Full Paper View Go Back
Covariate Measurement Error in a Non-Linear Model for Longitudinal Data
Rosa C. S. Oliveira1
- Matemática, Faculdade de Ciências, Porto, Portugal.
Correspondence should be addressed to: rosita21@gmail.com.
Section:Research Paper, Product Type: Journal-Paper
Vol.7 ,
Issue.2 , pp.17-33, Apr-2020
Online published on Apr 30, 2020
Copyright © Rosa C. S. Oliveira . This is an open access article distributed under the Creative Commons Attribution License, which permits unrestricted use, distribution, and reproduction in any medium, provided the original work is properly cited.
View this paper at Google Scholar | DPI Digital Library
How to Cite this Paper
- IEEE Citation
- MLA Citation
- APA Citation
- BibTex Citation
- RIS Citation
IEEE Style Citation: Rosa C. S. Oliveira, “Covariate Measurement Error in a Non-Linear Model for Longitudinal Data,” International Journal of Scientific Research in Mathematical and Statistical Sciences, Vol.7, Issue.2, pp.17-33, 2020.
MLA Style Citation: Rosa C. S. Oliveira "Covariate Measurement Error in a Non-Linear Model for Longitudinal Data." International Journal of Scientific Research in Mathematical and Statistical Sciences 7.2 (2020): 17-33.
APA Style Citation: Rosa C. S. Oliveira, (2020). Covariate Measurement Error in a Non-Linear Model for Longitudinal Data. International Journal of Scientific Research in Mathematical and Statistical Sciences, 7(2), 17-33.
BibTex Style Citation:
@article{Oliveira_2020,
author = {Rosa C. S. Oliveira},
title = {Covariate Measurement Error in a Non-Linear Model for Longitudinal Data},
journal = {International Journal of Scientific Research in Mathematical and Statistical Sciences},
issue_date = {4 2020},
volume = {7},
Issue = {2},
month = {4},
year = {2020},
issn = {2347-2693},
pages = {17-33},
url = {https://www.isroset.org/journal/IJSRMSS/full_paper_view.php?paper_id=1828},
publisher = {IJCSE, Indore, INDIA},
}
RIS Style Citation:
TY - JOUR
UR - https://www.isroset.org/journal/IJSRMSS/full_paper_view.php?paper_id=1828
TI - Covariate Measurement Error in a Non-Linear Model for Longitudinal Data
T2 - International Journal of Scientific Research in Mathematical and Statistical Sciences
AU - Rosa C. S. Oliveira
PY - 2020
DA - 2020/04/30
PB - IJCSE, Indore, INDIA
SP - 17-33
IS - 2
VL - 7
SN - 2347-2693
ER -
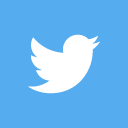
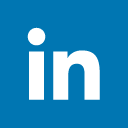
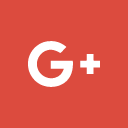
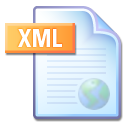
Abstract :
In this paper, we study nonlinear generalized regression models for the analysis of longitudinal data. We indicate a two-stage approach that, in the first stage models a linear model to the longitudinal data to estimate the random effect that characterizes the trajectory for each individual and in the second stage uses the estimated intercept and slope as predictors for the covariates used in a probit model. We show that the straight use of the estimates in the probit model produces biased estimates of their outcome. We show how to adapt a Regression Calibration and a Pseudo-Likelihood approach to this context and compare these approaches with a naive analysis where the estimation error is ignored. Regression Calibration and Pseudo-Likelihood methods seem to be the best choice as they perform well, even for small sample size, if data is not very noisy. It is fair to say, mainly, that the Regression Calibration and Pseudo-Likelihood method perform equally well, nonetheless, Regression Calibration seems to perform a bit better. Nevertheless, naive approach has smaller Mean Squared Error, has a great absolute bias. Regression Calibration seems to be the best. Our study indicates that correcting for measurement errors instead of falsely assume that errors are not present will produce less bias than ignoring exposure measurement error in the analysis.
Key-Words / Index Term :
measurement error, errors-in-variables, general nonlinear model, longitudinal data
References :
[1]. Póvoa P. C-reactive protein: a valuable marker of sepsis.. Intensive Care Medicine 2002; 28 (3): 235–243.
[2]. Tosteson JP, Demidenko E. Covariate measurement error and the estimation of random effect parameters in a mixed modelfor longitudinal data. Statistics in Medicina 1998; 17: 1959–1971.
[3]. Carroll R, Ruppert D, Stefanski L, Crainiceanu C. Measurement Error in Nonlinear Models: A Modern Perspective. Chapman and Hall . 2006.
[4]. Rosner B, Spiegelman D, Willett WC. Correction of logistic regression relative risk estimates and confidence intervals formeasurement error: the case of multiple covariates measured with error. American Journal of Epidemiology 1990; 132:734–745.
[5]. W. SD, . PK. Likelihood analysis for errors-in-variables regression with replicate measurements.. Biometrika 1996; 83:813–824.
[6]. Carroll RJ, Küchenhoff H, Lombard F, Stefanski LA. Asymptotics for the SIMEX estimator in nonlinear measurement errormodels. Journal of the American Statistical Association, 1996; 91: 242–250.
[7]. R.C. B, R.A. B, F.B. C, R.P. D, A.M. F, W.J. KWSRS. Definitions for sepsis and organ failure and guidelines for the use ofinnovative therapies in sepsis.. In: . 101. ; 1992: 1644-1655.
[8]. Póvoa P, M. TPA, Carneiro AH, SACiUCI. PCASSG. C-reactive protein, an early marker of community-acquired sepsis resolution: a multi-center prospective observational study. Critical Care 2011; 15 (4).
[9]. Lawrence Gould CMIJQGMSBF. Joint modeling of survival and longitudinal non-survival data: current methods and issues.Report of the DIA Bayesian joint modeling working group.. Stat Med. 2015; 34(14): 2181-95.
[10]. McCulloch CE, Searle SR. Generalized, Linear and Mixed Models. Wiley InterScience . 2001.
[11]. Akaike H. Information Theory and an Extension of The Maximum Likelihood Principle. International Symposium on Information Theory 1973; 2: 267–281.
[12]. Erling BA. Asymptotic Properties of Conditional Maximum-Likelihood Estimators. Journal of the Royal Statistical Society, Series B 1971; 32: 283–301.
[13]. Breslow NE, Holubkov R.Weighted likelihood, pseudo-likelihood and maximum likelihood methods for logistic regression analysis of two-stage data. Statistics in Medicine 1997; 16: 103–116.
[14]. Carroll RJ, Spiegelman CH, Gordon Lan KK, Bailey KT, Abbott RD. On errors-in-variables for binary regression models. Biometrika 1984; 71: 19–25.
[15]. Carroll RJ, Küchenhoff H, Lombard F, Stefanski LA. Asymptotics for the SIMEX estimator in nonlinear measurement error models. Journal of the American Statistical Association, 1996; 91: 242–250.
[16]. Chen B, Yi GY, Cook RJ.Weighted Generalized Estimating Functions for Longitudinal Response and Covariate Data That Are Missing at Random. Journal of the American Statistical Association 2010; 105: 336–353.
[17]. J. G. C-reactive protein: risk factor, biomarker and/or therapeutic target–. Canadian Journal of Cardiology 2010; 26, Suppl A: 41A–44A.
[18]. Huang YJ,WangCY. Consistent functional methods for logistic regression with errors in covariates. Journal of the American Statistical Association 2001; 96: 1469–1482.
[19]. Ma Y, Tsiatis AA. Closed form semiparametric estimators for measurement error models. Stat. Sin. 2006; 16: 183–193.
[20]. Chen MH, Ibrahim JG, Shao QM. Propriety of the Posterior Distribution and Existence of the MLE for Regression Models with Covariates Missing at Random. Journal of the American Statistical Association 2004; 99: 421–438.
[21]. Nugent W, Graycheck L, Basham R. A Devil Hidden in the Details: The Effects of Measurement Error in Regression Analysis. Journal of Social Service Research 2000; 27: 53–75.
[22]. Cox C. Nonlinear quasi-likelihood models: applications to continuous proportions. Computational Statistics and Data Analysis 1996; 21: 449–461.
[23]. Cox DR. Partial likelihood. Biometrika 1975; 62: 269–276.
[24]. Diggle PJ, Heagerty P, Liang KY, Zeger SL. Analysis of Longitudinal Data, 2nd edition. Oxford, U.K.: Oxford University Press. . 2002.
[25]. Fitzmaurice GM, Laird NM, Ware JH. Applied longitudinal analysis. Wiley-Interscience . 2004.
[26]. Fitzmaurice GM, Laird NM, Zahner GEP. Multivariate Logistic Models for Incomplete Binary Responses. Journal of the American Statistical Association 1996; 91: 99–108.
[27]. Fitzmaurice GM, Lipsitz SR, Molenberghs G, Ibrahim JG. A Protective Estimator for Longitudinal Binary Data Subject to Non-Ignorable Non-Monotone Missingness. Journal of the Royal Statistical Society. Series A 2005; 168: 723–735.
[28]. Fitzmaurice GM, Molenberghs G, Lipsitz SR. Regression Models for Longitudinal Binary Responses with Informative Drop-Outs. Journal of the Royal Statistical Society. Series B 1995; 57: 691–704.
[29]. Fuller W. Measurement Error Models. John Wiley and Sons . 1987.
[30]. Laird NM, Ware JH. Random effects models for longitudinal data. Biometrics 1982; 38: 963–974.
[31]. Li Y, Lin X. Covariate Measurement Errors in Frailty Models for Clustered Survival Data. Biometrika 2000; 87 (4): 849–866.
[32]. Chaganty NR, Joe H. Efficiency of Generalized Estimating Equations for Binary Responses. Journal of the Royal Statistical Society, Series B 2004; 66: 851–860.
[33]. Cook JR, Stefanski L. Simulation-extrapolation estimation in parametric measurement error models. Journal of the American Statistical Association, 1994; 89: 1314–1328.
[34]. W. SD. Semiparametric maximum likelihood for measurement error regression.. Biometrika 2001; 57: 53–61.
[35]. Stefanski LA, Buzas JS. Instrumental variable estimation in binary regression measurement error models. Journal of the American Statistical Association 1995; 90: 541–550.
[36]. Stefanski LA, Carroll R. Covariate measurement error in logistic regression. Annals of Statistics 1985; 13: 1335–1351.
[37]. Stefanski LA, Carroll R. Conditional scores and optimal scores for generalized linear measurement-error models. Biometrika 1987; 74: 703–716.
You do not have rights to view the full text article.
Please contact administration for subscription to Journal or individual article.
Mail us at support@isroset.org or view contact page for more details.