
You do not have rights to view the full text article.
Please contact administration for subscription to Journal or individual article.
Mail us at support@isroset.org or view contact page for more details.