Full Paper View Go Back
Amr R. Kamel1 , Abdulaziz A. Alqarni2 , Moataz A. Ahmed3
Section:Research Paper, Product Type: Journal-Paper
Vol.9 ,
Issue.4 , pp.5-22, Aug-2022
Online published on Aug 31, 2022
Copyright © Amr R. Kamel, Abdulaziz A. Alqarni, Moataz A. Ahmed . This is an open access article distributed under the Creative Commons Attribution License, which permits unrestricted use, distribution, and reproduction in any medium, provided the original work is properly cited.
View this paper at Google Scholar | DPI Digital Library
How to Cite this Paper
- IEEE Citation
- MLA Citation
- APA Citation
- BibTex Citation
- RIS Citation
IEEE Style Citation: Amr R. Kamel, Abdulaziz A. Alqarni, Moataz A. Ahmed, “On the Performance Robustness of Artificial Neural Network Approaches and Gumbel Extreme Value Distribution for Prediction of Wind Speed,” International Journal of Scientific Research in Mathematical and Statistical Sciences, Vol.9, Issue.4, pp.5-22, 2022.
MLA Style Citation: Amr R. Kamel, Abdulaziz A. Alqarni, Moataz A. Ahmed "On the Performance Robustness of Artificial Neural Network Approaches and Gumbel Extreme Value Distribution for Prediction of Wind Speed." International Journal of Scientific Research in Mathematical and Statistical Sciences 9.4 (2022): 5-22.
APA Style Citation: Amr R. Kamel, Abdulaziz A. Alqarni, Moataz A. Ahmed, (2022). On the Performance Robustness of Artificial Neural Network Approaches and Gumbel Extreme Value Distribution for Prediction of Wind Speed. International Journal of Scientific Research in Mathematical and Statistical Sciences, 9(4), 5-22.
BibTex Style Citation:
@article{Kamel_2022,
author = {Amr R. Kamel, Abdulaziz A. Alqarni, Moataz A. Ahmed},
title = {On the Performance Robustness of Artificial Neural Network Approaches and Gumbel Extreme Value Distribution for Prediction of Wind Speed},
journal = {International Journal of Scientific Research in Mathematical and Statistical Sciences},
issue_date = {8 2022},
volume = {9},
Issue = {4},
month = {8},
year = {2022},
issn = {2347-2693},
pages = {5-22},
url = {https://www.isroset.org/journal/IJSRMSS/full_paper_view.php?paper_id=2899},
publisher = {IJCSE, Indore, INDIA},
}
RIS Style Citation:
TY - JOUR
UR - https://www.isroset.org/journal/IJSRMSS/full_paper_view.php?paper_id=2899
TI - On the Performance Robustness of Artificial Neural Network Approaches and Gumbel Extreme Value Distribution for Prediction of Wind Speed
T2 - International Journal of Scientific Research in Mathematical and Statistical Sciences
AU - Amr R. Kamel, Abdulaziz A. Alqarni, Moataz A. Ahmed
PY - 2022
DA - 2022/08/31
PB - IJCSE, Indore, INDIA
SP - 5-22
IS - 4
VL - 9
SN - 2347-2693
ER -
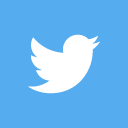
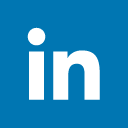
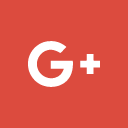
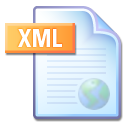
Abstract :
Engineers and scientists have historically focused on modeling and predicting extreme values of geophysical variables, such as wind, ocean surface waves, sea level, temperature, and river flow. In particular, the analysis of wind speed is crucial for different applications in maritime, environmental, civil engineering as well as for the preparation of mitigation and management of natural disasters. This topic is gaining interest in the field of renewable generation, from the viewpoint of assessing both wind power production and wind-tower mechanical reliability and safety. In order to generate trustworthy information for decision support, it is necessary to analyze data spanning extended time periods and recorded at diverse places. Such data must also be analyzed using a variety of techniques. In this paper, we study the Type I Gumbel extreme value (GEV) distribution using graphical and analytical methods for estimation its parameters and determine the best estimators of the unknown parameters of the GEV distribution which can be used to prediction the extreme wind speed. Also, Bayesian estimators of the parameters are derived by using Markov Chain Monte Carlo (MCMC) methods. Moreover, we proposed Bayesian GEV distribution approach to compare the Artificial Neural Networks (ANNs) based on Multi-Layer Perceptrons (MLPs) network for prediction of wind speed. The performance Robustness of the Bayesian GEV distribution approach and ANNs used in wind speed predication are evaluated by some model performance indicators viz, mean absolute error, mean relative error, root mean square error and correlation coefficient. The results showed that the proposed Bayesian GEV distribution approach is found to be better suited for prediction of wind speed.
Key-Words / Index Term :
Artificial neural networks; Bayesian estimation; Extreme value theory; Gumbel extreme value distribution; MCMC; Robustness; Evaluation metrics; Wind speed prediction.
References :
[1] Manwell, J. F., McGowan, J. G., and Rogers, A. L. Wind energy explained: theory, design and application. John Wiley & Sons. Chichester, UK, ?2010.
[2] Sivanandam, S. N., and Deepa, S. N. Introduction to neural networks using Matlab 6.0. Tata McGraw-Hill Education, 2006.
[3] Alharbi, A. A., Kamel, A.R., and Atia, S. A. A New Robust Molding of Heat and Mass Transfer Process in MHD Based on Adaptive-Network-Based Fuzzy Inference System. WSEAS Transactions on Heat and Mass Transfer, Vol.17, pp 80-96, 2022.
[4] Kotz, S., and Nadarajah, S. Extreme value distributions: theory and applications. world scientific, 2000.
[5] Lee, B. H., Ahn, D. J., Kim, H. G., and Ha, Y. C. (2012). An estimation of the extreme wind speed using the Korea wind map. Renewable energy, Vol.42, pp 4-10, 2012.
[6] Mahdi, S., and Cenac, M. Estimating Parameters of Gumbel Distribution using the Methods of Moments, probability weighted Moments and maximum likelihood. Revista de Matemática: Teoríay Aplicaciones, Vol.12, pp 151-156, 2005.
[7] Aydin, D., and ?eno?lu, B. Monte Carlo comparison of the parameter estimation methods for the two-parameter Gumbel distribution. Journal of Modern Applied Statistical Methods, Vol.14, 2015.
[8] Dey, S., Mazucheli, J., and Nadarajah, S. Kumaraswamy distribution: different methods of estimation. Computational and Applied Mathematics, Vol.37, pp 2094-2111, 2018.
[9] Alharbi, Y. S., and Kamel, A.R. Fuzzy System Reliability Analysis for Kumaraswamy Distribution: Bayesian and Non-Bayesian Estimation with Simulation and an Application on Cancer Data Set.? WSEAS Transactions on Biology and Biomedicine, Vol.19, pp118-139, ?2022.
[10] Demirhan, H., and Kalaylioglu, Z. On the generalized multivariate Gumbel distribution. Statistics & Probability Letters, Vol.103, pp 93-99.? 2015.
[11] Cordeiro, G. M., Nadarajah, S., and Ortega, E. M. The kumaraswamy gumbel distribution. Statistical Methods & Applications, Vol.21, pp 139-168, 2012.
[12] Cooray, K. Generalized gumbel distribution. Journal of Applied Statistics, Vol.37, pp 171-179, 2010.
[13] Azad, H. B., Mekhilef, S., and Ganapathy, V. G. Long-term wind speed forecasting and general pattern recognition using neural networks. IEEE Transactions on Sustainable Energy, Vol.5, pp 546-553, 2014.
[14] El-Shanshoury, G. I., and Ramadan, A. A. Estimation of extreme value analysis of wind speed in the north-western coast of Egypt. Arab J Nucl Sci Appl, Vol.45, pp 265-274, ?2012.
[15] Barhmi, S., Elfatni, O., and Belhaj, I. Forecasting of wind speed using multiple linear regression and artificial neural networks. Energy Systems, Vol.11, pp 935-946, 2020.
[16] Chiodo, E., Fantauzzi, M., and Mazzanti, G. The Compound Inverse Rayleigh as an Extreme Wind Speed Distribution and Its Bayes Estimation. Energies, Vol.15, 2022.
[17] Fu, J. Y., Li, Q. S., and Xie, Z. N. Prediction of wind loads on a large flat roof using fuzzy neural networks. Engineering Structures, Vol.28, pp 153-161, 2006.
[18] McCulloch, W. S., and Pitts, W. A logical calculus of the ideas immanent in nervous activity. The bulletin of mathematical biophysics, Vol.5, pp 115-133, 1943.
[19] Metropolis, N., Rosenbluth, A. W., Rosenbluth, M. N., Teller, A. H., and Teller, E. Equation of state calculations by fast computing machines. The journal of chemical physics, Vol.21, pp 1087-1092, 1953.
[20] Rumelhart, D. E. Hinton and R. Williams. Learning Internal Representations by Error Propagation, pp 318-362., 1986.
[21] Lek, S., and Park, Y, S. Artificial Neural Networks. In: Jorgensen, S.E., Fath, B. (Eds.), Encyclopedia of Ecology. Elsevier, Amsterdam, pp. 237-245. 2008.
[22] Perez-Garcia, A. N., Tornez-Xavier, G. M., Flores-Nava, L. M., Gómez-Castañeda, F., and Moreno-Cadenas, J. A. Multilayer perceptron network with integrated training algorithm in FPGA. In 2014 11-th International Conference on Electrical Engineering, Computing Science and Automatic Control (CCE) (pp. 1-6). IEEE.? 2014.
[23] Deshpande, R. R. On the rainfall time series prediction using multilayer perceptron artificial neural network. International Journal of emerging technology and advanced engineering, Vol.2, pp 2250-2459, 2012.
[24] Velo, R., López, P., and Maseda, F. Wind speed estimation using multilayer perceptron. Energy Conversion and Management, Vol.81, pp 1-9, 2014.
[25] van Cranenburgh, S., and Kouwenhoven, M. An artificial neural network based method to uncover the value-of-travel-time distribution. Transportation, Vol.48, pp 2545-2583.? 2021.
[26] Fosgerau, M. Using nonparametrics to specify a model to measure the value of travel time. Transportation Research Part A: Policy and Practice, Vol.41, pp 842-856, 2007.
[27] Coles, S., Bawa, J., Trenner, L., and Dorazio, P. An introduction to statistical modeling of extreme values (Vol. 208, p. 208). London: Springer.? 2001
[28] Kamel, A.R. Handling outliers in seemingly unrelated regression equations model, MSc thesis, Faculty of graduate studies for statistical research (FGSSR), Cairo University, Egypt, 2021.
[29] Gumbel, E.J. Statistical Theory of Extreme Values and Some Practical Applications, a Series of Lectures, Vol. 33, pp.1–49, National Bureau of Standards, Applied Mathematics Series, US Government Printing Office, 1954.
[30] Hansen, A. The three extreme value distributions: An introductory review. Frontiers in Physics, Vol. 8, pp 1-8,? 2020.
[31] Bali, T. G. The generalized extreme value distribution. Economics letters, Vol. 79, pp 423-427, 2003.
[32] Ramachandran, G. Properties of extreme order statistics and their application to fire protection and insurance problems. Fire Safety Journal, Vol. 5, pp 59-76, 1982.
[33] Brown, B. G., and Katz, R. W. Regional analysis of temperature extremes: Spatial analog for climate change?. Journal of Climate, Vol. 8, pp 108-119, 1995.
[34] Naess, A. Estimation of long return period design values for wind speeds. Journal of engineering mechanics, Vol. 124, pp 252-259, 1998.
[35] Broussard, J. P., and Booth, G. G. The behavior of extreme values in Germany`s stock index futures: An application to intradaily margin setting. European Journal of Operational Research, Vol. 104, pp 393-402, 1998.
[36] Xapsos, M. A., Summers, G. P., and Burke, E. A. Extreme value analysis of solar energetic proton peak fluxes. Solar Physics, Vol.183, pp 157-164, seller’s, 1998.
[37] Harlow, D. G., Smith, R. L., and Taylor, H. M. Lower tail analysis of the distribution of the strength of load-sharing systems. Journal of Applied Probability, Vol. 20, pp 358-367,? 1983.
[38] Kuru, G. A., Whyte, A. G. D., and Woollons, R. C. Utility of reverse Weibull and extreme value density functions to refine diameter distribution growth estimates. Forest ecology and management, Vol. 48(1-2), pp 165-174,? 1992.
[39] Jeribi, A., Ghorbel, A. Forecasting developed and BRICS stock markets with cryptocurrencies and gold: generalized orthogonal generalized autoregressive conditional heteroskedasticity and generalized autoregressive score analysis. International Journal of Emerging Markets, in press. 2021. https://doi.org/10.1108/ijoem-06-2020-0688.
[40] Abonazel, M. R., and Abd-Elftah, A. I. Forecasting Egyptian GDP using ARIMA models. Reports on Economics and Finance, Vol.5, pp 35-47, 2019.
[41] Swain, J. J., Venkatraman, S., and Wilson, J. R. Least-squares estimation of distribution functions in Johnson`s translation system. Journal of Statistical Computation and Simulation, Vol. 29, pp 271-297, 1988.
[42] Kao, J. H. Computer methods for estimating Weibull parameters in reliability studies. IRE Transactions on Reliability and Quality Control, pp 15-22,? 1958
[43] Youssef, A. H., Kamel, A. R., and Abonazel, M. R. Robust SURE estimates of profitability in the Egyptian insurance market. Statistical Journal of the IAOS, Vol. 37, pp 1275-1287,? 2021.
[44] Youssef, A. H., Kamel, A. R., and Abonazel, M. R. Efficiency Comparisons of Robust and Non-Robust Estimators for Seemingly Unrelated Regressions Model. WSEAS Transactions on Mathematics, Vol. 21, pp 218-244, 2022.
[45] Almongy, H. M., and Almetwally, E. M. Robust estimation methods of generalized exponential distribution with outliers. Pakistan Journal of Statistics and Operation Research, pp 545-559, 2020.
[46] Gauss, C. F. Least Squares method for the Combinations of Observations. Translated by J. Bertrand.? Mallet-Bachelier, Paris, 1955.
[47] Kumar, K. Classical and Bayesian estimation in log-logistic distribution under random censoring. International Journal of System Assurance Engineering and Management, Vol. 9, pp 440-451, 2018.
[48] Kamel, A.R. Markov Chain Monte Carlo Techniques: A Review and Comparison. Working paper, Faculty of Graduate Studies for Statistical Research, Cairo University, Egypt, 2022.
[49] Hastings, W. K. Monte Carlo sampling methods using Markov chains and their applications.? Biometrika, Vol.57, pp. 97–109. 1970.
[50] Gómez, Y. M., Bolfarine, H., and Gómez, H. W. Gumbel distribution with heavy tails and applications to environmental data. Mathematics and Computers in Simulation, Vol. 157, pp. 115-129, 2019.
[51] Al-Imam, A. A Gateway Towards Machine Learning: Predictive Analytics and Neural Networks in IBM-SPSS (SPSS v. 24). Retrieved January, Vol. 3, 2019.?
[52] Kamel, A. R., and Alqarni, M.A. A New Characterization of Exponential Distribution through Minimum Chi-Squared Divergence Principle. Journal of Advanced Research in Applied Mathematics and Statistics, Vol. 5, pp. 14-26, 2020.
[53] Alizadeh, M., Mojarad, M., and Arfaeinia, H. Provide a Dynamic Routing Algorithm for MPLS Networks Using Fuzzy Filtering Approach. International Journal of Scientific Research in Network Security and Communication, Vol.9, Issue.1, pp.1-7, 2021.
[54] Abonazel, M., and Rabie, A. The impact of using robust estimations in regression models: An application on the Egyptian economy. Journal of Advanced Research in Applied Mathematics and Statistics, Vol. 4, pp. 8-16.? 2019.
[55] Abonazel, M., and Nourhan, E. Using the ARDL bound testing approach to study the inflation rate in Egypt. Economic consultant, Vol. 3, pp. 24-41.? 2020.
[56] Mohamed S.A., and Mohamed S.M. Estimation Methods of Kumaraswamy Lindley Regression Model with Applications on Egyptian Stock Exchange Data. International Journal of Scientific Research in Mathematical and Statistical Sciences, Vol.9, Issue.1, pp.19-23, 2022.
You do not have rights to view the full text article.
Please contact administration for subscription to Journal or individual article.
Mail us at support@isroset.org or view contact page for more details.