Full Paper View Go Back
Volatility of daily and Weekly Crude Oil Prices – A Statistical Perspective
Kanthala Sampath Kumar1 , V. Swapna2 , S.A. JyothiRani3 , V.V. Hara Gopal4
Section:Research Paper, Product Type: Isroset-Journal
Vol.5 ,
Issue.5 , pp.108-113, Oct-2018
CrossRef-DOI: https://doi.org/10.26438/ijsrmss/v5i5.108113
Online published on Oct 31, 2018
Copyright © Kanthala Sampath Kumar, V. Swapna, S.A. JyothiRani , V.V. Hara Gopal . This is an open access article distributed under the Creative Commons Attribution License, which permits unrestricted use, distribution, and reproduction in any medium, provided the original work is properly cited.
View this paper at Google Scholar | DPI Digital Library
How to Cite this Paper
- IEEE Citation
- MLA Citation
- APA Citation
- BibTex Citation
- RIS Citation
IEEE Style Citation: Kanthala Sampath Kumar, V. Swapna, S.A. JyothiRani , V.V. Hara Gopal, “Volatility of daily and Weekly Crude Oil Prices – A Statistical Perspective,” International Journal of Scientific Research in Mathematical and Statistical Sciences, Vol.5, Issue.5, pp.108-113, 2018.
MLA Style Citation: Kanthala Sampath Kumar, V. Swapna, S.A. JyothiRani , V.V. Hara Gopal "Volatility of daily and Weekly Crude Oil Prices – A Statistical Perspective." International Journal of Scientific Research in Mathematical and Statistical Sciences 5.5 (2018): 108-113.
APA Style Citation: Kanthala Sampath Kumar, V. Swapna, S.A. JyothiRani , V.V. Hara Gopal, (2018). Volatility of daily and Weekly Crude Oil Prices – A Statistical Perspective. International Journal of Scientific Research in Mathematical and Statistical Sciences, 5(5), 108-113.
BibTex Style Citation:
@article{Kumar_2018,
author = {Kanthala Sampath Kumar, V. Swapna, S.A. JyothiRani , V.V. Hara Gopal},
title = {Volatility of daily and Weekly Crude Oil Prices – A Statistical Perspective},
journal = {International Journal of Scientific Research in Mathematical and Statistical Sciences},
issue_date = {10 2018},
volume = {5},
Issue = {5},
month = {10},
year = {2018},
issn = {2347-2693},
pages = {108-113},
url = {https://www.isroset.org/journal/IJSRMSS/full_paper_view.php?paper_id=876},
doi = {https://doi.org/10.26438/ijcse/v5i5.108113}
publisher = {IJCSE, Indore, INDIA},
}
RIS Style Citation:
TY - JOUR
DO = {https://doi.org/10.26438/ijcse/v5i5.108113}
UR - https://www.isroset.org/journal/IJSRMSS/full_paper_view.php?paper_id=876
TI - Volatility of daily and Weekly Crude Oil Prices – A Statistical Perspective
T2 - International Journal of Scientific Research in Mathematical and Statistical Sciences
AU - Kanthala Sampath Kumar, V. Swapna, S.A. JyothiRani , V.V. Hara Gopal
PY - 2018
DA - 2018/10/31
PB - IJCSE, Indore, INDIA
SP - 108-113
IS - 5
VL - 5
SN - 2347-2693
ER -
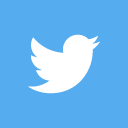
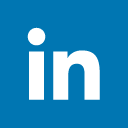
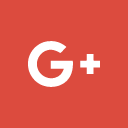
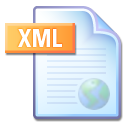
Abstract :
Crude oil is a key commodity for the economy of any country. The changes in Crude oil prices are very complex and therefore, seem to be unpredictable. The effect of increasing price and its daily fluctuations affects not only the economies and financial markets but extends to reach individuals. This is because an increase in oil prices has a direct effect on petrol prices, in addition, it also affects the prices of other goods and services. Therefore, forecasting crude oil is a very important task to reduce the impact of price fluctuations, and help investors, hedgers, and individuals to make decisions when dealing with energy markets. However, one of the main challenges of the econometric models is to forecast such a seemingly unpredictable economic series. The econometric models have not been promising when used for forecasting, particularly in the case of complex series such as oil prices. Although linear and nonlinear time series models have performed much better job in forecasting oil prices, there is yet room for an improvement. If the data generating process is nonlinear, applying linear models could result in large forecast errors. Model specification in nonlinear modeling can also be very case dependent and time-consuming. This article proposes a novel technique for forecasting crude oil price based on Neural Networks. The study adopts the data on crude oil price of West Texas Intermediate (WTI). To evaluate the performance of the model, the study employs three measures, RMSE, MAE and MAPE. In this study, the forecasting accuracy of two models of neural networks, BPN and RNN are compared with the traditional econometric model. The results reveal that the proposed method outperforms the other in terms of forecast accuracy
Key-Words / Index Term :
Neural networks, root mean squared error (RMSE), mean absolute error (MAE), mean absolute percentage error (MAPE),Recurrent Neural Networks(RNN),Multi-Layer Perceptron (MLP),Oil Price and ARIMA.
References :
[1] Bashar Al-hnaity and MaysamAbbod (2016), Predicting Financial Time Series Data Using Hybrid Model, © Springer International Publishing Switzerland.
[2] Box, G.E.O. and G.M. Jenkins (1976), Time Series Analysis, Revised Edition, Holden-Day San Francisco.
[3] Box, G. E. P. and Jenkins, G. M. (1970), Time Series Analysis Forecasting and Control, Pearson Education.
[4] Chatfield, C., 1991. The Analysis of Time Series. Chapman and Hall, New York.
[5] De Gooijer, Jan G., and Rob J. Hyndman. "25 years of time series forecasting." International journal of forecasting 22.3 (2006): 443-473.
[6] FatimahMohd. (1986), Crude Palm Oil Price Forecasting: Box-Jenkins Approach, Pertanika, Vol. 9 No.3.
[7] Haidar, Imad, Siddhivinayak Kulkarni, and Heping Pan. "Forecasting model for crude oil prices based on artificial neural networks." Intelligent Sensors, Sensor Networks and Information Processing, 2008. ISSNIP 2008. International Conference on. IEEE, 2008.
[8] Hamdi, Manel, and ChakerAloui. "Forecasting crude oil price using artificial neural networks: a literature survey." Econ Bull 3.2 (2015): 1339-1359.
[9] Koutroumanidis, Theodoros, Konstantinos Ioannou, and GaryfallosArabatzis. "
Predicting fuelwood prices in Greece with the use of ARIMA models, artificial neural
networks and a hybrid ARIMA–ANN model." Energy Policy 37.9 (2009): 3627-3634.
[10] Maditinos, Dimitrios, and ProdromosChatzoglou. "The use of neural networks in
forecasting." Review of Economic Sciences 6 (2004): 161-176.
[11] Mohamed A. Hmilad(2015), Regression analysis to forecast Malaysia’s imports of crude material, International Journal of Management and Applied Science, ISSN: 23947926, Volume-1, Issue-4.
[12] Oancea, B., &Ciucu, Ş. C. (2014). Time series forecasting using neural networks. arXiv preprint arXiv:1401.1333.
[13] Rana Abdullah Ahmed (2014), Daily crude oil price forecasting model using ARIMA, Generalised autoregressive Conditional Heteroscedastic and support vector Machines, American Journal of Applied Sciences, 11 (3): 425-432
[14] Rani, SA Jyothi, V. V. Haragopal, and M. Krishna Reddy. "Forecasting Inflation Rate
of India using Neural Networks." International Journal of Computer Applications
158.5 (2017).
[15] Rani, SA Jyothi, and V. V. Haragopal. "Forecasting Exchange Rates using Neural
Neworks."
[16] S. Moshiri and F. Foroutan, "Forecasting nonlinear crude oilfutures prices,
" The Energy Journal, vol. 27, pp. 81-95, 2005.
[17] Yadav, S., & Meshram, S. (2013). Brain tumor detection using clustering method. International Journal of Computational Engineering Research, 3(4), 11-14.
You do not have rights to view the full text article.
Please contact administration for subscription to Journal or individual article.
Mail us at support@isroset.org or view contact page for more details.