Full Paper View Go Back
Prediction of Stock Price Movement Using Knowledge Graph Embeddings
Eric Kwamena Asibu Yartey1
Section:Research Paper, Product Type: Journal-Paper
Vol.7 ,
Issue.6 , pp.12-19, Dec-2020
Online published on Dec 31, 2020
Copyright © Eric Kwamena Asibu Yartey . This is an open access article distributed under the Creative Commons Attribution License, which permits unrestricted use, distribution, and reproduction in any medium, provided the original work is properly cited.
View this paper at Google Scholar | DPI Digital Library
How to Cite this Paper
- IEEE Citation
- MLA Citation
- APA Citation
- BibTex Citation
- RIS Citation
IEEE Style Citation: Eric Kwamena Asibu Yartey, “Prediction of Stock Price Movement Using Knowledge Graph Embeddings,” International Journal of Scientific Research in Mathematical and Statistical Sciences, Vol.7, Issue.6, pp.12-19, 2020.
MLA Style Citation: Eric Kwamena Asibu Yartey "Prediction of Stock Price Movement Using Knowledge Graph Embeddings." International Journal of Scientific Research in Mathematical and Statistical Sciences 7.6 (2020): 12-19.
APA Style Citation: Eric Kwamena Asibu Yartey, (2020). Prediction of Stock Price Movement Using Knowledge Graph Embeddings. International Journal of Scientific Research in Mathematical and Statistical Sciences, 7(6), 12-19.
BibTex Style Citation:
@article{Yartey_2020,
author = {Eric Kwamena Asibu Yartey},
title = {Prediction of Stock Price Movement Using Knowledge Graph Embeddings},
journal = {International Journal of Scientific Research in Mathematical and Statistical Sciences},
issue_date = {12 2020},
volume = {7},
Issue = {6},
month = {12},
year = {2020},
issn = {2347-2693},
pages = {12-19},
url = {https://www.isroset.org/journal/IJSRMSS/full_paper_view.php?paper_id=2210},
publisher = {IJCSE, Indore, INDIA},
}
RIS Style Citation:
TY - JOUR
UR - https://www.isroset.org/journal/IJSRMSS/full_paper_view.php?paper_id=2210
TI - Prediction of Stock Price Movement Using Knowledge Graph Embeddings
T2 - International Journal of Scientific Research in Mathematical and Statistical Sciences
AU - Eric Kwamena Asibu Yartey
PY - 2020
DA - 2020/12/31
PB - IJCSE, Indore, INDIA
SP - 12-19
IS - 6
VL - 7
SN - 2347-2693
ER -
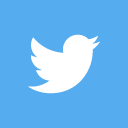
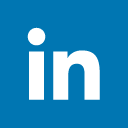
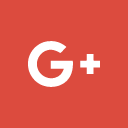
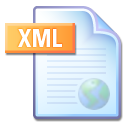
Abstract :
Given the advancement of artificial intelligence and increased computational capabilities to handle a massive amount of web Information, Knowledge graphs (KG) and Knowledge graphs (KG) embeddings have proven useful in modelling and representing factual information in various domains such as medical diagnosis, web search, semantic parsing, speech recognition, question answering, named entity disambiguation, link prediction, recommendation system among others. While TransE model has been adopted by some researchers in the field of finance to generate knowledge graph embeddings for their proposed stock prediction models, it has also been under criticism. Onuki et al. had claimed in their research work that their proposed KGML model outperforms TransE model in terms of predictive accuracy, learning speed, and convergence speed of learning. This study evaluates the effect that learned embedding vectors from TransE and KGML models have on the performance of a TCN stock prediction model. Results have shown that KG embeddings are powerful predictors, and the overall best KG embeddings are that of the TransE model.
Key-Words / Index Term :
Knowledge Graph, Knowledge Graph Embeddings, TransE Model, KGML Model, Temporal Convolution Network (TCN), Stock Price Movement Prediction
References :
[1] E. F. Fama, "The Behavior of Stock-Market Prices," The Journal of Business, Vol. 38, No.1, pp.34-105, 1965.
[2] B. G. Malkiel, "Efficient Market Hypothesis," in The New Palgrave: Finance., London, Palgrave Macmillan, 1989, pp. 127-134.
[3] Y. Zhai , A. Hsu and S. K. Halgamuge, "Combining News and Technical Indicators in Daily Stock Price Trends Prediction," Advances in Neural Networks,Vol 4493, pp.1087-1097, 2007.
[4] B. Xie, R. Passonneau, L. Wu and G. G. Creamer, "Semantic frames to predict stock price movement," in Proceedings of the 51st Annual Meeting of the Association for Computational Linguistics (ACL), Sofia, Bulgaria, pp.873-883, 2013.
[5] J. Si, A. Mukherjee, B. Liu, Q. Li, H. Li and X. Deng, "Exploiting Topic based Twitter Sentiment for Stock Prediction," in Proceedings of the 51st Annual Meeting of the Association for Computational Linguistics, Sofia, Bulgaria, pp.1139-1145, 2013.
[6] J. Si, A. Mukherjee, B. Liu, S. J. Pan, Q. Li and H. Li, "Exploiting Social Relations and Sentiment for Stock Prediction," in Proceedings of the 2014 Conference on Empirical Methods in Natural Language Processing (EMNLP), Doha, Qatar, pp.1139-1145, 2014.
[7] E. J. Ruiz, V. Hristidis, C. Castillo, A. Gionis and A. Jaimes, "Correlating financial time series with micro-blogging activity," in Proceedings of the fifth ACM international conference on Web search and data mining, USA, pp.513-512, 2012.
[8] H. Lee, M. Surdeanu, B. MacCartney and D. Jurafsky, "On the Importance of Text Analysis for Stock Price Prediction," in In Proceedings of the Ninth International Conference on Language Resources and Evaluation (LREC`14), Reykjavik, Iceland, pp.1170-1175, 2014.
[9] P. Wang, H. Jiang, J. Xu and Q. Zhang, "Knowledge Graph Construction and Applications for Web Search and Beyond," Data Intelligence, Vol.1, No.4, pp.333-349, 2019.
[10] L. Heck and H. Huang, "Deep learning of knowledge graph embeddings for semantic parsing of Twitter dialogs," in 2014 IEEE Global Conference on Signal and Information Processing (GlobalSIP), Atlanta, GA, USA, pp.597-601, 2014.
[11] Moschitti, a. Tymoshenko, P. Alexopoulos, A. Walker, M. Nicosia, G. Vetere, A. Faraotti, M. Monti, J. Z. Pan, H. Wu and Y. Zhao, "Question Answering and Knowledge Graphs," in Exploiting Linked Data and Knowledge Graphs in Large Organisations, Springer, Cham, pp.181-212, 2017
[12] Lin, S. Ong, C. Williams and Y. B. Zhang, "Named Entity Disambiguation with Knowledge Graphs," Institute for Applied Computational Science, Harvard university, USA, 2019.
[13] H. Xiao, M. Huang and X. Zhu, "From One Point to a Manifold: Knowledge Graph Embedding for Precise Link Prediction," in Proceedings of the Twenty-Fifth International Joint Conference on Artificial Intelligence, New York, USA, pp.1315-1321, 2016.
[14] Z. Sun, J. Yang, J. Zhang, A. Bozzon, L.-K. Huang and C. Xu, "Recurrent knowledge graph embedding for effective recommendation," in Proceedings of the 12th ACM Conference on Recommender Systems (RecSys `18), New York, USA, pp.297-305, 2018.
[15] Y. Onuki, T. Murata, S. Nukui, S. Inagi, X. Qiu, M. Watanabe and H. Okamoto, "Relation prediction in knowledge graph by Multi-Label Deep Neural Network," Applied Network Science, Vol.4, No.20, 2019.
[16] Z. Liu and X. Han, "Deep Learning in Knowledge Graph," in Deep Learning in Natural Language Processing, Springer, Singapore, pp.117-146, 2018.
[17] Q. Wang, Z. Mao, B. Wang and L. Guo, "Knowledge Graph Embedding: A Survey of Approaches and Applications," IEEE Transactions on Knowledge and Data Engineering, Vol.29, No.12, pp.2724-2473, 2017.
[18] Ringler and H. Paulheim, "One Knowledge Graph to Rule Them All? Analyzing the Differences Between DBpedia, YAGO, Wikidata & co.," in Kern-Isberner G., Fürnkranz J., Thimm M. (eds) KI 2017: Advances in Artificial Intelligence. KI 2017. Cham, pp.366-372, 2017.
[19] Bordes, N. Usunier, A. García-Durán, J. Weston and O. Yakhnenko, "Translating embeddings for modeling multi-relational data," In NIPS`13: Proceedings of the 26th International Conference on Neural Information Processing Systems, Lake Tahoe, USA.pp.2787-2795, 2013.
[20] T. Mikolov, I. Sutskever, K. Chen, G. Corrado and J. Dean, "Distributed Representations of Words and Phrases and their Compositionality," in NIPS`13: Proceedings of the 26th International Conference on Neural Information Processing Systems, Lake Tahoe, USA.pp.3111-3119, 2013.
[21] Y. Liu, Q. Zeng, H. Yang and A. Carrio, "Stock Price Movement Prediction from Financial News with Deep Learning and Knowledge Graph Embedding," In Knowledge Management and Accquisition for intelligent Systems. Springer, Cham, Switzerland, pp.102-113, 2018.
[22] Y. Liu, Q. Zeng, J. O. Meré and H. Yang, "Anticipating Stock Market of the Renowned Companies: A Knowledge Graph Approach," Hindawi Complexity, Vol.2019, 2019.
[23] X. Ding, Y. Zhang, T. Liu and J. Duan, "Knowledge-Driven Event Embedding for Stock Prediction," in Proceedings of COLING 2016, the 26th International Conference on Computational Linguistics: Technical Papers, Osaka, Japan, pp.2133-2142, 2016.
[24] S. Deng, N. Zhang, W. Zhang, J. Chen, J. Z. Pan and H. Chen, "Knowledge-Driven Stock Trend Prediction and Explanation via Temporal Convolutional Network," in Companion Proceedings of The 2019 World Wide Web Conference (WWW `19), New York, NY, USA, pp.678-685, 2019.
[25] M. Naved and P. Srivastava, "Profitability of Oscillators used in Technical Analysis for Financial Market," Advances in Economics and Business Management (AEBM), Vol.2, No.9, pp.925-931, 2015.
[26] R. D. Almeida, G. Reynoso-Meza and M. T. A. Steiner, "Multi-objective optimization approach to stock market technical indicators," in 2016 IEEE Congress on Evolutionary Computation (CEC), Vancouver, BC, Canada, pp.3670-3677, 2016.
[27] S. Bai, J. Z. Kolter and V. Koltun, "An Empirical Evaluation of Generic Convolutional and Recurrent Networks for Sequence Modeling", ArXiv, Vol.abs/1803.01271, 2018.
[28] Borovykh, S. Bohte and C. Oosterlee, "Dilated convolutional neural networks for time series forecasting," Journal of Computational Finance, Vol.22, No.4, pp.73-101, 2018.
[29] K. He, X. Zhang, S. Ren and J. Sun, "Deep Residual Learning for Image Recognition," in In Proceedings of the IEEE Conference on Computer Vision and Pattern Recognition, Las Vegas, NV, USA, pp.770-778, 2016.
[30] L. Costabello, S. Pai, C. L. Van, R. McGrath, N. McCarthy and P. Tabacof, "AmpliGraph: a Library for Representation Learning on Knowledge Graphs," zenodo, 2019.
[31] Goutte and E. Gaussier, "A Probabilistic Interpretation of Precision, Recall and F-Score, with Implication for Evaluation," in Advances in Information Retrieval. ECIR 2005. Lecture Notes in Computer Science, Berlin, Germany, pp.345-359, 2005.
[32] J. Brownlee, Better Deep Learning: Train Faster, Reduce Overfitting, and Make Better Predictions, Machine Learning Mastery, 2018.
You do not have rights to view the full text article.
Please contact administration for subscription to Journal or individual article.
Mail us at support@isroset.org or view contact page for more details.