Full Paper View Go Back
Mohammed Alqatqat1 , Ma Tie Feng2
Section:Research Paper, Product Type: Journal-Paper
Vol.8 ,
Issue.1 , pp.56-71, Feb-2021
Online published on Feb 28, 2021
Copyright © Mohammed Alqatqat, Ma Tie Feng . This is an open access article distributed under the Creative Commons Attribution License, which permits unrestricted use, distribution, and reproduction in any medium, provided the original work is properly cited.
View this paper at Google Scholar | DPI Digital Library
How to Cite this Paper
- IEEE Citation
- MLA Citation
- APA Citation
- BibTex Citation
- RIS Citation
IEEE Style Citation: Mohammed Alqatqat, Ma Tie Feng, “Comparison between Markov chain Techniques for Future Forecasting Using Production and Consumption of Electric Energy,” International Journal of Scientific Research in Mathematical and Statistical Sciences, Vol.8, Issue.1, pp.56-71, 2021.
MLA Style Citation: Mohammed Alqatqat, Ma Tie Feng "Comparison between Markov chain Techniques for Future Forecasting Using Production and Consumption of Electric Energy." International Journal of Scientific Research in Mathematical and Statistical Sciences 8.1 (2021): 56-71.
APA Style Citation: Mohammed Alqatqat, Ma Tie Feng, (2021). Comparison between Markov chain Techniques for Future Forecasting Using Production and Consumption of Electric Energy. International Journal of Scientific Research in Mathematical and Statistical Sciences, 8(1), 56-71.
BibTex Style Citation:
@article{Alqatqat_2021,
author = {Mohammed Alqatqat, Ma Tie Feng},
title = {Comparison between Markov chain Techniques for Future Forecasting Using Production and Consumption of Electric Energy},
journal = {International Journal of Scientific Research in Mathematical and Statistical Sciences},
issue_date = {2 2021},
volume = {8},
Issue = {1},
month = {2},
year = {2021},
issn = {2347-2693},
pages = {56-71},
url = {https://www.isroset.org/journal/IJSRMSS/full_paper_view.php?paper_id=2287},
publisher = {IJCSE, Indore, INDIA},
}
RIS Style Citation:
TY - JOUR
UR - https://www.isroset.org/journal/IJSRMSS/full_paper_view.php?paper_id=2287
TI - Comparison between Markov chain Techniques for Future Forecasting Using Production and Consumption of Electric Energy
T2 - International Journal of Scientific Research in Mathematical and Statistical Sciences
AU - Mohammed Alqatqat, Ma Tie Feng
PY - 2021
DA - 2021/02/28
PB - IJCSE, Indore, INDIA
SP - 56-71
IS - 1
VL - 8
SN - 2347-2693
ER -
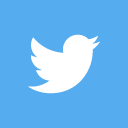
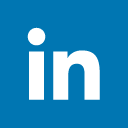
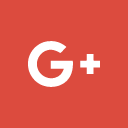
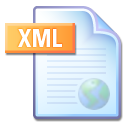
Abstract :
Forecasting of electric energy production and consumption is a fundamental phenomenon for a country’s planning. This study aims to make a comparison between four forecasting methods namely Markov developed by Fiering and Thomas , Markov chains Transformation , Fuzzy Time Series Markov Chain , and proposed method By combining two methods Markov developed by Fiering and Thomas and Markov chains Transformation of predict future production and consumption of electricity in 2016, 2017, 2018,2019 this study examined the accuracy of the prediction of production and consumption of electric energy based on monthly data for production and consumption of electricity during 2016 to 2019 in China. By comparing the four models using the Mean Absolute Percentage Error (MAPE), proposed method was found as the most appropriate model for predicting and analysing the data of interest. Based on this model, production and consumption of electricity can be forecasted. The predictive values were consistent with the original values of the series, which indicated the efficiency of the proposed method in production electric with lowest MAPE but the predictive of consumption electric get low MAPE by Markov chains Transformation and we note that the random number in Markov developed by Fiering and Thomas has impact in our proposed method.
Key-Words / Index Term :
Time series analysis, predictive modelling, Markov chains, Fuzzy time series
References :
[1] Weron R. “Electricity price forecasting: A review of the state-of-the-art with a look into the future”, International Journal of Forecasting.Vol.30, Issue.4, pp.1030-1081, 2014
[2] Bianco V, Manca O, Nardini S, et al. “Analysis and forecasting of nonresidential electricity consumption in Romania”, Applied Energy Journal. Vol.87, Issue.11, pp.3584-3590, 2010
[3] An N, Zhao W, Wang J, et al. ” Using multi-output feedforward neural network with empirical mode decomposition based signal filtering for electricity demand forecasting”. Energy Journal. Vol.49, pp.279-288, 2013
[4] Zhao W, Wang J, Lu H. ”Combining forecasts of electricity consumption in China with time-varying weights updated by a high-order Markov chain model”, Omega. Vol.45, pp.80-91, 2014
[5] Göb R, Lurz K, Pievatolo A. ” Electrical load forecasting by exponential smoothing with covariates”. Applied Stochastic Models in Business and Industry.Vol.29, Issue 6, pp.629-645, 2013
[6] Taylor JW. ”Short-term electricity demand forecasting using double seasonal exponential smoothing”. Journal of the Operational Research Society. Vol.54, Issue.8, pp.799-805, 2017
[7] Rahman A, Ahmar AS. Forecasting of primary energy consumption data in the United States: A comparison between ARIMA and Holter-Winters models. 2017;1885:020163. Vol.87, Issue.11, pp.3584-3590, 2010
[8] Kristie Seymore AM, ”Ronald Rosenfeld. Learning Hidden Markov Model Structure for Information Extraction”. AAAI Technical Report WS. Vol.99, Issue.11, pp.37-42, 1999
[9] Zhou P, Ang B, Poh K. ”A trigonometric grey prediction approach to forecasting electricity demand”. Energy. Vol.31, Issue.14, pp.2839-2847, 2006
[10] Azadeh A, Saberi M, Seraj O. ”An integrated fuzzy regression algorithm for energy consumption estimation with non-stationary data: A case study of Iran”. Energy.Vol.35, Issue.6, pp.2351-2366, 2010
[11] Taylor J W, Majithia S. ”Using combined forecasts with changing weights for electricity demand profiling”. Journal of the Operational Research Society Vol.51, Issue.1, pp.72-82, 2000
[12] Castelnuovo, Efrem, 2003. "Taylor rules, omitted variables, and interest rate smoothing in the US," Economics Letters, Elsevier, Vol.81, , Issue.1, pp.55-59, 2003
[13] Taylor J W, Majithia S. "Using combined forecasts with changing weights for electricity demand profiling [J] ". Journal of the Operational Research Society, Vol.51, pp.72-82, 2000
[14] Gupta RS. “Hydrology and hydraulic systems”. Waveland Press, USA, pp. 242-245, 2016.
[15] Guerrero VM. “Time?series analysis supported by power transformations”. Journal of Forecasting. Vol.12, Issue.1,pp.37-48, 1993
[16] Fiering MB, Bund B, Jackson BB. “Synthetic stream flows”. Vol. 1. American Geophysical Union; 1971. Vol.32, Issue.1, pp.73-76, 1971
[17] Mark A. Pinsky, Samuel Karlin, in “An Introduction to Stochastic Modeling” (Fourth Edition), USA, pp. 87-102, 2011.
[18] Stephen And rilli, David Hecker, “ in Elementary Linear Algebra (Fourth Edition) ”, USA, pp. 318-322, 2010.
[19] W.J. Stewart, “Introduction to Numerical Solutions of Markov Chains”, Princeton University Press, Princeton, USA, pp. 65-68, 1994.
[20] Tsaur, R. C. A” Fuzzy Time Series- Markov Chain Model with an Application to Forecast the Exchange Rate between the Taiwan and US Dollar”. International journal of innovative computing information and control, Vol.8, Issue.7B, pp.4931-4942, 2010
[21] Xihao, S. dan Yimin, L. 2008. ” Average-Based Fuzzy Time Series Models for Forecasting Shanghai Compound Index”. World journal of modelling and simulation. Vol.4, , Issue 2 ,pp.104-111, 2008
[22] Yaffee RA, McGee M.” An introduction to time series analysis and forecasting: with applications of SAS”® and SPSS®. Elsevier; Netherlands, pp. 173-182, 2000.
[23] Taylor, J.W. Short-term electricity demand forecasting using double seasonal exponential smoothing. Journal of the Operational Research Society, Vol .54, pp.799-805, 2003
[24] Akgün et al., Organizational learning: A socio-cognitive framework. Human Relations, Vol 56, pp. 839-868, 2003
[25] Montgomery, D. C., Jennings, C. L., Kulahci, M. Introduction to Time Series Analysis and Forecasting. John Wiley & Sons, Inc. USA, pp.212-236, 2008.
[26] Box, G. E. P., & Jenkins, G. M.. Time series analysis: Forecasting and control. San Francisco: Holden-Day. USA, pp.341-348, 1976
[27] Brown, R. G. Smoothing, Forecasting and Prediction of Discrete Time Series. Prentice Hall, Englewood Cliffs, N. J. USA, pp.78-85, 1962.
You do not have rights to view the full text article.
Please contact administration for subscription to Journal or individual article.
Mail us at support@isroset.org or view contact page for more details.