Full Paper View Go Back
Robust Depth based weighted Estimator with Application in Discriminant Analysis
R. Muthukrishnan1 , G. Poonkuzhali2
- Department of Statistics, Bharathiar University, Coimbatore, India.
- Department of Statistics, Bharathiar University, Coimbatore, India.
Section:Research Paper, Product Type: Isroset-Journal
Vol.5 ,
Issue.3 , pp.98-101, Jun-2018
CrossRef-DOI: https://doi.org/10.26438/ijsrmss/v5i3.98101
Online published on Jun 30, 2018
Copyright © R. Muthukrishnan, G. Poonkuzhali . This is an open access article distributed under the Creative Commons Attribution License, which permits unrestricted use, distribution, and reproduction in any medium, provided the original work is properly cited.
View this paper at Google Scholar | DPI Digital Library
How to Cite this Paper
- IEEE Citation
- MLA Citation
- APA Citation
- BibTex Citation
- RIS Citation
IEEE Style Citation: R. Muthukrishnan, G. Poonkuzhali, “Robust Depth based weighted Estimator with Application in Discriminant Analysis,” International Journal of Scientific Research in Mathematical and Statistical Sciences, Vol.5, Issue.3, pp.98-101, 2018.
MLA Style Citation: R. Muthukrishnan, G. Poonkuzhali "Robust Depth based weighted Estimator with Application in Discriminant Analysis." International Journal of Scientific Research in Mathematical and Statistical Sciences 5.3 (2018): 98-101.
APA Style Citation: R. Muthukrishnan, G. Poonkuzhali, (2018). Robust Depth based weighted Estimator with Application in Discriminant Analysis. International Journal of Scientific Research in Mathematical and Statistical Sciences, 5(3), 98-101.
BibTex Style Citation:
@article{Muthukrishnan_2018,
author = {R. Muthukrishnan, G. Poonkuzhali},
title = {Robust Depth based weighted Estimator with Application in Discriminant Analysis},
journal = {International Journal of Scientific Research in Mathematical and Statistical Sciences},
issue_date = {6 2018},
volume = {5},
Issue = {3},
month = {6},
year = {2018},
issn = {2347-2693},
pages = {98-101},
url = {https://www.isroset.org/journal/IJSRMSS/full_paper_view.php?paper_id=680},
doi = {https://doi.org/10.26438/ijcse/v5i3.98101}
publisher = {IJCSE, Indore, INDIA},
}
RIS Style Citation:
TY - JOUR
DO = {https://doi.org/10.26438/ijcse/v5i3.98101}
UR - https://www.isroset.org/journal/IJSRMSS/full_paper_view.php?paper_id=680
TI - Robust Depth based weighted Estimator with Application in Discriminant Analysis
T2 - International Journal of Scientific Research in Mathematical and Statistical Sciences
AU - R. Muthukrishnan, G. Poonkuzhali
PY - 2018
DA - 2018/06/30
PB - IJCSE, Indore, INDIA
SP - 98-101
IS - 3
VL - 5
SN - 2347-2693
ER -
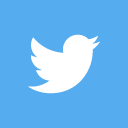
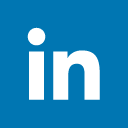
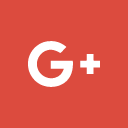
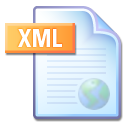
Abstract :
Data depth concept used to measure the deepness of a given point in the entire multivariate data cloud. It leads to center-outward ordering of sample points used rather than usual smallest to largest rank. The ordering starts from middle and moves in all directions. Multivariate location and scatter can be computed by using the depth value of each data point. Various depth procedures have been established by many authors. In this paper, a new depth procedure is proposed, namely Modified Mahalanobis Depth (MMD), which calculates depth based on robust distance with Minimum Covariance Determinant (MCD) approach and a weight function is established to determine the location and scale. The superiority of the proposed depth based procedure over existing depth procedures has been studied in simulated environment using R software with respect to application in discriminant analysis. The proposed depth procedure performs well when compared with the existing procedures even with higher contamination levels and larger sample sizes.
Key-Words / Index Term :
Data Depth, Mahalanobis Distance, MCD, Robust Distance, Weight Function, Discriminat Analysis, R Software
References :
[1]. T.W. Tukey, “Mathematics and picturing data,” In Proceedings of the International Congress on Mathematics (R. D. James, ed.), Canadian Math. Congress, 1975, Vol. 2, pp. 523–531
[2]. H. Oja, “Descriptive statistics for multivariate distributions,” Statistics and Probability Letters, Vol. 1, pp. 327–332, 1983.
[3]. R.Y. Liu, “On a notion of data depth based on random simplices,” Annals of Statistics Vol. 18, pp. 405–414, 1990.
[4]. P.J. Rousseeuw, I. Ruts, “Bivariate location depth,” Journal of the Royal Statistical Society, Vol. 45, Series C, pp. 516-526, 1996.
[5]. G. Koshevoy, K. Mosler,” Zonoid trimming for multivariate distributions,” Annals of Statistics, Vol. 25, pp. 1998-2017, 1997.
[6]. Y. Zuo, R. Serfling, “General notions of statistical depth function,” The Annals of Statistics, Vol. 28, pp. 461–482, 2000.
[7]. Y. Vardi, C.H. Zhang,. “The multivariate L1-median and associated data depth,” Proceedings of the National Academy of Sciences, U.S.A., 2000,Vol. 97, pp. 1423-1426.
[8]. K. Mosler, Multivariate Dispersion, Central Regions and Depth : The Lift Zonoid Approach. Springer, New York, 2002.
[9]. A. Cuevas, M. Febrero, R. Fraiman, “Robust estimation and classification for functional data via projection-based depth notions,” Computational Statistics, Vol. 22, pp. 481-496, 2007.
[10]. J. Cuesta-Albertos, A. Nieto-Reyes, “The random Tukey depth,” Computational Statistics and Data Analysis, Vol. 52, pp. 4979-4988, 2008.
[11]. Y. Hu, Q. Li, Y. Wang, Y. Wu, “Rayleigh Projection depth,” Computational Statistics, Vol. 27, pp. 523-530, 2012.
[12]. D. Paindaveine, G. Van Bever, “From depth to local depth: a focus on centrality,” Journal of the American Statistical Association, Vol. 105, pp. 1105-1119, 2013.
[13]. D. Chen, P. Morin, “Approximating majority depth,” Computational geometry: theory and applications, Vol. 46, pp. 1059-1064, 2013.
[14]. R. Dyckerhoff, C. Ley, D. Paindaveine, “Depth-based runs tests for bivariate central symmetry,” Annals of the Institute of Statistical Mathematics, Vol. 67, Issue 5, pp. 917-941, 2015.
[15]. M. Hubert, P.J. Rousseeuw, P. Segaert, “Multivariate and functional classification using depth and distance,” Advances in Data Analysis and Classification.Vol. 11, Issue 3, pp. 445-466, 2017.
[16]. R. Muthukrishnan, G. Poonkuzhali, “Computing Robust Measure of Multivariate location – data depth approach,” Global Journal of Pure and Applied Mathematics, Vol. 13, Issue 2, pp. 691-700, 2017.
[17]. I. Cascos, Data depth: Multivariate statistics and geometry (W. Kendall, I. Molchanov, eds.) New Perspectives in Stochastic Geometry, Clarendon Press, Oxford University Press, Oxford, 2009.
[18]. K. Mosler, Depth statistics (C. Becker, R. Fried, S. Kuhnt, eds.) Robustness and Complex Data Structures, Festschrift in Honour of Ursula Gather, Springer, Berlin pp. 17–34, 2013.
[19]. R. Muthukrishnan, G. Poonkuzhali, “Computing median with data depth in multivariate data,” Journal of modern sciences, Vol. 7, Issue 2, pp. 11-19, 2015.
[20]. P.C. Mahalanobis, “On the generalized distance in statistics”, Proceedings of National Institute of Sciences. India, 1936,Vol. 12, pp. 49-55,
[21]. P. Chaudhuri, “On a geometric notion of quantiles for multivariate data,” Journal of the American Statistical Association, Vol. 91, pp. 862-872, 1996.
[22]. R. Serfling, “Depth functions in nonparametric multivariate inference. In R. Liu, R. Serfling and D. Souvaine, eds., Data Depth: Robust Multivariate Analysis,” Computational Geometry and Applications, American Mathematical Society, 1–16, 2006.
[23]. P.J. Rousseeuw, “Least median of squares regression,” Journal of the American Statistical Association, Vol. 79, pp. 871-880, 1984.
You do not have rights to view the full text article.
Please contact administration for subscription to Journal or individual article.
Mail us at support@isroset.org or view contact page for more details.