Full Paper View Go Back
Comparison of Different Parametric Modelling for Child Dengue Patients: A Survival Approach
V. Raghul Gandhi1 , R. Nandhinidevi2 , R. Reka3 , K. Saravanaraj4 , Saravanan G.5
Section:Research Paper, Product Type: Journal-Paper
Vol.8 ,
Issue.4 , pp.47-53, Aug-2021
Online published on Aug 31, 2021
Copyright © V. Raghul Gandhi, R. Nandhinidevi, R. Reka, K. Saravanaraj, Saravanan G. . This is an open access article distributed under the Creative Commons Attribution License, which permits unrestricted use, distribution, and reproduction in any medium, provided the original work is properly cited.
View this paper at Google Scholar | DPI Digital Library
How to Cite this Paper
- IEEE Citation
- MLA Citation
- APA Citation
- BibTex Citation
- RIS Citation
IEEE Style Citation: V. Raghul Gandhi, R. Nandhinidevi, R. Reka, K. Saravanaraj, Saravanan G., “Comparison of Different Parametric Modelling for Child Dengue Patients: A Survival Approach,” International Journal of Scientific Research in Mathematical and Statistical Sciences, Vol.8, Issue.4, pp.47-53, 2021.
MLA Style Citation: V. Raghul Gandhi, R. Nandhinidevi, R. Reka, K. Saravanaraj, Saravanan G. "Comparison of Different Parametric Modelling for Child Dengue Patients: A Survival Approach." International Journal of Scientific Research in Mathematical and Statistical Sciences 8.4 (2021): 47-53.
APA Style Citation: V. Raghul Gandhi, R. Nandhinidevi, R. Reka, K. Saravanaraj, Saravanan G., (2021). Comparison of Different Parametric Modelling for Child Dengue Patients: A Survival Approach. International Journal of Scientific Research in Mathematical and Statistical Sciences, 8(4), 47-53.
BibTex Style Citation:
@article{Gandhi_2021,
author = {V. Raghul Gandhi, R. Nandhinidevi, R. Reka, K. Saravanaraj, Saravanan G.},
title = {Comparison of Different Parametric Modelling for Child Dengue Patients: A Survival Approach},
journal = {International Journal of Scientific Research in Mathematical and Statistical Sciences},
issue_date = {8 2021},
volume = {8},
Issue = {4},
month = {8},
year = {2021},
issn = {2347-2693},
pages = {47-53},
url = {https://www.isroset.org/journal/IJSRMSS/full_paper_view.php?paper_id=2487},
publisher = {IJCSE, Indore, INDIA},
}
RIS Style Citation:
TY - JOUR
UR - https://www.isroset.org/journal/IJSRMSS/full_paper_view.php?paper_id=2487
TI - Comparison of Different Parametric Modelling for Child Dengue Patients: A Survival Approach
T2 - International Journal of Scientific Research in Mathematical and Statistical Sciences
AU - V. Raghul Gandhi, R. Nandhinidevi, R. Reka, K. Saravanaraj, Saravanan G.
PY - 2021
DA - 2021/08/31
PB - IJCSE, Indore, INDIA
SP - 47-53
IS - 4
VL - 8
SN - 2347-2693
ER -
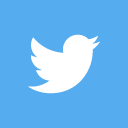
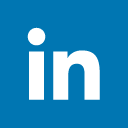
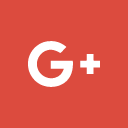
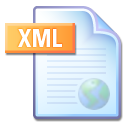
Abstract :
Survival Analysis is one of the important statistical procedures which are mainly used to analyze the time to event data in various fields such as Public Health, Medicine, Political Science, Biostatistics and Epidemiology etc. A Parametric and Non-Parametric method plays a vital role in building a survival model for many diseases. In this paper these parametric models were applied to the data of 109 child dengue patients. The primary goal of this paper is to study the parametric survival models such as Exponential distribution, Weibull distribution, Gompertz distribution and some life time distributions and distribution free Methods such as Kaplan Meier Estimator and Log-Rank test for the dengue data and to compare the best model among Parametric methods by using model measurement criterion as the best model fit is identified by smaller AIC, BIC value and Non Parametric methods is used to examine the survival curves of the Dengue patients. As a result, Logistic distribution is found to be best model. The Statistical Analysis is done by using Statistical software such as IBM SPSS Statistics 21 and R i386 3.6.3 language.
Key-Words / Index Term :
Survival Analysis, Parametric methods, Distribution free methods, AIC and BIC.
References :
[1] L. Handayani, M. Fatekurohman, and D. Anggraeni, “Survival Analysis in Patients with Dengue Hemorrhagic Fever (DHF) Using Cox Proportional Hazard Regression,” 2017.
[2] D. Collett, Modelling survival data in medical research. CRC press, 2015.
[3] E. T. Lee and J. Wang, Statistical methods for survival data analysis, vol. 476. John Wiley & Sons, 2003.
[4] M. A. Pourhoseingholi, E. Hajizadeh, B. Moghimi Dehkordi, A. Safaee, A. Abadi, and M. R. Zali, “Comparing Cox regression and parametric models for survival of patients with gastric carcinoma,” Asian Pacific J. Cancer Prev., vol. 8, no. 3, p. 412, 2007.
[5] V. Vallinayagam, S. Prathap, and P. Venkatesan, “Parametric regression models in the analysis of breast cancer survival data,” Int. J. Sci. Technol., vol. 3, no. 3, pp. 163–167, 2014.
[6] S. E. Amran, M. A. A. Abdullah, S. L. Kek, and S. A. M. Jamil, “Analysis of survival in breast cancer patients by using different parametric models,” in Journal of Physics: Conference Series, 2017, vol. 890, no. 1, p. 12169.
[7] E. A. Hayat, A. Suner, B. Uyar, Ö. Dursun, M. N. Orman, and G. Kitapcioglu, “Comparison of five survival models: Breast cancer registry data from ege university cancer research center,” Turkiye Klin. J Med Sci, vol. 30, no. 5, pp. 1665–1674, 2010.
[8] P. O. Awodutire, O. A. Kolawole, and O. R. Ilori, “Parametric modeling of survival times among breast cancer patients in a teaching hospital, Osogbo,” J. Cancer Treat. Res, vol. 5, no. 5, pp. 81–85, 2017.
[9] S. F. Nisai and I. N. Budiantara, “Analisis Survival dengan Pendekatan Multivariate Adaptive Regression Splines pada Kasus Demam Berdarah Dengue (DBD),” J. sains dan seni its, vol. 1, no. 1, pp. D318–D323, 2012.
[10] K. Srividhya and A. Radhika, “Comparison of Different Parametric Modeling for Time-to-Event Data among Cancer Patients,” vol. 6, no. 1, pp. 187–192, 2019.
[11] N. Sari, “Aplikasi Regresi Cox Propotional Hazard Pada Analisis Kesintasan Dan Identifikasi Faktor Resiko.” Skripsi, 2011.
[12] P. Venkatesan and N. Sundaram, “Exponentiated exponential models for survival data,” Indian J. Sci. Technol., vol. 4, no. 8, pp. 923–930, 2011.
[13] R. Shanker, F. Hagos, and S. Sujatha, “On modeling of Lifetimes data using exponential and Lindley distributions,” Biometrics Biostat. Int. J., vol. 2, no. 5, pp. 1–9, 2015.
[14] N. A. Rather and T. A. Rather, “New Generalizations of Exponential Distribution with Applications,” J. Probab. Stat., vol. 2017, 2017.
[15] W. Weibull, “A statistical distribution function of wide applicability,” J. Appl. Mech., vol. 18, no. 3, pp. 293–297, 1951.
[16] R. Inghelmann et al., “National estimates of cancer patients survival in Italy: a model-based method,” Tumori J., vol. 91, no. 2, pp. 109–115, 2005.
[17] A. R. Baghestani, S. S. Moghaddam, H. A. Majd, M. E. Akbari, N. Nafissi, and K. Gohari, “Survival analysis of patients with breast cancer using weibull parametric model,” Asian Pacific J. Cancer Prev., vol. 16, no. 18, pp. 8567–8571, 2016.
[18] R. D. Horner, “Age at onset of Alzheimer’s disease: clue to the relative importance of etiologic factors?,” Am. J. Epidemiol., vol. 126, no. 3, pp. 409–414, 1987.
[19] P. Tai et al., “Disease-specific survival for limited-stage small-cell lung cancer affected by statistical method of assessment,” BMC Cancer, vol. 7, no. 1, pp. 1–9, 2007.
[20] P. Royston, “The lognormal distribution as a model for survival time in cancer, with an emphasis on prognostic factors,” Stat. Neerl., vol. 55, no. 1, pp. 89–104, 2001.
[21] R. C. Gupta, O. Akman, and S. Lvin, “A study of log?logistic model in survival analysis,” Biometrical J. J. Math. Methods Biosci., vol. 41, no. 4, pp. 431–443, 1999.
[22] Y. Y. Zhou, J. Mi, and S. Guo, “Estimation of parameters in logistic and log-logistic distribution with grouped data,” Lifetime Data Anal., vol. 13, no. 3, pp. 421–429, 2007.
[23] M. M. Siddiqui, “Some problems connected with Rayleigh distributions,” J. Res. Natl. Bur. Stand. D, vol. 66, pp. 167–174, 1962.
[24] H. A. Howlader and A. Hossain, “On Bayesian estimation and prediction from Rayleigh based on type II censored data,” Commun. Stat. Methods, vol. 24, no. 9, pp. 2251–2259, 1995.
[25] S. Dey, “Comparison of Bayes estimators of the parameter and reliability function for Rayleigh distribution under different loss functions,” Malaysian J. Math. Sci., vol. 3, no. 247–264, 2009.
[26] S. Dey and M. K. Das, “A note on prediction interval for a Rayleigh distribution: Bayesian approach,” Am. J. Math. Manag. Sci., vol. 27, no. 1–2, pp. 43–48, 2007.
[27] A. M. Sarhan, D. C. Hamilton, and B. Smith, “Parameter estimation for a two-parameter bathtub-shaped lifetime distribution,” Appl. Math. Model., vol. 36, no. 11, pp. 5380–5392, 2012.
[28] C. Cox, H. Chu, M. F. Schneider, and A. Munoz, “Parametric survival analysis and taxonomy of hazard functions for the generalized gamma distribution,” Stat. Med., vol. 26, no. 23, pp. 4352–4374, 2007.
[29] A. Abadi, F. Amanpour, C. Bajdik, and P. Yavari, “Breast cancer survival analysis: Applying the generalized gamma distribution under different conditions of the proportional hazards and accelerated failure time assumptions,” Int. J. Prev. Med., vol. 3, no. 9, p. 644, 2012.
[30] S. Viscomi, G. Pastore, E. Dama, F. Merletti, and C. Magnani, “Life expectancy as an indicator of outcome,” Epidemiol. Prev., vol. 28, no. 3, pp. 174–177, 2004.
[31] J. W. Vaupel, “How change in age-specific mortality affects life expectancy,” Popul. Stud. (NY)., vol. 40, no. 1, pp. 147–157, 1986.
[32] A. El-Gohary, A. Alshamrani, and A. N. Al-Otaibi, “The generalized Gompertz distribution,” Appl. Math. Model., vol. 37, no. 1–2, pp. 13–24, 2013.
You do not have rights to view the full text article.
Please contact administration for subscription to Journal or individual article.
Mail us at support@isroset.org or view contact page for more details.