Full Paper View Go Back
Solomon Eshun1 , Alberta Araba Johnson2 , Richmond Essieku3
- School of Mathematical and Statistical Sciences, The University of Texas Rio Grande Valley, Edinburg, TX, United States.
- School of Mathematical and Statistical Sciences, The University of Texas Rio Grande Valley, Edinburg, TX, United States.
- Department of Economics, Texas Tech University, Lubbock, TX, United States.
Section:Research Paper, Product Type: Journal-Paper
Vol.10 ,
Issue.3 , pp.1-8, Jun-2023
Online published on Jun 30, 2023
Copyright © Solomon Eshun, Alberta Araba Johnson, Richmond Essieku . This is an open access article distributed under the Creative Commons Attribution License, which permits unrestricted use, distribution, and reproduction in any medium, provided the original work is properly cited.
View this paper at Google Scholar | DPI Digital Library
How to Cite this Paper
- IEEE Citation
- MLA Citation
- APA Citation
- BibTex Citation
- RIS Citation
IEEE Style Citation: Solomon Eshun, Alberta Araba Johnson, Richmond Essieku, “COVID-19 Tweets Sentiment Classification: A Deep Learning Model with Stacking Ensemble for Improved Performance,” International Journal of Scientific Research in Mathematical and Statistical Sciences, Vol.10, Issue.3, pp.1-8, 2023.
MLA Style Citation: Solomon Eshun, Alberta Araba Johnson, Richmond Essieku "COVID-19 Tweets Sentiment Classification: A Deep Learning Model with Stacking Ensemble for Improved Performance." International Journal of Scientific Research in Mathematical and Statistical Sciences 10.3 (2023): 1-8.
APA Style Citation: Solomon Eshun, Alberta Araba Johnson, Richmond Essieku, (2023). COVID-19 Tweets Sentiment Classification: A Deep Learning Model with Stacking Ensemble for Improved Performance. International Journal of Scientific Research in Mathematical and Statistical Sciences, 10(3), 1-8.
BibTex Style Citation:
@article{Eshun_2023,
author = {Solomon Eshun, Alberta Araba Johnson, Richmond Essieku},
title = {COVID-19 Tweets Sentiment Classification: A Deep Learning Model with Stacking Ensemble for Improved Performance},
journal = {International Journal of Scientific Research in Mathematical and Statistical Sciences},
issue_date = {6 2023},
volume = {10},
Issue = {3},
month = {6},
year = {2023},
issn = {2347-2693},
pages = {1-8},
url = {https://www.isroset.org/journal/IJSRMSS/full_paper_view.php?paper_id=3165},
publisher = {IJCSE, Indore, INDIA},
}
RIS Style Citation:
TY - JOUR
UR - https://www.isroset.org/journal/IJSRMSS/full_paper_view.php?paper_id=3165
TI - COVID-19 Tweets Sentiment Classification: A Deep Learning Model with Stacking Ensemble for Improved Performance
T2 - International Journal of Scientific Research in Mathematical and Statistical Sciences
AU - Solomon Eshun, Alberta Araba Johnson, Richmond Essieku
PY - 2023
DA - 2023/06/30
PB - IJCSE, Indore, INDIA
SP - 1-8
IS - 3
VL - 10
SN - 2347-2693
ER -
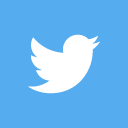
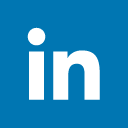
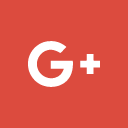
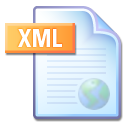
Abstract :
The global impact of COVID-19 has resulted in profound consequences on society, the economy, and public health. In response to this, individuals have turned to social media platforms such as Twitter as a means to voice their sentiments and viewpoints regarding the pandemic. Sentiment analysis can be used to identify public concerns and challenges, such as misinformation and negative attitudes towards vaccinations and public health measures. However, manually analyzing such vast amounts of data is laborious and expensive. Deep learning models have demonstrated encouraging outcomes for sentiment analysis, due to their ability to learn complex patterns and relationships within datasets. As a result, this study aims to use deep learning models to predict sentiment expressed in a tweet on COVID-19 and compare their performances. The objective of the study is to gain insights into how the pandemic is affecting the population and to help policymakers and public health officials develop effective communication strategies and targeted interventions. By comparing the performances of these models, we hope to identify the most effective approach for classifying these sentiments, contributing to the broader conversation about the impacts of the pandemic on public sentiment and mental health.
Key-Words / Index Term :
COVID-19, social media, twitter, sentiment analysis, deep learning
References :
[1]. A. Mislove, S. Lehmann, Y. Y. Ahn, J. P. Onnela and J. N. Rosenquist, “Understanding the Demographics of Twitter Users,” In Proceedings of the Fifth International AAAI Conference on Weblogs and Social Media, pp.554-557, 2010.
[2]. A. Java, X. Song, T. Finin and B. Tseng, “Why we twitter: Understanding Microblogging Usage and Communities,” In Proceedings of the 9th WebKDD and 1st SNA-KDD 2007 Workshop on Web Mining and Social Network Analysis, pp.56-65, 2007.
[3]. P. Bo and L. Lee, “Opinion Mining and Sentiment Analysis,” Foundations and Trends in Information Retrieval, vol.2, no.1-2, pp.1-135, 2008.
[4]. B. Liu, “Sentiment Analysis and Opinion Mining,” Synthesis Lectures on Human Language Technologies, vol.5, no.1, pp.1-167, 2012.
[5]. Y. Zhang and B. Wallace, “A sensitivity Analysis of (and Practitioners’ Guide to) Convolutional Neural Networks for Sentence Classification,” arXiv preprint arXiv:1510.03820, 2015.
[6]. Z. Jalil, A. Abbasi, A.R. Javed, K.B. Muhammad, H. Abul, H. Mozaherul, K.M. Malik and A.K.J. Saudagar, “Covid-19 Related Sentiment Analysis using State-of-the-Art Machine Learning and Deep Learning Techniques,” Frontiers in Public Health, vol.9, 2022.
[7]. F. Barbieri, L.E. Anke and J. Camacho-Collados, “Xlm-t: Multilingual Language Models in Twitter for Sentiment Analysis and Beyond,” In Proceedings of the Thirteenth Language Resources and Evaluation Conference, pp.258-266, 2022.
[8]. L. Rokach, “Ensemble-Based Classifiers,” Artificial Intelligence Review, vol.33, no.1-2, pp.1-39, 2010.
[9]. K. Cho, B. Van Merriënboer, D. Bahdanau and Y. Bengio, “On the Properties of Neural Machine Translation: Encoder–Decoder Approaches,” arXiv preprint arXiv:1409.1259, 2014.
[10]. U. Naseem, I. Razzak, M. Khushi, P. W. Eklund and J. Kim, “COVIDSenti: A Large-Scale Benchmark Twitter Data Set for COVID-19 Sentiment Analysis,” IEEE Transactions on Computational Social Systems, vol.8, no.4, pp.1003-1015, 2021.
[11]. A. Bandi and A. Fellah, “Socio-Analyzer: A Sentiment Analysis using Social Media Data,” In Proceedings of the 28th International Conference, vol.64, pp.61-67, 2017.
[12]. S. Suriy and K. Sindhu Meena, “Sentimental Analysis of Twitter Using Long Short-Term Memory and Gate Recurrent Unit,” International Journal of Scientific Research in Computer Science and Engineering, Vol.8, Issue.1, pp.1-6, 2020.
[13]. S.P. Akinrinwa, O. Olabode, O.C. Agbonifo and K.G. Akintola, “Breast Histopathology Images Multi-Classification using Ensemble of Deep Convolutional Neural Networks,” International Journal of Scientific Research in Computer Science and Engineering, Vol.10, Issue.6, pp.9-21, 2022.
You do not have rights to view the full text article.
Please contact administration for subscription to Journal or individual article.
Mail us at support@isroset.org or view contact page for more details.