Full Paper View Go Back
The Performance of a Ridge Estimator Based on Harmonic Mean
Satish Bhat1 , R. Vidya2
Section:Research Paper, Product Type: Journal-Paper
Vol.6 ,
Issue.4 , pp.70-76, Aug-2019
CrossRef-DOI: https://doi.org/10.26438/ijsrmss/v6i4.7076
Online published on Aug 31, 2019
Copyright © Satish Bhat, R. Vidya . This is an open access article distributed under the Creative Commons Attribution License, which permits unrestricted use, distribution, and reproduction in any medium, provided the original work is properly cited.
View this paper at Google Scholar | DPI Digital Library
How to Cite this Paper
- IEEE Citation
- MLA Citation
- APA Citation
- BibTex Citation
- RIS Citation
IEEE Style Citation: Satish Bhat, R. Vidya, “The Performance of a Ridge Estimator Based on Harmonic Mean,” International Journal of Scientific Research in Mathematical and Statistical Sciences, Vol.6, Issue.4, pp.70-76, 2019.
MLA Style Citation: Satish Bhat, R. Vidya "The Performance of a Ridge Estimator Based on Harmonic Mean." International Journal of Scientific Research in Mathematical and Statistical Sciences 6.4 (2019): 70-76.
APA Style Citation: Satish Bhat, R. Vidya, (2019). The Performance of a Ridge Estimator Based on Harmonic Mean. International Journal of Scientific Research in Mathematical and Statistical Sciences, 6(4), 70-76.
BibTex Style Citation:
@article{Bhat_2019,
author = {Satish Bhat, R. Vidya},
title = {The Performance of a Ridge Estimator Based on Harmonic Mean},
journal = {International Journal of Scientific Research in Mathematical and Statistical Sciences},
issue_date = {8 2019},
volume = {6},
Issue = {4},
month = {8},
year = {2019},
issn = {2347-2693},
pages = {70-76},
url = {https://www.isroset.org/journal/IJSRMSS/full_paper_view.php?paper_id=1433},
doi = {https://doi.org/10.26438/ijcse/v6i4.7076}
publisher = {IJCSE, Indore, INDIA},
}
RIS Style Citation:
TY - JOUR
DO = {https://doi.org/10.26438/ijcse/v6i4.7076}
UR - https://www.isroset.org/journal/IJSRMSS/full_paper_view.php?paper_id=1433
TI - The Performance of a Ridge Estimator Based on Harmonic Mean
T2 - International Journal of Scientific Research in Mathematical and Statistical Sciences
AU - Satish Bhat, R. Vidya
PY - 2019
DA - 2019/08/31
PB - IJCSE, Indore, INDIA
SP - 70-76
IS - 4
VL - 6
SN - 2347-2693
ER -
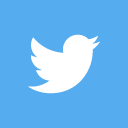
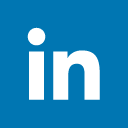
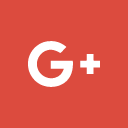
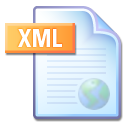
Abstract :
Linear dependence between predictors is one of the serious issues in regression analysis. Due to near linear dependence (or multicollinearity) between any two or more predictors, ordinary least squares (OLS) method will yield unstable estimates to the regression coefficients. In the literature, several techniques like Ridge regression, Principal component regression, Partial least squares regression, Liu method of regression etc., have been developed to overcome problem of multicollinearity. Among them Ridge regression is one of the most widely used methods, which will yield more stable estimate’s as compared to OLS estimator. Here we propose a new ridge estimator based on Harmonic mean method. Performance of the ridge estimators is evaluated both theoretically and empirically under a wide range of degree of multicollinearity and error variances. Both methods have indicated that the performance of the suggested estimator is slightly more stable than some existing estimators, which are considered under study with respect to various degrees of multicollinearity, sample size, and error variance.
Key-Words / Index Term :
Multiple linear regression (MLR), Multicollinearity, Ridge regression, and MSE
References :
[1] R. Nair, V. Jain, A. Bhagat, R. Agarwal, “An Efficient approach for Sentiment Analysis Using Regression analysis Technique”, International Journal of Computer Sciences and Engineering, Vol. 7, issue 3, pp. 161-165, 2019.
[2] T. Sur, A. Nath, “An innovative Method to Calculate the Economic Development Index of Important Cities in West Bengal From Satellite Imagery”, International Journal of Computer Sciences and Engineering, Vol. 7, issue 4, pp. 411-417, 2019.
[3] A. E. Hoerl, “Application of Ridge Analysis to Regression Problems”, Chemical Engineering Progress, Vol. 58, pp. 54–59, 1962.
[4] A.E. Hoerl, R. W. Kennard, “Ridge Regression: Biased Estimation for Non Orthogonal Problems”, Technometrics, Vol. 12, pp. 69–82, 1970.
[5] A. E. Hoerl, R.W. Kennard, K. F. Baldwin, “Ridge Regression: Some Simulations”, Communications in Statistics-Theory and Methods, Vol. 4, No., 2, pp.105–123, 1975.
[6] T. Hastie, R. Tibshirani, “Generalised Additive Models”, Statistical Science, Vol. 1, No., 3, pp. 297–318, 1986.
[7] A. M. Halawa, M.Y. El Bassiouni, “Tests of Regression Coefficients under Ridge Regression Models”, Journal of Statistical Computation and Simulation, Vol. 65, pp. 341–356, 2000.
[8] J. F. Lawless, P. Wang, “A Simulation Study of Ridge and Other Regression Estimators”, Communications in Statistics-Theory and Methods, Vol. 5, No., 4, pp. 307–323, 1976.
[9] M. Nomura, “On the Almost Unbiased Ridge Regression Estimator”, Communications in Statistics – Simulation and Computation, Vol. 17, No., 3, pp. 729–743, 1988.
[10]G. Khalaf, G. Shukur, “Choosing Ridge Parameter for Regression Problems”, Communications in Statistics-Theory and Methods, Vol. 34, pp. 1177–1182, 2005.
[11]A.V. Dorugade, D. N. Khashid, “Alternative Method for Choosing Ridge Parameter for Regression”, Applied Mathematical Sciences, Vol .4, No., 9, pp. 447–456, 2010.
[12]A. V. Dorugade, “New Ridge Parameters for Ridge Regression”, Journal of the Association of Arab Universities for Basic and Applied Sciences. Vol. 15, pp. 94–99, 2014.
[13]S. Bhat, R. Vidya, “Improvement on Ridge Regression Estimator”, In the Proceedings of the National Conference on Statistical Methods and Data Analysis, Yuvaraja’s College, Mysore, India, ISBN No. 81-7877-093-8, pp. 65–71, 2014.
[14]S. Bhat, R. Vidya, “A Comparative Study on the Performance of New Ridge Estimators”, Pakistan Journal of Statistics and Operations Research (PJSOR), Vol. 12, No. 2, pp. 317–325, 2016.
[15]H. D. Vinod, A. Ullah, “Recent Advances in Regression Methods”, Marcel Dekker, New York, 1981.
[16]K. Liu, “Using Liu Type Estimator to Combat Collinearity”, Communications in Statistics-Theory and Methods. Vol. 32, pp. 1009–1020, 2003.
[17]S. Bhat, R. Vidya, “A New Ridge Estimator and its Performance”, In the Proceedings of the National Conference on Operations Management, Analytics and Statistical Methods(NOMAS-2017), Mysore, India, ISBN No. 978-9383302-20-8, 2017a.
[18]S. Bhat, “Contributions to the study of Partial Least Squares Regression(PLSR)”, Ph. D. Thesis, Submitted to University of Mysore, Mysuru, India, chapter 3, pp. 71–105, 2017b.
[19]G. Khalaf, “A Proposed Ridge Parameter to Improve the Least Square Estimator”, Journal of Modern Applied Statistical Method, Vol. 11, No., 2, pp. 443–449, 2012.
[20]B. M. Kibria, “Performance of Some Ridge Regression Estimators”, Communications in Statistics-Simulation and Computation, Vol. 32, pp. 419–435, 2003.
[21]S. Mardikyan, E. Cetin, “Efficient Choice of Biasing Constant for Ridge Regression”, International Journal of Contemporary Mathematical Sciences, Vol. 3, pp. 527–547, 2008.
[22]D. C. Montgomery, E. A. Peck, G. G. Vining, “Introduction to linear regression Analysis”, 3rd Edition, John Wiley and Sons (Asia), Singapore, 2003.
[23]G. Muniz, B. M. Kibria, “On Some Ridge Regression Estimators-An Empirical Comparisons”, Communications in Statistics –Simulation and Computation, Vol. 38, No., 3, pp. 621–630, 2009.
[24]M. Yazid Al-Hassan, “Performance of a New Ridge Regression Estimator”, Journal of the Association of Arab Universities for Basic and Applied Sciences, Vol. 9, 23–26, 2010.
You do not have rights to view the full text article.
Please contact administration for subscription to Journal or individual article.
Mail us at support@isroset.org or view contact page for more details.